Abstract
The objective of this paper is to evaluate the effect of different machining parameters on the surface roughness of Hardox 600 (hard and tough steel with high wear resistance and hardness of 600 HBW) in the milling process using the Taguchi optimization method. The selected process parameters are feed rate, spindle speed, the depth of cut, and radial immersion. Based on the number of parameters and their levels L9, an orthogonal array of the Taguchi was chosen. Surface roughness data were collected for nine experiments. Signal to noise (S/N) ratio and analysis of variance calculation were conducted to determine the optimum level and percentage of contribution of each parameter. Furthermore, the mathematical model was created to determine the predicted value of S/N ratio, and the experiments were implemented to justify the mathematical model. It is found that the margin error for the mathematical model and the experimental result was 5.5%, resulting from any uncontrollable parameters affecting the machining process. It is also identified that the radial immersion was the most significant parameter with 45.33% of contribution in the milling procedure of steel with high hardness (Hardox 600). Hence, the surface roughness was mostly influenced by radial immersion (D), followed by the depth of cut (C), spindle speed (B), and finally, feed rate (A) respectively based on the level of their significance. The optimal level of the selected parameters for the minimum surface roughness value is the radial immersion at level 1, depth of cut at level 1, spindle speed at level 2, and feed rate at level 2.
Similar content being viewed by others
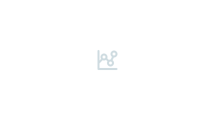
1 Introduction
1.1 Milling operations
In today’s competitive world, there is a significant demand for high-performance materials, such as super-alloys and hardened steels. Milling operations are widely used for the manufacturing of complex sculptured parts using mentioned high-performance materials in different industrial sectors, such as aerospace, biomedical, and automotive. It is obvious that understanding of the surface generation mechanism and estimation of final surface roughness during milling operation is crucial to eliminate trial and error methods in achieving desired surface quality. Concurrently, achieving desired surface quality in the fastest way will reduce manufacturing costs and increase productivity. Therefore, a significant amount of fundamental research has been performed in this field to simulate surface texture and estimate surface roughness during milling operations [1,2,3].
Some investigations have been performed to determine the significant parameters affecting surface roughness. The effects of chatter vibrations during heavy-duty machining operations were analytically investigated by Altintas and Budak [4]. Recently, Munoa et al. [5] excellently performed a critical review over 60 years on chatter suspension techniques in metal cutting operations. Zahoor et al. [6] also analyzed the effect of forced vibrations on surface roughness of AISI P20 during vertical milling and stated that the vibration amplitude and depth of cut are 2 most significant factors affecting surface roughness. Also, the authors mentioned that excessive machine vibrations resulted in cutting tool failures. Bagci and Aykut [7] analyzed the effect of feed rate, cutting speed, and the depth of cut on optimal surface roughness of cobalt-based alloy through the set of milling experiments employing the Taguchi optimization method. Several researchers have similarly employed statistical methods to estimate or minimize surface roughness. Kuram and Ozcelik [8] used Taguchi’s signal to noise ratio to optimize the tool wear, cutting forces, and surface roughness. Also, the relation between dependent and independent variables was modeled using fuzzy and regression modeling. The model proved that the implemented tools with the optimization process are efficient enough to predict the tool wear, cutting forces, and surface roughness. Costa et al. [9] employed Weighted Principal Component Analysis (WPCA) combined with Taguchi’s signal to noise ratio to identify optimal cutting parameters for the dry milling process of AISI 1045 that will result in maximum Material Removal Rate (MRR) and minimum surface roughness. The final result demonstrates that fz = 0.08 mm/tooth, ap = 1.62 mm, Vc = 331 m/min, and ae = 15.49 mm are the optimum level for minimum roughness and maximum material removal. Tlhabadira et al. [10] proposed a combined mathematical model that uses inputs from numerical and experimental results to express surface roughness as a function of machining parameters. The numerical model estimated potential contact pressure, stress, and deformation of a cutting tool while cutting operation, and experimental results, in the form of the Taguchi method were used to understand the influence of the cutting speed, feed, and the depth of cut on final surface roughness. The Technique for Order of Preference by Similarity to Ideal Solution (TOPSIS) is another optimization technique in engineering applications. In electrical discharge machining, the effect of different parameters on the Machinability of Inconel 718 is evaluated using TOPSIS [11]. Also, the surface roughness of stainless steel has been evaluated using statistical methods such as TOPSIS [12]. Manav et al. [13] proposed an improvement of surface roughness for sculptured surfaces based on the toolpath optimization algorithm. The introduced algorithm simultaneously considers multiple objectives such as cutting force, scallop height, and cycle time minimization.
Additional complications occur during machining of high-performance materials, such as super-alloys and hardened steels. Arruda et al. [14] presented a comprehensive optimization model that employs robust parameter design and response surface methodologies to estimate surface roughness of AISI H13 hot-working tool steel during the finishing operation. Optimization model used tool overhang length, workpiece tilt angle, tool flank wear, and cutting strategy as noise factors in achieving control factors’ (feed per tooth, axial depth of cut, radial depth of cut, and cutting speed) levels insensitive to noise variations. Mia et al. [15] used Taguchi-based Grey relational analysis to find the optimum fluid flow rate for MQL (Minimum Quantity Lubrication) that will result in minimum cutting force and surface roughness during end milling of hardened AISI 4140 steel.
The most common application of Hardox is to fabricate different parts of machinery in agriculture, mining, construction, and transportation, resulting from an exceptional combination of material properties in higher levels, namely hardness, toughness, and strength [16]. High resistance to wear friction and weldability are the central capability of Hardox [17]. Hardox 600 is known as one of the categories of Hardox apparatus with high ductility, toughness, and resistance to impact loads and abrasion [18]. The most common application of Hardox 600 is to have high resistance to the abrasion of any parts [18]. It is worth noting that machining the steel with high hardness is not an easy task; hence, setting the machining parameters at their optimum levels is very significant.
1.2 Taguchi application
One of the conventional methods to implement the experiments is the trial and error method, which is very time consuming and expensive. Taguchi method is an acceptable method to be replaced instead of conventional methods, which leads to a reduction in cost and time [19,20,21]. Taguchi method is one of the significant quality strategies to minimize the number of experiments and also consider the effects of all controllable factors [22]. A wide range of studies focused on determining the importance of the parameters and the percentage of their contribution, there are many studies, demonstrate that the Taguchi method is a suitable tool to apply. In the injection moulding industry, to find the optimum levels of significant controllable parameters to reduce the amount of internal and external defects, namely shrinkage, warpage, weld lines, and short shot, the Taguchi method was applied. The result showed that the right set of machine parameters would reduce the scrap rate by up to 25% [23,24,25].
The aforementioned literature survey reveals that the effects of machining parameters on machining any type of steel with high hardness are significant to consider in milling procedure. The review offered here highlights that the number of studies that evaluated the influence of machining parameters on surface roughness of Hardox 600 is limited. To the best of authors, there is not enough study on the surface roughness of steel with high hardness (Hardox 600). Therefore, the main goal of this paper is to investigate and evaluate the effect of selected parameters, namely the feed rate, the spindle speed, the depth of cut, and the radial immersion on the surface roughness of Hardox 600 via the Design of Experiment (DOE). The main application of DOE is to have control over the controllable parameters. To ensure that the clamping force or vibration of the workpiece, which is extraneous parameter, does not show any effect on the surface roughness, certain protocols have been conducted. The protocol details are explained in the experimental stage in detail.
The paper is structured as follow; after a brief introduction in Sect. 1, the experimental methodology is described in Sect. 2. The process of experimental design; a selection of parameters and details of numerical analysis are presented in Sect. 3, which is followed by results and discussion in Sect. 4. Sample of calculations are presented in this section as well. Finally, this work will end up with the conclusions in Sect. 5.
2 Experimental methodology
2.1 Selection of materials and machining setup
Hardox 600 is selected as the workpiece for this study. Hardox 600 is a quenched and tempered wear-resistant steel plate with hardness 570 HBW, yield strength 1650 N/mm2, and tensile strength 2000 N/mm2. The workpiece has a dimension of 20 × 45 × 300 mm. The maximum chemical compositions of Hardox are presented in Table 1.
The selected cutting tool for this research is ZCC (TPKN2204) with cutting speed (Vc) 220 m/min and feed per tooth (fz) 0.2 mm/tooth. The milling machine is the conventional 3-axis machine with a maximum spindle speed of 800 rpm and main power of 7.5 kW drive motor. Four inserts are mounted on the cartridge with a diameter of 100 mm.
2.2 Measuring response parameters
The objective of this paper is to measure the surface roughness (Rz) of Hardox 600 in the milling process. The measurement system for this experiment is Surfcam 200. To guarantee that the accuracy and precision of the measurement procedure are obtained, each experiment was implemented 3 times at different points of the machined surfaces. Hence, the average value of surface roughness for individual experiments is considered for the next step of calculations. The setup for implementing the experiment and measurement process is shown in Fig. 1. Firstly, the machine setup and clamping of Hardox 600 are conducted by following certain protocols. In the second step, 9 experiments with different levels of the selected parameters are implemented based on the Taguchi method. The surface roughness of each part from different experiments, then, is measured for 3 times. Finally, the data analysis on surface roughness of Hardox 600 to find the significant parameters, their optimal levels, and their percentage of contribution, is conducted via Signal to Noise ratio and Analysis of Variance (ANOVA).
3 Process of experimental design
3.1 Selection of parameters
There are several parameters that affect the surface quality in machining procedures. Based on the selected material (Hardox), it is a need to know the right set of significant process parameters in the milling procedure. The selected process parameters are considered and selected based on the literature survey. These chosen parameters are A = feed rate (mm/min), B = the spindle speed (RPM), C = the depth of cut (mm), and D = the radial immersion (%), as shown in Fig. 2 and tabulated in Table 2. It is worth noting that based on the author’s knowledge, the number of studies about the effect of radial immersion rate (D) for machining steel with high hardness (570 HBW) is rare.
3.2 Selection of orthogonal array
Based on the number of selected parameters and their levels, the orthogonal array of Taguchi will be L9. This array is tabulated in Table3. It is seen that 3 levels are chosen for each parameter, which provides 9 trails in total.
3.3 S/N ratio approach
The main concept of Signal to Noise ratio (S/N) is a reduction of the noise based on different definitions of quality characteristics. There are 3 different types of quality characteristics for S/N calculation namely, the smaller the better, the nominal the best, and the larger the better.
According to the main objective of this study, which focuses on the reduction of surface roughness, it is worth noting that the smaller the better quality characteristic will be applied as shown by Eqs. 1, 2 [26]:
The mean squared division (MSD) for the smaller the better quality characteristic can be stated by following equation [26]:
Here, \(N\) is the total number of data points for each experiment and \({y}_{i}\) represents the surface roughness of the different tests. In this paper, the number of data points for each experiment was repeated 3 times. This guarantees that the precision and accuracy of the individual tests can be obtained.
3.4 Analysis of variance (ANOVA)
After determining the most significant parameters affecting the surface roughness and their optimum levels, the next step is to determine the percentage of their contributions using ANOVA. Equations 3–5 employed to evaluate the percentage of contribution of individual parameters [27]. As a result, the sum of squares, the pure sum of a square, and the errors can be found, respectively as:
where \(SS_{{P_{i} }}\) is the sum of the square, \(SS_{T}\) is the total sum of the square, and \(SS_{E}\) is the error value of the sum of square. \(A_{i}\) is the average surface roughness at each level, \(n_{{A_{i} }}\) stands for the number of parameter levels, \(x_{i}\) represents the surface roughness value in each trial, and \(N\) is the number of experiments based on the selected orthogonal array.
The percentage of contribution of each parameter (\(P_{{P_{i} }}\)) is calculated via Eq. 6. Also, the error value for the sum of the square is also calculated by Eq. 7 [27].
4 Results and discussion
In this study, L9 orthogonal array of the Taguchi is selected (Table 3) according to the number of parameters and the number of levels. Consequently, there are 9 experiments that require to be implemented to evaluate the surface roughness of Hardox 600. For each experiment, the level of all selected parameters has been changed to measure the surface roughness value. There are 3 different blocks of Hardox in this study, as shown in Fig. 3a. Fixing the Hardox blocks on the milling machine table is one of the challenging steps to eliminate the influence of extraneous parameters affecting the milling process, such as vibration from clamping. To achieve this, considering different protocols to avoid having vibration from clamping was required. One of the significant protocols is to fill the gap space between the part and the clamp from different sides. For instance, using thin plates to fill the space between the button side of the workpiece and clamp is one of the protocols to follow as shown in Fig. 3b. Another protocol is to ensure that the selected blocks are clamped at the center of the clamp to avoid any vibration; although, it is not possible to remove the vibration completely during the milling process. Also, the height difference between the top face of the clamp and block should be set at a minimum to avoid any vibration. Finally, the top face of the clamp and the selected block need to be aligned in the same plane.
The next step is to implement three experiments on each block by setting different parameters for individual tests based on Table 3. For instance, test 1 was conducted with a feed rate of 16 mm/min (parameter A), spindle speed of 200 rpm (parameter B), depth of cut 0.2 mm (parameter c), and tool interaction 33% (parameter D). Feed rate, cutting speed, and depth of cut had been evaluated for surface roughness [8, 10], but only few studies evaluated the effect of tool interaction for the surface roughness of different steels and based on the literature survey, there is a need to consider the tool interaction for tough steel with high wear resistance and hardness.
Nine different experiments have been implemented with different conditions as shown in Fig. 4 for Hardox 600. The measurement tool of Surfscan 200 was used to measure the average roughness (Ra) and the difference between the maximum peak and the deepest valley (Rz). Accordingly, Ra and Rz were evaluated for 9 experiments. The measuring length of 9 experiments to determine the Ra and Rz value is 50 mm. Nine experiments are conducted and the results are tabulated in Table 4 for a better comparison.
After determining the Rz and Ra value, S/N ratio for test cases is determined based on Eqs. 1, 2 and \({L}_{9}\) orthogonal array. Because the selected quality characteristic is smaller the better, then Rz value is selected and tabulated, as shown in Table 5.
Now, the response table (Table 6) can be evaluated according to the average S/N ratio (Table 5). Also, Figs. 5, 6 are plotted based on Table 6 to present and highlight the optimal levels and the interaction plot of four selected parameters, respectively.
From the S/N responses obtained in Table 6, it can be concluded that the higher S/N ratios of individual parameters of different levels represent the most optimal level of each parameter. Hence, the highest difference between the maximum value and minimum value of the S/N ratio for each parameter represents the most significant factor affecting the experimental result. It is seen that the surface roughness was mostly influenced by radial immersion (D), followed by the depth of cut (C), spindle speed (B), and finally, feed rate (A).
As shown in Fig. 5, by selecting the highest level of S/N ratio for each parameter, it is seen that the optimal level of the selected parameters for the minimum surface roughness value is the radial immersion (D) at level 2, which is followed by the depth of cut (C) at level 2, spindle speed (B) at level 1, and feed rate (A) at level 1. Thus, the result is a combination of A1, B1, C2, and D2.
The value of this study in addition to experimental result, is to develop a mathematical model which represent the effect of different variables on surface roughness of the selected material. Comparing the predicted value using the following code with the experimental result with low margin error reflects the robustness of Taguchi method, experimental text and the mathematical model. A mathematical model was employed to calculate the maximum and minimum value of the S/N ratio, as shown in Eq. 8. The mathematical program was written in MATLAB based on Taguchi orthogonal array L9 and all required equations to predict the maximum value of Signal to Noise Ratio. Therefore,
Maximum S/N
where (A1) Ave is the S/N value of parameter A at level 1, (B1) Ave is the S/N value of parameter B at level 1, (C2) Ave is the S/N value of parameter C at level 2, (D2) Ave is the S/N value of parameter D at level 2.
By applying the optimum level for each parameter, the maximum value of S/N ratio can be evaluated as follow:
The maximum value of the S/N ratio represents the minimum value of surface roughness. Hence, by applying the maximum value of S/N ratio, the optimum level of surface roughness is (based on Eqs. 1, 2):
Minimum surface roughness:
then
By comparing the optimum level of surface roughness with the result in Table 5, it can be seen that the optimum value of surface roughness is the lowest value among all implemented experiments. To justify the mathematical model, the experiment was conducted by applying the optimum level throughout the milling process, as shown in Fig. 6. After the milling process, Rz value is measured (Rz = 0.085 \(\mu m\)), and the result represents the reasonability of the predicted value via the mathematical model of S/N ratio. The margin error was also calculated based on Eq. 9:
By using Eq. 9, the Margin error is 5.5%, which guarantees that the predicted value of the S/N ratio using the mathematical model is acceptable. Having margin error below 10% in engineering fields is reasonable for the predicted value based on a mathematical model and the experimental result [28]. The reason for having a 5.5% margin error is related to any uncontrollable parameters affecting the milling process.
The percentage of contribution (PC) for each parameter is calculated using Analysis of Variance (ANOVA) as follow [27]:
-
1.
Degree of freedom
The total degree of freedom (\({df}_{T}\)), and the degree of freedom of parameter A (\({df}_{A}\)) are calculated based on Eqs. 10, 11:
where \(N\) is the total number of trials, kA represents the number of selected factors and dfE stands for the degree of freedom for error variance based on Eq. 12.
-
2.
Sum of squares
The sum of the square of factor A (SSA)the total sum of square (SST)and the sum of the square for error variance (SSE) are calculated using Eqs.3, 4, 5 respectively, as shown in Table 7.
-
3.
PC
The percentage of contribution for n parameters is calculated using Eq. 6, as tabulated in Table 7.
The surface roughness data in Table 5 were analyzed via ANOVA and the percentage of contribution (PC%) for each selected factor was evaluated based on Eq. 6 as shown in Table 7. The percentage weight of radial immersion has the highest contribution of 45.33%, followed by spindle speed at 21.12%, the depth of cut at 19.97%, and feed rate at 13.56%.
Degree of freedom:
\(df_{SR} = \left( {K_{SR} - 1} \right) = \left( {3 - 1} \right) = 2\), for factor (A)
Sum of squares:
5 Conclusion
The objective of this paper was to analyze the effect of different machining parameters on surface roughness of Hardox 600 in the milling process. Nine different experiments were conducted for the selected parameters, based on the Taguchi optimization method. Signal to Noise (S/N) ratio and the analysis of variance (ANOVA) was applied to determine the optimum level and the percentage of their contributions. The optimum level of individual parameters was radial immersion (D) at level 1, depth of cut (C) at level 1, spindle speed (B) at level 2, and feed rate (A) at level 2. Percentage of contribution for the selected parameters are as follow: radial immersion with 45.33%, spindle speed with 21.12%, depth of cut with 19.97%, and feed rate with 13.56%. The mathematical model was developed to predict the S/N value using MATLAB. The experiment was conducted by applying the optimum level from the experimental result to justify the mathematical model. Rz value was measured (Rz = 0.085 \(\mu m\)), and the result represented the reasonability of the predicted value via the mathematical model of the S/N ratio with a margin error of 5.5%. Hence, radial immersion was the most significant parameter with the highest contribution percentage followed by spindle speed and depth of cut. The number of selected parameters for evaluating the surface roughness of the selected material was the main limitation for this study. Considering more process parameters for machining of steel with high wear resistance and hardness is one of the potentials for future research.
References
Montgomery D, Altintas Y (1991) Mechanism of cutting force and surface generation in dynamic milling. J Eng Ind 113(2):160–168
Ehmann KF, Hong MS (1994) A Generalized model of the surface generation process in metal cutting. CIRP Ann Manuf Technol 43:483–486
Layegh KSE, Lazoglu I (2017) 3D surface topography analysis in 5-axis ball-end milling. CIRP Ann Manuf Technol 66:133–136
Altintas Y, Budak E (1995) Analytical prediction of stability lobes in milling. CIRP Ann Manuf Technol 44:357–362
Munoa J, Beudaert X, Dombovari Z, Altintas Y, Budak E, Brecher C, Stepan G (2016) Chatter suppression techniques in metal cutting. CIRP Ann Manuf Technol 65:785–808
Zahoor S, Mufti NA, Saleem MQ, Mughal MP, Qureshi MAM (2017) Effect of machine tool’s spindle forced vibrations on surface roughness, dimensional accuracy, and tool wear in vertical milling of AISI P20. Int J Adv Manuf Technol 89:3671–3679
Bagci E, Aykut S (2005) A study of Taguchi optimization method for identifying optimum surface roughness in CNC face milling of cobalt-based alloy (stellite 6). Int J Adv Manuf Technol 29:940–947
Kuram E, Ozcelik B (2016) Micro-milling performance of AISI 304 stainless steel using Taguchi method and fuzzy logic modelling. J Intell Manuf 27:817–830
Costa D, Belinato G, Brito T, Paiva A, Ferreira J, Balestrassi P (2017) Weighted principal component analysis combined with Taguchi’s signal-to-noise ratio to the multiobjective optimization of dry end milling process: a comparative study. J Braz Soc Mech Sci Eng 39:1663–1681
Tlhabadira I, Daniyan IA, Machaka R, Machio C, Masu L, VanStaden LR (2019) Modelling and optimization of surface roughness during AISI P20 milling process using Taguchi method. Int J Adv Manuf Technol 102:3707–3718
Geethapriyan T, Muthuramalingam T, Kalaichelvan K (2019) Influence of process parameters on machinability of inconel 718 by electrochemical micromachining process using TOPSIS technique. Arab J Sci Eng 44(9):7945–7955
Huo J, Liu S, Wang Y (2019) T Muthuramalingam, Vu Ngoc Pi, "influence of process factors on surface measures on electrical discharge machined stainless steel using TOPSIS". Mater Res Express 6(8):086507
Manav C, Bank HS, Lazoglu I (2013) Intelligent toolpath selection via multi-criteria optimization in complex sculptured surface milling. J Intell Manuf 24(2):349–355
Arruda EM, Paiva AP, Brandão LC, Ferreira JR (2019) Robust optimisation of surface roughness of AISI H13 hardened steel in the finishing milling using ball nose end mills. Precis Eng 60:194–214
Mia M, Al Bashir M, Awal Khan MD, Dhar NR (2017) Optimization of MQL flow rate for minimum cutting force and surface roughness in end milling of hardened steel (HRC 40). Int J Adv Manuf Technol 89:675–690
PAWLAK K (2015) A review of high-strength wear-resistant steel–HARDOX. Politechnika Wrocławska, Wydział Mechaniczny, Katedra Materiałoznawstwa, Wytrzymałości i Spawalnictwa, ul. Smoluchowskiego, 25, pp.50–370
ŁĘTKOWSKA B (2013) Wpływ obróbki cieplnej na strukturę i wybrane własności stali gatunku B27 oraz 28MCB5, Rozprawa doktorska, Politechnika Wrocławska, Wrocław
Information materials SSAB (2015). Available online: https://www.ssab.com/
Baban CF, Baban M, Radu IE (2008) Reliability improvement of deformation tools with the Taguchi robust design. In: Annual reliability and maintainability symposium, RAMS 2008. IEEE, pp 218–223
Reddy SM, Reddy AC (2013) Influence of process parameters on residual stresses induced by milling of aluminum alloy using Taguchi’s. Tech IJME 6(2):69–74
Moayyedian M, Derakhshandeh JF, Lee SH (2019) Optimization of strain measurement procedure based on fuzzy quality evaluation and Taguchi experimental design. SN Appl Sci 1(11):1392
Shen C, Wang L, Cao W, Qian L (2007) Investigation of the effect of molding variables on sink marks of plastic injection molded parts using Taguchi DOE technique. Polym Plast Technol Eng 46(3):219–225
Moayyedian M, Abhary K, Marian R (2016) The analysis of defects prediction in injection molding. Int J Mech Mechatron Eng 10(12):1883–1886
Amer Y, Moayyedian M, Hajiabolhasani Z, Moayyedian L (2013) Improving injection moulding processes using experimental design (Doctoral dissertation, WASET-world academy of science engineering a)
Moayyedian M, Abhary K, Marian R (2015) Improved gate system for scrap reduction in injection molding processes. Procedia Manuf 2:246–250
Moayyedian M (2018) Intelligent optimization of mold design and process parameters in injection molding. Springer
Yang K, Basem S, El-Haik B (2003) Design for six sigma. McGraw-Hill, New York, pp 184–186
Author information
Authors and Affiliations
Corresponding author
Ethics declarations
Conflict of interest
The authors declare that they have no conflict of interest.
Additional information
Publisher's Note
Springer Nature remains neutral with regard to jurisdictional claims in published maps and institutional affiliations.
Rights and permissions
About this article
Cite this article
Moayyedian, M., Mohajer, A., Kazemian, M.G. et al. Surface roughness analysis in milling machining using design of experiment. SN Appl. Sci. 2, 1698 (2020). https://doi.org/10.1007/s42452-020-03485-5
Received:
Accepted:
Published:
DOI: https://doi.org/10.1007/s42452-020-03485-5