Abstract
Introduction
This study aimed to determine if data mining methodologies could identify reproducible predictors of dapagliflozin-specific treatment response in the phase 3 clinical program dataset.
Methods
Baseline and early treatment response variables were selected and data mining used to identify/rank all variables associated with reduction in glycated hemoglobin (HbA1c) at week 26. Generalized linear modeling was then employed using an independent dataset to identify which (if any) variables were predictive of dapagliflozin-specific treatment response as compared with treatment response in the study’s control arm. The most parsimonious (i.e., simplest) model was validated by meta-analysis of nine other trials. This staged approach was used to minimize risk of type I errors.
Results
From the large dataset, 22 variables were selected for model generation as potentially predictive for dapagliflozin-specific reduction in HbA1c. Although baseline HbA1c was the variable most strongly associated with reduction in HbA1c at study end (i.e., the best prognostic variable), baseline fasting plasma glucose (FPG) was the only predictive dapagliflozin-specific variable in the model. Placebo-adjusted treatment effect of dapagliflozin plus metformin vs. metformin alone for change in HbA1c from baseline was −0.65% at the average baseline FPG of 192.3 mg/dL (10.7 mmol/L). This response changed by −0.32% for every SD [57.2 mg/dL (3.2 mmol/L)] increase in baseline FPG. Effect of baseline FPG was confirmed in the meta-analysis of nine studies, but the magnitude was smaller. No other variable was independently predictive of a dapagliflozin-specific reduction in HbA1c.
Conclusions
This methodology successfully identified a reproducible baseline predictor of differential response to dapagliflozin. Although baseline FPG was shown to be a predictor, the effect size was not of sufficient magnitude to suggest clinical usefulness in identifying patients who would uniquely benefit from dapagliflozin treatment. The findings do support potential benefit for dapagliflozin treatment that is consistent with current recommended use.
Similar content being viewed by others
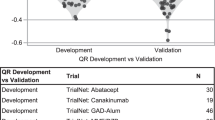
Avoid common mistakes on your manuscript.
Introduction
Choosing among the many antihyperglycemic treatment options now available for patients with type 2 diabetes mellitus (T2DM) involves matching the clinical profile of each drug, which has been assessed using aggregate data in clinical trials, to the characteristics of the individual patient [1]. In practice, the relevant parameters involve tolerability and safety; for example, whether the treatment exposes the patient to hypoglycemia or if the patient has renal impairment. Relatively little is known about the differential efficacy of a drug on a patient-by-patient basis, and the factors that might underlie differential responses are not well understood [2, 3].
Dapagliflozin, a sodium-glucose co-transporter 2 inhibitor (SGLT2) approved for use in the EU, US, and numerous other countries, has been shown to reduce hyperglycemia consistently by increasing urinary glucose excretion [4]. Additionally, dapagliflozin has been associated with reductions in body weight and blood pressure, and an incidence of adverse events comparable with those seen in control arms in a diverse patient population from an extensive clinical trial program [5–10]. The dapagliflozin development program, which included a large number of patients from independent clinical trials, provided the opportunity to explore the possibility that baseline characteristics or early treatment responses might predict which patients would most benefit from dapagliflozin therapy.
We used data mining—a computational process used to identify patterns in complex datasets—to extract clinically useful information from dapagliflozin phase 3 trials that might otherwise have remained unknown. Data mining algorithms are used to interrogate data to develop a classification rule that can be predictive for outcomes of interest [11]. They feature extensively in handling very large datasets, where such a hypothesis-independent approach has delivered particularly innovative insights. To date, there are limited examples of the use of such applications to identify predictive variables within conventional clinical datasets, as generated during late-stage clinical trials [12]. A comprehensive analysis of the dapagliflozin phase 3 program was undertaken to determine whether there are baseline characteristics or early responses to treatment that could be used to predict which patients would benefit the most from receiving dapagliflozin treatment in conjunction with other treatments administered in the program.
Methods
The overall analysis comprised three stages: (1) variable selection, (2) model generation, and (3) clinical validation (Fig. 1). Each stage used data from independent clinical trials within the phase 3 program. Studies were selected for analysis if they had a dapagliflozin arm and had been completed by the time this analysis was initiated; all studies fulfilling these criteria were used in these analyses (Table 1) [5, 9, 13–19].
The study was designed with expert clinical, personalized healthcare, statistical, and informatics input. All analysis methods and variable selection criteria were agreed a priori and were captured in an exploratory analysis plan.
This article does not contain any new studies with human or animal subjects performed by any of the authors.
Variable Selection
The variable selection stage was performed on data from the metformin plus dapagliflozin arm of a randomized, 52-week, double-blind, active-controlled non-inferiority study of dapagliflozin vs. glipizide as add-on to metformin therapy in patients with T2DM with inadequate glycemic control on metformin alone [8]. The primary endpoint of this study was change in glycated hemoglobin (HbA1c) from baseline to week 26. In the studies used in the later stages, the primary endpoint was measured at week 24 instead of week 26. Missing 26-week data were imputed using the last observation carried forward technique. Similarly, the early post-treatment time point was week 3, but because studies used in subsequent stages of the analysis collected data at week 4, week 4 data were used in both the model generation and validation stages. The goal at this stage was to identify those baseline and early treatment response variables with the largest influence on change in HbA1c level. These variables were then ranked based on the strength of their association with the endpoint. Due to a lack of a control arm (metformin alone) in this specific dataset, it was not possible to assess which variables would be specific predictors of dapagliflozin treatment response per se as opposed to more general prognostic factors. The term prognostic as used here has virtually the same meaning as in routine clinical medicine; namely, baseline or early response characteristics that influence outcome, independent of treatment. In contrast, a predictor is a baseline or early response characteristic that has an impact on response to a particular treatment.
The variables selected for model generation were determined by combining the variable lists from two different data mining methods, gradient boosting [20, 21] and elastic net [22], using a set of data-driven guidelines. These two methods were selected to complement each other. (For elastic net, the most influential variables were identified as the variables selected when the regularization parameter lambda was increased to the highest level achieving a cross-validated mean squared error (CV MSE) within 1 standard error of the lowest CV MSE, and for gradient boosting it was the top ranked variables with the cutoff determined by a noticeable drop in relative influence score). Two variable lists were defined: clinically relevant data available at baseline, and clinically relevant data available at baseline plus data available at the early post-treatment time point.
Model Generation
The purpose of model building was to refine the selected variables by eliminating prognostic variables (i.e., those that are associated with response independent of treatment) and false positives (i.e., those that were positive in the variable selection phase only) to build a model predictive of dapagliflozin-specific HbA1c reduction at 24 weeks. The lists of variables identified in the variable selection stage (Table 2) were used for model generation in an independent dataset. During this stage, the purpose was to build a robust predictive model that would include variables with estimated effect sizes large enough to be clinically meaningful. Model generation was carried out in a placebo-controlled dataset, allowing variables predictive of response to dapagliflozin treatment specifically to be distinguished from those variables that were predictive of response to any treatment.
The dataset comprised two randomized, 24-week, double-blind, active-controlled studies comparing the combinations of dapagliflozin (5 or 10 mg) plus metformin with dapagliflozin plus placebo or metformin plus placebo [10]. The modeling comprised further variable selection, model refinement, and assessment of model performance for patient segmentation. Both univariate and multivariate stepwise linear regression techniques were used to model the main effects of treatment, each clinical variable, and each treatment by clinical variable interaction term. The SAS (SAS institute, SAS Foundation v9. 2, Cary NC, USA) software package was used for these analyses.
The most parsimonious model (i.e., the simplest model which best described the predictive relationship with dapagliflozin treatment response) was put forward for validation.
Clinical Validation
The selected model was validated using a meta-analysis of nine phase 3 placebo-controlled trials in patients receiving various treatments, such as insulin, glimepiride, pioglitazone, metformin, or sitagliptin (Table 1). Because we were trying to identify predictors of dapagliflozin response that would be valid for virtually any patient with T2DM, it was important to include trials that were heterogeneous with respect to concomitant treatments as well as to the demographic and disease characteristics of the trial participants. The primary aim was to use a robust method to estimate the predictive effect of fasting plasma glucose (FPG) in the remaining individual studies, from which we derived an overall estimate using a meta-analytic approach.
The meta-analysis was conducted using Bayesian hierarchical modeling, which accounts for any heterogeneity between trials by adaptively fitting the data from different trials based on their similarity. This methodology allows inferences to be drawn at the level of individual trials and for the entire set of trials [23].
Results
Variable Selection Phase
Data from 400 patients with a value for their change in HbA1c at week 26, including 46 variables of clinical relevance at baseline, were used in the variable selection stage. An additional 11 explanatory variables representing change at week 3 from baseline were used in the baseline plus early follow-up dataset. Variables included patient demographics, baseline lipids, kidney function, HbA1c, FPG, and insulin resistance and sensitivity. A total of 14 baseline variables and 8 additional baseline plus week 3 variables were selected for model generation based on the strength of their association with reduction in HbA1c at week 26 (Table 2). Of these, only 17 variables were carried forward to the model generation phase because 5 of the variables chosen were not included in the dataset of the 2 studies used for model generation. Prominent among the baseline variables put forward for validation were HbA1c and FPG, and among the early response variables were change from baseline to week 3 in HbA1c and in FPG.
Model Generation Phase
Modeling identified two variables that could have independent predictive value. For change from baseline in HbA1c at week 24, baseline FPG and race were found to significantly influence the effect of dapagliflozin treatment in the two studies. Other baseline and early post-treatment time point variables were either found to be covariates, rather than predictors of dapagliflozin-specific treatment response (e.g., HbA1c), or did not replicate in the independent dataset and were probably false positives (e.g., urinary glucose concentration).
A linear relationship between baseline FPG and outcome was modeled, which suggested that the placebo-adjusted response to dapagliflozin treatment was greater in patients with high FPG at baseline compared with those with lower levels. This effect remained after adjustment for a number of prognostic covariates (main effects) including baseline HbA1c, which was the strongest prognostic factor. Further analysis of patients in the highest tertile of baseline FPG [≥220 mg/dL (12.2 mmol/L)] indicated that there was no difference in demographics or adverse event profile in these patients compared with patients with lower baseline FPG, for whom the model predicts lower efficacy.
An independent predictive effect of race was identified, which suggested that African American patients may benefit more from dapagliflozin treatment than white and Asian patients. No other predictive effect of race was found. However, the subgroup of African American patients (with an HbA1c measurement on treatment) was small (n = 29, 4%), resulting in imprecise estimates of treatment response. This limitation precluded consideration of race as a predictive variable and it was not advanced to the validation phase of the analysis. Moreover, the number of African Americans in the nine studies included in the meta-analysis was too small (ranging from 2.1 to 5.9% of the study populations) to be able to validate a proposed model.
The linear model, limited to the single variable FPG, was used to predict the effect of metformin plus dapagliflozin compared with metformin alone for all white (n = 628, 81%) and Asian patients (n = 119, 15%) in the study with HbA1c measurement on treatment. The placebo-adjusted treatment effect (i.e., change in HbA1c from baseline to week 24 of dapagliflozin plus metformin vs. metformin alone) in this model was estimated to be –0.65% (95% CI −0.84 to –0.47), with average baseline FPG of 192.3 mg/dL (10.7 mmol/L) (Fig. 2). The predicted placebo-adjusted HbA1c response varied according to the level of baseline FPG, from a treatment benefit of –0.25% (95% CI –0.55 to –0.05) for 120 mg/dL (6.7 mmol/L) baseline FPG to –1.36% (95% CI –1.82 to –0.90) for 320 mg/dL (17.8 mmol/L) baseline FPG (Fig. 2). These estimates of the effect sizes of dapagliflozin treatment based on baseline FPG were not affected by the omission of the prognostic factors from the model.
Model of fasting plasma glucose (FPG) as predictor (In White and Asian patients n = 747). bDapagliflozin treatment effect increases by 32% for every one unit standard deviation [57.2 mg/dL (3.2 mmol/L)] increase in baseline FPG; dotted lines represent the 95% confidence interval. HbA 1c glycated hemoglobin
Clinical Validation Phase
A meta-analysis technique used to assess the interaction between baseline FPG and treatment in nine dapagliflozin studies (Table 1) indicated that patients with higher levels of baseline FPG were repeatedly found to have a greater response to dapagliflozin treatment, on average, compared with patients with lower baseline FPG levels. The observed effects were consistent with the initial hypothesis derived from the first two stages of the project, but the overall estimate from the Bayesian hierarchical model was smaller and was not statistically significant (a median additional effect of dapagliflozin of −0.12% change in HbA1c at 24 weeks for every additional 50 mg/dL of baseline FPG; 95% credible interval crossed 0, Fig. 3). In addition, there was variability in the effect size estimates across the nine different studies (Fig. 3) that led to wide confidence limits around the overall estimate derived from the meta-analysis.
Effect of baseline FPG on change in HbA1c from baseline to week 24. cThese studies are shown for reference only and were not included in the overall analysis. CVD cardiovascular disease, DPP4 dipeptidyl peptidase-4, FPG fasting plasma glucose, HbA 1c glycated hemoglobin, Met metformin, SU sulfonylurea, T2DM type 2 diabetes mellitus, TZD thiazolidinediones
Discussion
A comprehensive analysis of the dapagliflozin phase 3 program was undertaken with the goal of identifying and validating baseline characteristics (or early responses to treatment) that could be used to predict which patients would respond best to dapagliflozin treatment. We used a novel, customized approach of response profiling to identify predictors of dapagliflozin efficacy of clinical value in the treatment of patients with T2DM. From an initial group of 48 variables, of which 28 were selected for modeling, only one variable, FPG, was found to significantly predict response to dapagliflozin. Although the results of the meta-analysis indicate that baseline FPG was reproducibly predictive of the effect of dapagliflozin on HbA1c change from baseline to 24 weeks, the magnitude and wide confidence interval of the effect size observed was neither clinically nor statistically significant, giving little potential scope for use as a clinical predictor of efficacy. Baseline HbA1c, which was found to be the strongest prognostic variable in our analyses (i.e., associated independently of treatment with the largest change in HbA1c from baseline to week 24/26), was not an independent predictor of dapagliflozin-specific treatment effect.
Our results support the findings of conventional, hypothesis-driven analyses, which have shown that dapagliflozin offers significant clinical benefit across all groups of patients in a broad-based clinical trial program, including patients across the continuum of T2DM, from treatment naïve to those requiring high doses of insulin [24, 25]. It is also consistent with previously published evidence showing that the beneficial effect of dapagliflozin therapy in terms of reduction from baseline in HbA1c is greatest in those with the highest baseline HbA1c [6]. The methodology applied was sufficiently sensitive to detect a signal of a predictive marker for differential response to dapagliflozin that was below a threshold of clinical significance, suggesting that the model would have been able to detect a clinically relevant predictor if one were included in the original set of variables evaluated in this analysis. Given the breadth of the clinically available data captured in the clinical trials databases and the thoroughness of this analysis, however, it is unlikely that we would have failed to include a potentially relevant clinically available variable in this large set of variables. Our preliminary conclusion, therefore, is that there are no subgroups identifiable from baseline or from baseline plus early on-treatment data in dapagliflozin studies that are associated with clinically relevant differential response to dapagliflozin treatment.
We attempted to combine complementary statistical methods for data mining to cast a wide net for potential signals. Elastic nets [22] allow an efficient handling of correlated variables, while decision tree algorithms, such as gradient boosting [20, 21], are most suitable for the analysis of complex interactions and heterogeneity. Combined, these two methods complement each other and give a good chance of finding predictive variables. Such an approach, which poses a risk of type I error, was controlled for by using a staged approach (i.e., hypothesis generation, testing, and validation) and multiple independent datasets. The study described by Maeda et al. [12] evaluating whether baseline HbA1c, post-prandial glucose, body mass index, and duration of diabetes may be predictors of HbA1c reduction when using sitagliptin in Japanese patients with T2DM, for example, was less informative. This was because it only involved one stage and could not distinguish between prognostic and predictive effects specific to sitagliptin, as no control arm was included. In addition, control over type I error was limited and there was no indication of clinical relevance [12].
The strengths of the approach described herein are its hypothesis-independent basis and consequent ability to generate truly innovative insights. This approach was deliberately chosen to allow all studies to be analyzed together and to identify any variables that would be predictive of response across studies and across the entire spectrum of patients with T2DM. Because the full range of baseline and early on-treatment data were considered as variables that could potentially affect treatment response, the analysis was not limited to those that have a plausible rationale; therefore, the potential to discover a completely novel predictor was increased.
The weaknesses of the method are predicated on the same basis and are exemplified by the high false discovery rates requiring independent validation to deliver sufficient confidence. One way of partially overcoming this problem would be to use a dapagliflozin add-on study that has a placebo-control group for the variable selection phase, which would facilitate identification of possible dapagliflozin-specific effects and reduce the false discovery rate. A second limitation is a consequence of the fact that our approach necessitated the measurement of factors across the complete program of studies. Because the studies that comprised the phase 3 clinical development program for dapagliflozin were relatively heterogeneous by design, the results of meta-analysis of nine of the 12 studies is not meaningful in its own right and should therefore be interpreted with caution. In fact, as shown in Fig. 3, the estimated effect size of FPG as a predictor was quite large (approximately 1 standard deviation) in treatment-naïve patients in the monotherapy study [26], whereas it was estimated to be essentially nil in the two studies of older patients with cardiovascular disease [15, 17]. Although pooling of studies that were all similar in design would have mitigated the problems associated with study heterogeneity, the realities of clinical development programs make this an unavoidable limitation of dealing with these data sets.
In conclusion, our findings are consistent with those of conventional, hypothesis-driven analyses that dapagliflozin offers significant and predictable clinical benefit across all groups of patients, from treatment naïve to those requiring high doses of insulin [24, 25]. Furthermore, we suggest that this hypothesis-independent approach may be applied to other drugs for which substantial clinical data are available.
References
Inzucchi SE, Bergenstal RM, Buse JB, et al. Management of hyperglycaemia in type 2 diabetes: a patient-centered approach. Position statement of the American Diabetes Association (ADA) and the European Association for the Study of Diabetes (EASD). Diabetologia. 2012;55:1577–96.
Johnson JA. Ethnic differences in cardiovascular drug response: potential contribution of pharmacogenetics. Circulation. 2008;118:1383–93.
Ma Q, Lu AY. Pharmacogenetics, pharmacogenomics, and individualized medicine. Pharmacol Rev. 2011;63:437–59.
Marsenic O. Glucose control by the kidney: an emerging target in diabetes. Am J Kidney Dis. 2009;53:875–83.
Bailey CJ, Gross JL, Pieters A, Bastien A, List JF. Effect of dapagliflozin in patients with type 2 diabetes who have inadequate glycaemic control with metformin: a randomised, double-blind, placebo-controlled trial. Lancet. 2010;375:2223–33.
Ferrannini E, Ramos SJ, Salsali A, Tang W, List JF. Dapagliflozin monotherapy in type 2 diabetic patients with inadequate glycemic control by diet and exercise: a randomized, double-blind, placebo-controlled, phase 3 trial. Diabetes Care. 2010;33:2217–24.
List JF, Woo V, Morales E, Tang W, Fiedorek FT. Sodium-glucose cotransport inhibition with dapagliflozin in type 2 diabetes. Diabetes Care. 2009;32:650–7.
Nauck MA, Del PS, Meier JJ, et al. Dapagliflozin versus glipizide as add-on therapy in patients with type 2 diabetes who have inadequate glycemic control with metformin: a randomized, 52-week, double-blind, active-controlled noninferiority trial. Diabetes Care. 2011;34:2015–22.
Strojek K, Yoon KH, Hruba V, Elze M, Langkilde AM, Parikh S. Effect of dapagliflozin in patients with type 2 diabetes who have inadequate glycaemic control with glimepiride: a randomized, 24-week, double-blind, placebo-controlled trial. Diabetes Obes Metab. 2011;13:928–38.
Henry RR, Murray AV, Marmolejo MH, Hennicken D, Ptaszynska A, List JF. Dapagliflozin, metformin XR, or both: initial pharmacotherapy for type 2 diabetes, a randomised controlled trial. Int J Clin Pract. 2012;66:446–56.
Ozery-Flato M, Parush N, El-Hay T, et al. Predictive models for type 2 diabetes onset in middle-aged subjects with the metabolic syndrome. Diabetol Metab Syndr. 2013;5:36.
Maeda H, Kubota A, Tanaka Y, Terauchi Y, Matsuba I. The safety, efficacy and predictors for HbA1c reduction of sitagliptin in the treatment of Japanese type 2 diabetes. Diabetes Res Clin Pract. 2012;95:e20–2.
Bailey CJ, Gross JL, Hennicken D, Iqbal N, Mansfield TA, List JF. Dapagliflozin add-on to metformin in type 2 diabetes inadequately controlled with metformin: a randomized, double-blind, placebo-controlled 102-week trial. BMC Med. 2013;11:43.
Bolinder J, Ljunggren Ö, Kullberg J, et al. Effects of dapagliflozin on body weight, total fat mass, and regional adipose tissue distribution in patients with type 2 diabetes mellitus with inadequate glycemic control on metformin. J Clin Endocrinol Metab. 2012;97:1020–31.
Cefalu WT, Leiter LA, Debruin TW, Gause-Nilsson I, Sugg JE, Parikh SJ. Dapagliflozin treatment for type 2 diabetes mellitus patients with comorbid cardiovascular disease and hypertension. Diabetes. 2012;61(suppl 1):A271. (Abstract 1056-P).
Jabbour S, Hardy E, Sugg JE, Parikh SJ. Dapagliflozin as add-on therapy to sitagliptin with or without metformin: a randomized, double-blind, placebo-controlled study. Diabetes. 2012;61(suppl 1):A275–6. (Abstract 1071-P).
Leiter LA, Cefalu WT, Debruin TW, Gause-Nilsson I, Sugg JE, Parikh SJ. Efficacy and safety of dapagliflozin for type 2 diabetes mellitus patients with a history of cardiovascular disease. Diabetes. 2012;61(suppl 1):A287. (Abstract 1114-P).
Rosenstock J, Vico M, Wei L, Salsali A, List JF. Effects of dapagliflozin, an SGLT2 inhibitor, on HbA(1c), body weight, and hypoglycemia risk in patients with type 2 diabetes inadequately controlled on pioglitazone monotherapy. Diabetes Care. 2012;35:1473–8.
Wilding JP, Woo V, Soler NG, et al. Long-term efficacy of dapagliflozin in patients with type 2 diabetes mellitus receiving high doses of insulin: a randomized trial. Ann Intern Med. 2012;156:405–15.
Freund Y, Schapire RE. Experiments with a new boosting algorithm. In: Saitta L, editor. Machine Learning: Proceedings of the Thirteenth International Conference (ICML ‘96). Burlington: Morgan Kaufmann Publishers; 1996. p. 156–8.
Friedman JH. Greedy functions approximation: a gradient boosting machine. Ann Stat. 2001;29:1189–232.
Zou H, Hastie T. Regularization and variable selection via the elastic net. J R Stat Soc B Stat Methodol. 2005;67:301–20.
Rossi PE, Alenby GM, McCulloch R. Bayesian statistics and marketing. Chichester: Wiley; 2005.
Gerich JE, Bastien A. Development of the sodium-glucose co-transporter 2 inhibitor dapagliflozin for the treatment of patients with type 2 diabetes mellitus. Expert Rev Clin Pharmacol. 2011;4:669–83.
Bristol-Myers Squibb, AstraZeneca. Forxiga Product Information. Australian Government Department of Health and Ageing-Therapeutic Goods Administration website. http://www.tga.gov.au/pdf/auspar/auspar-dapagliflozin-propanediol-monohydrate-130114-pi.pdf. Accessed July 8, 2013.
Bailey CJ, Iqbal N, T’joen C, List JF. Dapagliflozin monotherapy in drug-naive patients with diabetes: a randomized-controlled trial of low-dose range. Diabetes Obes Metab. 2012;14:951–9.
Acknowledgments
Sponsorship and article processing charges for this study were funded by AstraZeneca and Bristol-Myers Squibb. The authors would like to thank Aruna Bansal and Joan Sopczynski for execution of analyses contained within this paper. Medical writing assistance was provided by Ray Ashton and Bill Kadish of PPSI, Hackensack, New Jersey, USA (a PAREXEL company) and was funded by AstraZeneca. Anne-Marie Boothman, Angelo Del Parigi, Susan Grandy, and Chris Chamberlain made substantial contributions to the conception and design and interpretation of data. Sarah Bujac and Martin Karpefors made substantial contributions to the conception and design, analysis, and interpretation of data. Jennifer Sugg made a substantial contribution to the conception and design, acquisition of data, and analysis and interpretation of data. Tom Liptrot made a substantial contribution to the design, analysis and interpretation of data. All named authors meet the ICMJE criteria for authorship for this manuscript, take responsibility for the integrity of the work as a whole, and have given final approval for the version to be published.
Conflict of interest
Anne-Marie Boothman is an employee of AstraZeneca. Sarah Bujac is an employee of AstraZeneca. Jennifer Sugg is an employee of AstraZeneca. Susan Grandy is an employee of AstraZeneca. Martin Karpefors is an employee of AstraZeneca. Tom Liptrot and Angelo Del Parigi were employees of AstraZeneca at the time of the analysis. Chris Chamberlain was an employee of AstraZeneca at the time of the analysis, but is currently an employee of UCB SA, Slough, UK.
Compliance with ethics guidelines
This article does not contain any new studies with human or animal subjects performed by any of the authors.
Open Access
This article is distributed under the terms of the Creative Commons Attribution Noncommercial License which permits any noncommercial use, distribution, and reproduction in any medium, provided the original author(s) and the source are credited.
Author information
Authors and Affiliations
Corresponding author
Electronic supplementary material
Below is the link to the electronic supplementary material.
Rights and permissions
Open Access This article is distributed under the terms of the Creative Commons Attribution 4.0 International License (https://creativecommons.org/licenses/by/4.0), which permits use, duplication, adaptation, distribution, and reproduction in any medium or format, as long as you give appropriate credit to the original author(s) and the source, provide a link to the Creative Commons license, and indicate if changes were made.
About this article
Cite this article
Bujac, S., Del Parigi, A., Sugg, J. et al. Patient Characteristics are not Associated with Clinically Important Differential Response to Dapagliflozin: a Staged Analysis of Phase 3 Data. Diabetes Ther 5, 471–482 (2014). https://doi.org/10.1007/s13300-014-0090-y
Received:
Published:
Issue Date:
DOI: https://doi.org/10.1007/s13300-014-0090-y