Abstract
Projected warming and drying trends over the Mediterranean region represent a substantial threat for wheat production. The present study assesses winter wheat yield response to potential climate change and estimates the quantitative effectiveness of using early flowering cultivars and early sowing dates as adaptation options for the major wheat production region of Portugal. A crop model (STICS) is used for this purpose, which is calibrated for yield simulations before projecting future yields. Climate projections over 2021–2050 and 2051–2080 under two emission scenarios (RCP4.5 and RCP8.5) are retrieved from bias-adjusted datasets, generated by a ten-member climate model ensemble. Projected intensification of water deficits and more frequent high-temperature events during late spring (April–June), coinciding with the sensitive grain filling stage, primarily result in continuous mean yield losses (relative to 1981–2010) by − 14% (both scenarios) during 2021–2050 and by − 17% (RCP4.5) or − 27% (RCP8.5) during 2051–2080, also accompanied by increased yield variabilities. Of evaluated adaptation options at various levels, using earlier flowering cultivars reveals higher yield gains (26–38%) than that of early sowings (6–10%), which are able to reverse the yield reductions. The adopted early flowering cultivars successfully advance the anthesis onset and grain filling period, which reduces or avoids the risks of exposure to enhanced drought and heat stresses in late spring. In contrast, winter warming during early sowing window could affect vernalization fulfillment by slowing effective chilling accumulation, thus increasing the pre-anthesis growth length with limited effects on advancing reproductive stage. Crop yield projections and explored adaptation options are essential to assess food security prospects (availability and stability) of dry Mediterranean areas, providing crucial insights for appropriate policymaking.
Similar content being viewed by others
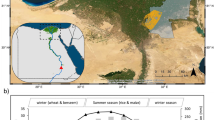
Avoid common mistakes on your manuscript.
1 Introduction
How to improve agricultural production to meet projected increasing demand of global food products by around 60% until 2050, due to growing population and economic development, represents a substantial challenge, particularly in the context of climate change (Alexandratos and Bruinsma 2012). Projected anthropogenic-driven climate change, with elevated atmospheric CO2 level, rising surface air temperatures, and changes in local precipitation regimes, is expected to adversely affect crop growth and yields in many parts of the world (IPCC 2013), bringing numerous uncertainties and risks for agricultural production and food security (Schmidhuber and Tubiello 2007).
Wheat (Triticum aestivum L.) is the staple crop throughout the world, and Portugal is a country that still highly depends on the importation of wheat, e.g., used as fodder crop in many dairy farms. In this context, satisfying internal demands via increased domestic production may play a vital social-economic role (Páscoa et al. 2017). Wheat production is mainly concentrated in southern Portugal, namely in the Alentejo region, which contributes to more than 75% of national wheat production (INE 2018). In Alentejo, the prevalence of dryland farming systems leads to wheat cultivation under rainfed conditions (Valverde et al. 2015). Approximately, 95% of wheat growing areas in Alentejo are devoted to bread wheat production (Gouveia and Trigo 2008). The typical Mediterranean climate in this region causes a high evaporative demand in late spring (ca. April–June) when precipitation is low, considerably enhancing the risks of occurrence of severe water deficit during the most susceptible growth stage of winter wheat, i.e., flowering and post-anthesis grain filling period (Costa et al. 2013; Páscoa et al. 2017). A previous analysis for this region revealed climatic water deficits in May and June, largely coinciding with the grain filling and ripening stages, could impose strong limitation on wheat yields (Páscoa et al. 2017). Moreover, such a critical growing period is also frequently exposed to extremely high temperatures, with clear detrimental effects on final grain yield (Dias and Lidon 2009; Scotti-Campos et al. 2014). For instance, post-anthesis high temperature (> 30 °C), which is common in Alentejo (Scotti-Campos et al. 2014), can cause significant grain yield reductions, resulting from a shortened grain filling phase and increased leaf senescence (Asseng et al. 2011; Dias and Lidon 2009). A modeling study in major wheat growing regions of Australia suggested that variations in mean growing season temperature by ± 2 °C could impose a substantial reduction on wheat grain production by up to 50% (Asseng et al. 2011). Observed climate conditions in southern Portugal have shown a clear trend toward a more arid climate, with increased mean temperature and decreased annual precipitation, particularly spring precipitation (Páscoa et al. 2017; Rolim et al. 2017; Valverde et al. 2015). The observed warming and drying trends are likely to be strengthened in future climates (Páscoa et al. 2017; Rolim et al. 2017), with a concomitant increase in the frequency and intensity of extreme weather events, e.g., droughts (Santos et al. 2016).
Adaptation measures on cropping systems have shown great potential to reduce or counteract the negative climate change impacts (Howden et al. 2007). For instance, a meta-analysis reviewing numerous studies revealed that the projected wheat yield losses, in both tropical and temperate regions, can be avoided or even reversed by implementing crop-level adaptation options, such as cultivar changes, adjusting planting date, irrigation, and residue management (Challinor et al. 2014). However, analysis of the effects of these adaption options will rely on a contextual approach (Challinor et al. 2014; Howden et al. 2007), which requires incorporating local characteristics, such as local soil properties, climatic projections, crop behavior, and common agronomic practices. Process-based crop models are efficient tools for simulating interactions among weather, soil, crop, and management practices and are increasingly used to project future crop yield and explore adaptation options in different regions worldwide (Asseng et al. 2013; Kassie et al. 2015; Wang et al. 2017). STICS is such a model, initially parameterized and validated for cereal crops (Brisson et al. 2003, 1998; Brisson et al. 2002), and has been thoroughly evaluated over a wide range of agro-environmental conditions (including Mediterranean-type climates), showing a satisfactory and robust performance in simulating growth and yield of winter wheat (Coucheney et al. 2015).
Although future climate projections are often carried out by global climate models (GCMs), their coarse spatial resolutions (100–500 km) often constrain the direct use of GCM outputs in crop models (often operated on a 1-ha basis). Dynamical downscaling is a common approach to obtain appropriate regional climate information, in which higher resolution regional climate models (RCMs) are applied within limited areas, with boundary conditions provided by coupled GCMs (IPCC 2015). Within the European branch of the global Coordinated Regional Downscaling Experiment (EURO–CORDEX) initiative, a number of RCMs, driven by large-scale outputs of GCMs under different representative concentration pathways (RCPs), were used to carry out high-resolution RCM simulations (~ 12.5 to 50 km) throughout Europe (Jacob et al. 2014). Nevertheless, raw outputs from GCM–RCM model chains still tend to have systemic errors (bias) as compared to observations, because either GCMs or RCMs are just an approximation of the earth climate system, which highlights the need for bias adjustments toward observed climatology (IPCC 2015; Yang et al. 2010). While multiple climate models are increasingly used for a comprehensive understanding of potential climate change, few studies have applied bias-corrected multi-model ensembles from high-resolution RCMs, to assess climate change impacts on crop yields.
Previous studies on potential climate change influences on wheat production in Portugal were focused on either assessing crop water deficits (Rolim et al. 2017) or using climate change projections without accounting for the potential changes in climate variabilities and associated extreme events (Valverde et al. 2015). Besides, neither of these studies attempt to explore adaptation strategies. In the present study, ten bias-corrected GCM–RCM pairs and two RCPs are used to cover both model and anthropogenic forcing uncertainties for future climate projections (IPCC 2015). STICS is calibrated using local wheat yield data before projecting future yields. We aim to (i) assess impacts of a range of climate change projections on winter wheat yield for the major wheat production region (Alentejo) of Portugal and (ii) explore consistent and suitable adaptation strategies to cope with potential climate change.
2 Data and methods
2.1 Study region and representative site
The study was performed within the Alentejo region (southern Portugal), featuring vast open areas of rolling plains, with some mountainous areas in the northeast (Fig. 1a). The area was characterized as a dry (sub-humid to semi-arid) Mediterranean climate, with extensive development of dryland farming systems, occupying ~ 63,400 ha (Valverde et al. 2015). Rainfed winter wheat was typically sown in November, with a flexible sowing window, and harvested in June of the next year (Gouveia and Trigo 2008). Pest/disease damage and weed infestation were generally well managed and controlled (Costa et al. 2013). Owing to the relatively homogeneous regional climate, the study site was chosen at Beja district (38.0° N, 7.9° W, 192 m a.s.l., Fig. 1a) to represent the dominant soil type (vertisol), where a weather station is also located < 10 km away. Standard soil physical properties were primarily obtained from local measurements (Carvalho and Basch 1995), complemented by information from the global SoilGrids dataset at 250-m resolution (Hengl et al. 2017) and Harmonized World Soil Database (~ 1-km resolution) (FAO/IIASA/ISRIC/ISSCAS/JRC 2012), which are summarized in Online Resource (OR) 1. Required soil hydraulic properties were directly obtained from EU–SoilHydroGrids (OR1), a newly developed fine-resolution (1 km) multi-player soil hydraulic database (Brigitta et al. 2017).
Study site and characterization of historical climate conditions. a Geographic location of the Beja district in southern Portugal (Alentejo region) with b average annual and monthly minimum (Tmin, °C), maximum (Tmax, °C), and mean (Tmean, °C) temperatures, precipitation sum (mm), and potential evapotranspiration (PET, mm) over the baseline period (1981–2010). Mean and standard deviation of c cumulative water deficit (precipitation minus PET, mm) and of d days (only positive error bars are plotted) with maximum temperature > 30 °C in three wheat growing phases during baseline
2.2 STICS description and calibration
In STICS, simulations for crop development and growth processes mainly involve phenological stages, leaf growth and senescence, and transformation of intercepted photosynthetic radiation into aerial biomass, followed by its partition into various organs (e.g., grain). These processes were simultaneously governed by simulated stress factors, such as water shortage or waterlogging, N deficiency, and thermal stresses. Furthermore, for winter wheat, the phenology development could also be slowed either by the sub-optimal photoperiod conditions or by non-compliance with vernalization requirement. Detailed model parameters, formalizations, and modeling approaches are available at Brisson et al. (2009).
STICS was calibrated for simulating local grain yield (15% grain moisture) of winter wheat, using available published yield data for consecutive five growing seasons (1981–1986) at Beja (Carvalho and Basch 1995) (OR2). Yield data were averaged over two experimental cultivars (Etoile and Mara) to facilitate comparison with our simulations, as no significant differences were found between them (OR2). Detailed information on experimental design and relevant inputs, such as common seeding date and rate as well as N fertilization practices, were documented by Carvalho and Basch (1995) and summarized in OR2.
For calibration, the performance of nine built-in cultivars of winter wheat was firstly examined. As only yield data was available, no attempt was made to calibrate default cultivar parameters, such as phenology and leaf area index (LAI) dynamics, but focusing only on the cultivar choice (No.1 to No.9). Subsequently, the general plant parameters, i.e., radiation use efficiency (RUE) that represented how the crop net photosynthesis was modeled (Brisson et al. 2009), were adjusted by testing a wide range of predefined values (2.25–4.25 with 0.25 interval). Various combinations of RUE with cultivar choice were thus investigated. The pair providing the best goodness-of-fit between observed and simulated yields was eventually selected. The overall approach was in agreement with standard procedures proposed by Jégo et al. (2010). The calibrated crop parameters and agronomic input values (OR2) were kept invariant in the following analysis of climate change impact.
2.3 Climate data
For the historical period of 1981–2010 (hereafter “baseline”), observed daily minimum and maximum air temperatures (°C) and precipitation (mm) were directly obtained from the Beja weather station, available at European Climate Assessment & Dataset (ECA&D, www.ecad.eu) (Klein Tank et al. 2002). Daily surface solar radiation data (MJ m−2 day−1) were extracted from both the coarse-resolution (0.75° × 0.75°) ERA-Interim reanalysis (Dee et al. 2011) and the finer-resolution (0.05° × 0.05°) satellite-based observations (CM SAF) (Pfeifroth et al. 2018). A good linear agreement was found within their overlap period (1983–2010), and the corresponding linear function was then applied to calibrate ERA-Interim data for the entire baseline period (OR3). Potential evapotranspiration (PET) was externally calculated using the FAO ET0 (v3.2) calculator. Annual records of atmospheric CO2 concentration (ppm) for baseline were retrieved from NOAA (www.esrl.noaa.gov/gmd/) and supplied as input in STICS.
The future periods of 2021–2050 (near-future) and 2051–2080 (distant-future) were selected. High-resolution (~ 12.5 km) projections for temperature (minimum and maximum) and precipitation were retrieved from ten bias-adjusted GCM–RCM simulations, produced by the EURO–CORDEX project and under RCP4.5 and RCP8.5, and for each period (10 models × 2 scenarios) (Jacob et al. 2014). RCP4.5 corresponds to an anthropogenic radiative forcing reaching 4.5 W/m2 by 2100 relative to the pre-industrial level, whereas RCP8.5 is a high-emission scenario, with a radiative forcing of 8.5 W/m2 by 2100 (van Vuuren et al. 2011). The ten GCM–RCM pairs combine five RCMs, three GCMs, and four initializations (OR4). The bias adjustment was based on distribution-based scaling approach, where corrected distribution parameters were obtained by comparing model simulations and observations during the control period (1989–2010), and then applied to adjust the frequency distribution of raw model future projections (Yang et al. 2010). Such an approach was known to better preserve projected climate variability generated by individual RCM, as well as being able to realistically consider the covariance between temperature and precipitation (Yang et al. 2010). The observation source for bias adjustments was the Mesoscale Analysis (MESAN) dataset at 3–12-km resolution throughout Europe, which was extensively used for regional reanalysis of a number of surface parameters (e.g., temperature and precipitation) (Dahlgren et al. 2016; Landelius et al. 2016). Moreover, as recommended from IPCC (2015), it is also essential to verify the performance of the bias adjustment using independent (additional) observational data (IPCC 2015). Hence, the cumulative distribution functions of monthly mean temperature and precipitation sum between local weather station data and model simulations were thus compared for the control period (OR5). An overall agreement was found, particularly for monthly precipitation (OR5), suggesting sufficient bias adjustment for individual model outputs, as well as demonstrating the relevance of regional climate projections for the local impact study.
Radiation projections were not directly retrievable from bias-adjusted model outputs, but from raw outputs of each GCM–RCM pair within EURO-CORDEX (Jacob et al. 2014). Nonetheless, the bias adjustment was still performed by firstly deriving the ratios of mean monthly radiation sum between observations and model simulations over baseline. These monthly ratios were then applied as multiplicative correction factors to the raw projections of daily radiation of the respective month. The projected radiation sum eventually shows no significant differences at monthly scale compared to baseline data (not shown). Furthermore, to account for elevated CO2 effect on crop growth and yield, the predefined future atmospheric CO2 concentrations for each scenario were considered (RCP4.5 or RCP8.5).
2.4 Exploration of adaptation strategies
Two potentially suitable adaptation strategies were proposed in an attempt to minimize exposure of the most sensitive grain filling phase (i.e., anthesis to grain maturity) to the typical unfavorable spring (April–June) conditions that were expected to be exacerbated in future climate. The first adaptation strategy assumed the genotypic development and use of earlier flowering wheat cultivar, which was suggested to be useful in avoiding critical/terminal stress conditions during reproductive stages for winter wheat under Mediterranean-type climates (Debaeke 2004; Wang et al. 2017). Simulation of a future early flowering cultivar was achieved by reducing the growing degree days (GDD) requirement between emergence and anthesis, without altering other cultivar parameters (e.g., GDD for grain filling duration) in STICS (OR2). Three different adaptation levels were set, corresponding to 10%, 20%, and 30% GDD reductions. Note that 30% reduction represents about the maximum extent of earliness to ensure no prior occurrence of anthesis over heading onset, while it still remains practical for cultivar breeding efforts. In general, this adaptation strategy tends to explore the trade-off effect between lower risk of yield limitation by drought/heat stress and higher risk of potential yield reduction with shorter growth duration (because of GDD reductions). Nevertheless, early flowering cultivars could be subject to the risk of spring frost damage with yield losses. In view of predictable climate warming, occurrence of spring frost is expected to be markedly reduced, such as the projections obtained for the wheat belt of Eastern Australia (Wang et al. 2015), thus likely being a lesser concern for yield threat under a warmer climate. The second adaptation strategy, namely early sowings, hypothesized that similar avoiding effects could be equally achieved from a management perspective, resulting from an anticipation of the growth cycle. Range of sowing dates (three different levels) was tested, namely 10, 20, and 30 days early sowing (i.e., early sowing window from Oct_30 to Nov_20 with 10-day interval) relative to the baseline adopted average sowing date of Nov_30 (OR2), without changing other parameters. Late sowings, such as sowing in late December or early January, are not considered as they were expected to notably increase the crop exposure to frequent drought and heat stresses during the sensitive grain filling period, thus leading to more yield reductions (Dias and Lidon 2009).
2.5 Statistical analysis
The comparison of STICS simulations with local observed wheat yields was statistically assessed using the following complementary metrics: normalized root mean square error (nRMSE, %), mean absolute error (MAE, kg ha−1), and correlation coefficient (r). Regarding future yield projections, Student’s independent sample t test was applied for assessing the significance of differences in means between baseline and each future period. Yield interannual variability of each period was expressed using the coefficient of variation (CV).
3 Results
3.1 Calibration for simulating wheat yield
Prediction errors (nRMSE and MAE) reveal a gradual increase as a function of RUE for individual cultivars, while differences of errors among cultivars tend to enlarge (Fig. 2a, b). The lowest nRMSE (stabilized at 20%) and MAE (432–476 kg ha−1) are consistently found for cultivar No.7 with RUE ranging from 2.75 to 3, though 2.75 should be preferentially selected to minimize cultivar differences (Fig. 2a, b). Furthermore, a robust model performance is found, i.e., simulated yields are highly correlated with observations (r > 0.75), irrespective of RUE and cultivar (Fig. 2c). Highest r is also obtained using cultivar No.7, for which r tends to stabilize with RUE > 2.75 (Fig. 2c). Hence, for the combination of cultivar No.7 and RUE = 2.75 (OR2), the simulations explain 90% of observed variance, with nRMSE of 20% and MAE of 464 kg ha−1 (Fig. 2d), which are chosen henceforth for the following analysis.
Comparison between observed yield data and simulations, with inputs from different combinations of a general plant parameter (RUE, radiation use efficiency) and STICS built-in cultivar choice (No.1 to No.9). The following evaluation metrics are considered: a nRMSE (normalized root mean square error), b MAE (mean absolute error), and c correlation coefficient (r), together with d the results from the selected combination of RUE (approximation of 2.75 to 2.8 g MJ−1 day−1) and cultivar choice (cultivar No.7–Thetalent). Refer to online resource 2 for summarized input parameters used for calibration
3.2 Baseline and projected climates
Baseline average annual mean temperature is 16.9 °C, with monthly mean temperature varying from 9.9 °C in January to 24.7 °C in August (Fig. 1b). For the growing season, mild winter temperatures (typically > 10 °C) are followed by a steady increase from 14.7 °C in April to 22.0 °C in June (end of the growing season), with average maximum temperature reaching 30.0 °C in June (Fig. 1b). The rainy season concentrates in October–March, with baseline average precipitation of 403 mm and low evaporative demand (Fig. 1b), leading to a negligible climatic water deficit (Fig. 1c). In contrast, lower spring precipitation with rising temperature results in a mean baseline climatic water deficit of − 324 mm for April–June (Fig. 1b, c). High-temperature events (daily maximum temperature > 30 °C) are also frequent during these months, with an average occurrence of 20 days in baseline (Fig. 1d).
Climate projections for the selected models and scenarios show increased annual mean temperature by an average (among models) of 0.8 °C (0.6–1.0 °C) in RCP4.5 or 1.0 °C (0.8–1.2 °C) in RCP8.5 for 2021–2050 and of 1.3 °C (1.0–1.7 °C) in RCP4.5 or 2.3 °C (2.2–2.5 °C) in RCP8.5 for 2051–2080, with respect to baseline (OR6). Projected warming rates show a remarkable asymmetry at the monthly scale, with highest mean temperature increase (by model-average) in May (up to 2.9 °C) and lowest increase in March (up to 1.7 °C) over the growing season (OR6). Further, higher temperature increases in RCP8.5 than in RCP4.5 are clearly discernible in 2051–2080 (OR6). As a result of temperature increase, mean annual PET is also increased, depending on RCP4.5 or RCP8.5, by an average of 30 or 44 mm in 2021–2050 and of 56 or 105 mm in 2051–2080, respectively (OR6). Precipitation projections indicate that annual precipitation reductions are very likely, in which projected mean precipitation changes vary from − 8 to + 7% (excluding outlier) in RCP4.5 or from − 24 to − 2% in RCP8.5 during 2021–2050 and from − 19 to − 4% (RCP4.5) or − 28 to −6% (RCP8.5) during 2051–2080 (OR6). However, monthly precipitation projections are more uncertain, e.g., varying widely from − 78 to 39% (RCP4.5) in June during 2021–2050 and from − 29 to 42% in March (RCP8.5) during 2051–2080 (OR6). Climate projections over the whole Alentejo region (as indicated by multi-model ensemble mean) also show increased annual mean temperature (up to 2.7 °C) and decreased annual precipitation (up to − 18%), revealing a regional homogeneity of climate signals for a given scenario and period (OR7).
3.3 Impacts of climate change projections on wheat yield
The simulated 30-year baseline yield (inter-quartile) ranges from 1409 to 2848 kg ha−1, with an average of 2045 kg ha−1 and a strong inter-annual variability (CV = 47%) (Fig. 3a). Future projections tend to show an overall decrease in mean yield, accompanied by enhanced variability. For RCP4.5, ensemble mean yield is of 1427–2109 kg ha−1 for 2021–2050 and of 1310–1962 kg ha−1 for 2051–2080, with mean yield reductions (relative to baseline) of − 14% and − 17%, respectively (Fig. 3a, b). Increased yield variability (CV > 47%) is projected in 2021–2050 by 50% of climate model projections and by 70% in 2051–2080 (Fig. 3a). Under RCP4.5, mean yield change under individual model projection ranges from − 25 to − 5% in 2021–2050 and − 33 to 6% (including significant reductions from − 33 to − 24%) in 2051–2080 (Fig. 3b). For RCP8.5, ensemble mean yield shows a range of 1471–2119 kg ha−1 in 2021–2050, with decreased mean yield by − 14% and of 1180–1804 kg ha−1 in 2051–2080, with significant mean yield reduction of − 27% (Fig. 3c). Increased yield variability is also projected in 2021–2050 by 50% of climate projections, whereas it is projected by all models in 2051–2080 (RCP8.5) (Fig. 3c). The range of mean yield changes varies from − 22 to 5% in 2021–2050, while significant mean yield reductions are consistently projected in 2051–2080 (RCP8.5), ranging from − 39 to − 22% (Fig. 3d). Uncertainties are higher among climate models than between scenarios, in which mean yield reductions are of − 18 to 0% for models (averaged over scenarios) and − 13 to − 11% for scenarios (averaged over models) during 2021–2050 and of − 36 to − 8% for models and − 28 to − 17% for scenarios during 2051–2080 (Fig. 3). Elevated atmospheric CO2 levels reveal limited benefits on crop yield, mitigating mean yield reductions by an average of 4% for RCP4.5 or 5% for RCP8.5 during 2021–2050 and 7% for RCP4.5 or 10% for RCP8.5 during 2051–2080 (OR8).
Projections of wheat yield under a, b RCP4.5 and c, d RCP8.5 over the near-future (2021–2050) and distant-future periods (2051–2080) under climate projections from ten models (GCM-RCM). Refer to online resource 4 for detailed information of individual climate models. Dashed lines indicate the median value of baseline yield and the diamond denotes increased yield interannual variability under respective climate model projection (left segments). Statistically significant changes (p < 0.05) of mean yield with respect to baseline are highlighted with asterisks (right segments)
3.4 Projections of water deficit and high-temperature events
Climate projections reveal a high likelihood of increased climatic water deficit and more frequent high-temperature events during April–June (Fig. 4), which are assumed as the primary drivers of the projected yield reductions and increased variability. In April–June, multi-model ensemble mean indicates significantly enhanced (increased) water deficits by − 38 (RCP4.5) or − 51 (RCP8.5) mm for 2021–2050 and by − 59 (RCP4.5) or − 90 (RCP8.5) mm for 2051–2080, with respect to baseline (Fig. 4a, b). The projected range of changes of mean water deficit during April–June primarily shows significant intensification, adding up to − 65 (RCP4.5) or − 76 (RCP8.5) mm deficits in 2021–2050 (Fig. 4a, b). Significant increases of mean water deficits are coherently found in 2051–2080 apart from one model projection, adding up to − 89 (RCP4.5) or – 107 (RCP8.5) mm deficits for this critical growing period (Fig. 4a, b). Regarding high-temperature events in April–June, ensemble means indicate significant increases by 3 (RCP4.5) or 6 (RCP8.5) days in 2021–2050 and by 8 (RCP4.5) or 14 (RCP8.5) days in 2051–2080 (Fig. 4c, d). There are significant mean increases over 2021–2050, varying from 4 to 8 days (RCP4.5) or 6 to 11 days (RCP8.5) (Fig. 4c). Until 2051–2080, 70% of the projections under RCP4.5 suggest significant increases by 7 to 12 days, while significant increases are consistently found under RCP8.5, ranging from 10 to 19 days (Fig. 4d). Note that significant mean increases of high-temperature occurrences (by up to 6 days) with enhanced water deficits (adding up to − 87 mm) are also projected during October–December, particularly over 2051–2080, but the overall effects are much less pronounced for the cool rainy season (October–March) (Fig. 4b, d).
3.5 Adaptation strategies
It is clear that projected negative climate change impacts (Fig. 3) are gradually alleviated by using 10%, 20%, and 30% earlier flowering cultivars, in which projected yield losses are offset or eventually reversed (Fig. 5a). During 2021–2050, ensemble means reveal that projected mean yield reductions of − 14% under both scenarios (without cultivar adaptation) (Fig. 3b) are mitigated to − 7% or − 2% (10% early) and are reversed to an increase of 3% or 11% (20% early) and a continuous increase of 12% or 24% (30% early), depending on RCP4.5 or RCP8.5, respectively (Fig. 5a). Likewise, during 2051–2080, ensemble means indicate that mean yield reductions of − 17% (RCP4.5) or − 27% (RCP8.5) (without cultivar adaptation) (Fig. 3d) are continuously ameliorated to − 8% or − 17% (10% early), 3% or − 6% (20% early), and 14% or 6% (30% early) (Fig. 5a). Hence, mean yield gains from no cultivar adaptation to use of up to 30% earlier flowering cultivar are of 26% (RCP4.5) or 38% (RCP8.5) for 2021–2050 and 31% (RCP4.5) or 33% (RCP8.5) for 2051–2080. Moreover, the consistent significant mean yield reductions during 2051–2080 under RCP8.5 (Fig. 3d) almost disappear by only introducing the 10% early flowering cultivar, while projected yield losses are almost reversed in 2021–2050 with the adoption of the 20% early flowering cultivar (Fig. 5a). The use of the 30% early flowering cultivar contributes to a nearly consistent increase in mean yield for both 2021–2050 and 2051–2080, during which potential increases are projected to reach up to 32% and 39%, respectively (Fig. 5a).
Effects of adaptation measures on wheat yield by using a early flowering cultivars with three different extent of earliness at anthesis (earlier than the baseline cultivar) and by using b three early sowing dates (earlier than the baseline adopted average sowing date: Nov_30) for the near-future (2021–2050) and distant-future (2051–2080) periods, under RCP4.5 and RCP8.5. Statistically significant mean yield changes (p < 0.05) with respect to baseline are highlighted with asterisks
In contrast, wheat yield seems to be less responsive to early sowings with only slight yield improvement, in which projected yield losses are unlikely to be fully counteracted under a range of climate projections (Fig. 5b). Ensemble means reveal that projected yield losses of − 14% during 2021–2050 (without early sowings) (Fig. 3b) are slightly reduced to a range of − 8 to − 4% (RCP4.5) or − 7 to − 4% (RCP8.5), following 10–30 days early sowing strategies (Fig. 5b). Similarly, mean yield reductions of − 17% (RCP4.5) or − 27% (RCP8.5) in 2051–2080 (Fig. 3d) are only marginally alleviated to a range of − 13 to − 11% (RCP4.5) or − 24 to − 19% (RCP8.5) (Fig. 5b). Thus, maximum mean yield gains by early sowings are projected to be 10% (both scenarios) in 2021–2050 and 6% (RCP4.5) or 8% (RCP8.5) in 2051–2080. There are no increases in yield gains from 10 to 30 days early sowings, particularly during 2051–2080, in which more adverse results (significant reductions up to − 27%) are found with 30 days early sowing than that of 20 days (Fig. 5b). Less favorable performance of early sowing adaptations can be largely attributed to its limited effects to advance the onset of anthesis and grain filling period to avoid intensified drought/heat stress late in the season. This could be reflected by increased pre-anthesis growth durations when sowings occur earlier than the prescribed date (Nov 30). There are robust (small variations of results among climate projections and between scenarios) mean increases of 6, 13, and 21 days (ensemble means) in phenology phase between germination and stem elongation over both 2021–2050 and 2051–2080 periods, with 10, 20, and 30 days early sowings, respectively (OR9). The extended early growth duration effectively leads to prolonged vegetative growth, in which days to anthesis are increased by an average of 8, 17, and 26 days for both 2021–2050 and 2051–2080 with 10, 20, and 30 days early sowings, respectively (OR9).
4 Discussions
4.1 Calibration performance
Soil-crop models, such as STICS, have been increasingly used as powerful tools to assess interactive effects of crop growth, climate conditions, soil properties, and management practices on yield and environment impacts on agriculture (Coucheney et al. 2015). When the model is applied to address a particular research question at a given site, calibrations of some model parameters are often firstly performed to fit simulations to available observations for better representing local production conditions. Our results indicate that an appropriate adjustment of general plant parameter and built-in cultivar choice could lead to a considerable improvement of prediction accuracy for wheat yield, where nRMSE is reduced from up to 100% to as low as 20% (Fig. 2a). In the pilot project of Agricultural Model Intercomparison and Improvement (AgMIP), a similar prediction accuracy of wheat yields (nRMSE of 30%) has been achieved using STICS under various environmental conditions, before being applied to project yield response to future climate change (Asseng et al. 2013). Furthermore, the 5-year observed yields are herein obtained under quite different meteorological conditions (including an extremely dry year) and over a wide range of possible yields, i.e., 800–4000 kg ha−1 (Fig. 2d and OR2). The model’s ability to reproduce observed yield variability, as reflected by a consistently high agreement between simulations and observations (r > 0.75, Fig. 2c), suggests that interannual sensitivity of wheat yield to weather variations could be skillfully captured by the model (in particular from extreme weather events), which may warrant its applicability in climate change impact assessments. Moreover, observed yields are directly obtained from independent field measurements of published data, thus further strengthening the reliability of our model calibrations and outcomes. However, the relevance of newly calibrated parameter values for local conditions (e.g., RUE = 2.8) should be further evaluated using additional representative datasets.
4.2 Climate projections
Climate models are widely accepted tools to simulate present and future climates. However, climate model projections are inherently uncertain, resulting from simplified representation of the real climate system by climate models with different numerical approaches for describing physical processes (IPCC 2015), from social-economic uncertainties regarding influences on future trajectories of greenhouse gas emissions (Asseng et al. 2013; van Vuuren et al. 2011), and from model initializations (Deser et al. 2012). Within the EURO-CORDEX initiative, a coordinated bias-adjusted multi-model, multi-scenario, and multi-initialization ensemble of downscaled experiments with fine spatial resolution (0.11°) was generated (Jacob et al. 2014). A subset of these model runs is employed in our study to address these uncertainties, in which the diverse ensemble composition (ten models and four initializations under two forcing scenarios) enables a wide range of probable projections. The resulting climate projections over near- and distant-future periods indeed give a relatively robust climate change signal with a small range of variations, e.g., projected annual mean temperature increase by 2.2–2.5 °C accompanied by precipitation reductions by up to − 28% in 2051–2080 under RCP8.5 (OR6). Hence, a reasonable level of confidence for climate projections has been achieved in the current study, despite some uncertainties found at a monthly scale (e.g., in June) (OR6). It is worth mentioning that these multi-model ensembles of climate projections also account for a broad range of altered climate variabilities; thus, the projected yield impacts implicitly integrate the potential changes (increase) in the frequency and intensity of extreme events.
4.3 Impacts of climate change and regional food security
The overall climate change projections depict a moderate warming and enhanced dryness with increased magnitudes as a function of time (OR6), resulting in a continuously decreased mean yield with increased variabilities (Fig. 3). During 2021–2050, projected variations of mean yield changes are relatively close between RCP4.5 (− 25 to − 5%) and RCP8.5 (− 22 to 5%), in which both scenarios agree on a mean yield reduction of − 14% (by ensemble mean) (Fig. 3b, d). The two emission scenarios indeed present relatively smaller differences in the projected trends of greenhouse gas concentrations (in particular CO2 concentration) before the 2050s, and only begin to diverge substantially in the latter half of the century, with different impacts on climate simulations (van Vuuren et al. 2011). During 2051–2080, significant decreases of mean yields (− 39 to − 22% with an ensemble mean of − 27%) are consistently found under high emission scenarios (RCP8.5), with a strong agreement concerning increased yield variabilities (Fig. 3c, d). The stabilization scenario (RCP4.5) is also likely to have a mean yield loss (− 33 to 6% with an ensemble mean of − 17%) over this period, together with the projected high likelihood (70%) of increased yield interannual variabilities (Fig. 3a, b).
The overall results are consistent with a meta-analysis of crop yield response to projected climate change, concluding that wheat yield changes are expected to be negatively affected by even moderate warming (by 2 °C of local warming), with higher risk of mean yield loss and greater yield variabilities in the second half of the twenty-first century than in the first one (Challinor et al. 2014). In southern Portugal (Guadiana river basin), a similar study also indicates the susceptibility of rainfed winter wheat to climate change, where projected mean yield reductions range from − 8 to − 4% for 2011–2040 and from − 14 to − 7% for 2041–2070, across multiple climate models and different emission scenarios (Valverde et al. 2015). In comparison, these relatively smaller magnitudes of yield losses could be attributed to the lack of introducing climate projections with altered climate variabilities, where variance of projected future climate is kept the same as in the historical baseline period (Valverde et al. 2015), which are unlikely true. In general, our findings indicate that negative yield impacts are very likely (i.e., high agreement in yield reductions with increased variabilities) despite the magnitudes of impacts that vary among models and between scenarios, which are particularly emphasized for 2051–2080 (Fig. 3). Simulated yield variations among climate model projections represent a major source of impact uncertainties when compared to variations between scenarios (Fig. 3). In fact, uncertainties in simulating yield impacts among climate model projections tend to dominate regional climate impact assessment (Kassie et al. 2015; Osborne et al. 2013). However, this can also be attributed to the asymmetry between the numbers of models (ten) and of scenarios (two) in our case. On the other hand, the simulated yield benefits from atmospheric CO2 enrichment, particularly under the high emission scenario of RCP8.5 (i.e., up to 10% mean yield mitigations) (OR8), are in contrast to reported average yield increment by about 16–22% (depending on soil water and N availability) for C3 cereals under 190 ppm CO2 increment (Kimball 2016). The limited yield response may be explained by the fact that a projected higher temperature above the optimum growth range could partially offset CO2-induced stimulation of photosynthesis, in which similar simulation results were previously obtained by Wang et al. (2017). Interactive effects of temperature and CO2 on crop photosynthesis and biomass growth are able to be captured by STICS via influences on crop RUE (Brisson et al. 2009).
The projected mean yield decrease with increased variability may undermine the two important dimensions of food security, i.e., availability and stability (Schmidhuber and Tubiello 2007). Historically, wheat production policies in Portugal encouraged increases in harvest areas, while supporting seed selection and massive use of chemical fertilizers, resulting in an intensification of cropping systems and severe soil degradation on marginal lands (Jones et al. 2011). Following the introduction of afforestation measures and policies favoring meat/milk products since the 1980s, arable crop land (including wheat areas) substantially declined with a concomitant increase of forest land and grassland areas (Jones et al. 2011). On the other hand, wheat yield increased as a result of management and cultivar improvements (Páscoa et al. 2017), as well as by abandonment of less fertile soils. However, recent common agricultural policy promotes integrated management and soil conservation practices (Jones et al. 2011); thus, yield improvements by means of intensive resource use (e.g., water and fertilizers) are likely to be more and more constrained. Hence, in the national context of growing environmental concerns on soil degradation, increasing land use competition, and restricted resource use, influence of projected wheat yield reductions shall be more pronounced, as the efforts for maintaining or increasing grain production in order to achieve self-sufficiency could be substantially undermined, provided no adaptation measures are implemented.
Annual recorded (winter) wheat yield statistics in the Alentejo region over the past three decades has been characterized by a strong variability (~ 30% of CV), ranging from 566 kg ha−1 in 2005 (associated with severe drought) to 2482 kg ha−1 in 2016 (national statistics at www.ine.pt). Other than some external factors such as technical trends and growing area changes, this variability could be largely explained by increased climate variability, particularly by the strong interannual variability of seasonal precipitation. During 1986–2012, simultaneous occurrences of dry events and anomalously low wheat yields are consistently found for most of the Iberian Peninsula (Páscoa et al. 2017), showing the vulnerability of rainfed wheat cropping systems to extreme weather conditions, particularly severe drought events. Thus, climate change is expected to further aggravate this vulnerability through increased climate variability with more aridity and frequent extreme temperature, such as projections shown in Fig. 4. As a result, the projected increase of yield interannual variabilities implies a substantial threat to future year-to-year stability of food crop supply with notable impacts to food chain resilience (Challinor et al. 2014).
4.4 Adaptation to enhanced water deficits and heat stress
Grain yield production of winter wheat in regions with typical Mediterranean climate is commonly limited by water deficits and heat stress during the flowering and grain filling period, and such unfavorable growing conditions are likely to be further worsened in the future climate (Asseng et al. 2011; Páscoa et al. 2017; Wang et al. 2017). Projected negative yield impacts in our study are largely due to the intensified water deficits and more frequent high-temperature events during the April–June period, within which grain filling phase typically occurs (Fig. 4). Significant mean increases of water deficits (− 38 to − 90 mm) and of high-temperature events (3 to 14 days) during April–June are coherently projected for the two future periods, along with smaller magnitudes of increases for the early growing season, i.e., October–March (Fig. 4). In line with our analysis, Rolim et al. (2017) suggest that average seasonal water deficits of local rainfed winter wheat are projected to increase across three climate models and two scenarios. Moreover, as indicated by Asseng et al. (2011), wheat yield losses owed to high temperatures during the important grain filling phase are likely to be an important constraint for major wheat-producing regions worldwide, thus substantially undermining global food security. In particular, our case study illustrates that average hot days (> 30 °C) during April–June are projected to increase significantly by 14 days over 2051–2080, RCP8.5 (Fig. 4d), reaching > 34 days (20 days in baseline) for this critical period with enormous detrimental impacts for successful grain production.
Between the adaptation options explored, our study reveals that the use of early flowering cultivars results in more yield gains under a range of climate projections, and thus may outperform the other adaptation measure of early sowings (Fig. 5). By adopting early flowering wheat cultivars, crop growing season lengths are expected to markedly decrease under combined effects of reduced thermal requirement and accelerated development rate under warmer climates, resulting in less intercepted nutrients and radiation, with consequently lower biomass accumulation and yield formation (Asseng et al. 2011; Debaeke 2004; Kassie et al. 2015). Nonetheless, such negative impacts of potential yield reductions with shorter growing duration are shown to be counterbalanced, with less pronounced effects than the positive effects by advancing anthesis, where risks of crop exposure to intensified drought and heat stresses during grain filling are reduced or avoided, leading to net seasonal yield gains and mitigations of mean yield reductions (Fig. 5a). Besides, a shortened vegetative phase with early flowering cultivar is also likely to result in reduced grain numbers (Farooq et al. 2011), with subsequent detrimental impacts on final grain yields, but this process is currently not incorporated in the model. The projected mean yield reductions (Fig. 3) are gradually alleviated and eventually reversed when considering cultivars with progressively early flowering, resulting in maximum yield gains of 26–38% (Fig. 5a). In many dry Mediterranean (typical winter-dominant rainfall) environments, earlier flowering has proven to enable shifting the sensitive wheat growth stage (i.e., grain filling) to the cooler and wetter part of the season, thus increasing the harvest index by minimizing the risks of exposure to terminal drought and very high temperatures late in the season (Asseng et al. 2011; Debaeke 2004; Wang et al. 2015, 2017). Moreover, the nearly consistent increases in the mean yields for both 2021–2050 and 2051–2080 (up to 39%), using 30% early flowering cultivar (Fig. 5a), may point out the potential opportunities for local yield improvement despite increasingly unfavorable climate conditions. On the other hand, Wang et al. (2017) projected increased yield of rainfed winter wheat in the warm and dry sites of Eastern Australia, benefiting from warming-induced early flowering even without cultivar adjustment. Without cultivar adaptation, our results clearly indicate negative yield response, which probably could be attributed to insufficient extent of growth advancement from projected temperature increase alone.
In contrast, 10–30 days early sowing strategy appears to be less favorable with maximum mean yield gains of only 6–10% (Fig. 5b), owing to the weak effects of advancing the onset of anthesis and grain filling stage. When sowing occurs 10, 20, and 30 days earlier, duration of pre-anthesis growth increases by an average of 8, 17, and 26 days (OR9), respectively, thus largely offsetting the effects of anticipation of the growth cycle. Most of these increases originate from the prolonged seasonal growth duration between germination and stem elongation (OR9), corresponding to the main phase for crop vernalization fulfillment (an important prerequisite for the induction of reproductive growth for winter wheat). Climate warming during the vernalization period may affect and slow effective chilling accumulation before anthesis, thus increasing the vegetative phase and delaying the onset of anthesis (Rosenzweig and Tubiello 1996; Wang et al. 2015). The flowering date of winter wheat was previously projected to be delayed by an average of 14 days under RCP8.5 in eastern Australia, resulting from restricted vernalization fulfillment with temperature increase (Wang et al. 2015). Indeed, the current mean monthly temperature (~ 15 °C) around the early sowing window (i.e., mid of October to early November) at the study area is already close to the defined upper threshold (16.5 °C) of effective chilling accumulation (vernalization value) for winter wheat (Brisson et al. 2009). Therefore, early sowing, which allows making use of more winter rainfall, may be compromised by climate warming, resulting from a decreased number of effective vernalization days. As such, adopting winter wheat varieties with lower vernalization requirements may be useful to deal with this constraint.
5 Conclusion
In summary, among a large range of yield projections, simulations with early flowering cultivars result in higher yield gains than that of early sowings, which successfully mitigate and even reverse the projected mean yield reductions. Therefore, development of early flowering cultivars from breeding program may help maintain and increase local grain yield productions in future climates for the major wheat production region of Portugal, along with likely effects for regions with similar Mediterranean-type climates. However, the extent of flowering earliness should only reach up to a point where shortened duration of vegetative growth does not constitute significant potential yield reduction. Despite some inherent uncertainties (e.g., climate projection uncertainties) and limitations (e.g., lack of inclusion of other crop models), our findings are expected to contribute to a better understanding of crop yield response to future climate changes under typical Mediterranean environments, as well as aiding in designing suitable adaptation strategies for policymakers, e.g., by providing insights for guiding breeding efforts.
References
Alexandratos N, Bruinsma J (2012) World agriculture towards 2030/2050: the 2012 revision. ESA working paper no. 12-03. FAO, Rome
Asseng S, Foster I, Turner NC (2011) The impact of temperature variability on wheat yields. Glob Chang Biol 17:997–1012. https://doi.org/10.1111/j.1365-2486.2010.02262.x
Asseng S et al (2013) Uncertainty in simulating wheat yields under climate change. Nat Clim Chang 3:827–832. https://doi.org/10.1038/Nclimate1916
Brigitta T, Melanie W, László P, Tomislav H (2017) 3D soil hydraulic database of Europe at 250 m resolution. Hydrol Process 31:2662–2666. https://doi.org/10.1002/hyp.11203
Brisson N et al (1998) STICS: a generic model for the simulation of crops and their water and nitrogen balances. I. Theory and parameterization applied to wheat and corn. Agronomie 18:311–346. https://doi.org/10.1051/agro:19980501
Brisson N et al (2002) STICS: a generic model for simulating crops and their water and nitrogen balances. II. Model validation for wheat and maize. Agronomie 22:69–92. https://doi.org/10.1051/agro:2001005
Brisson N et al (2003) An overview of the crop model stics. Eur J Agron 18:309–332. https://doi.org/10.1016/S1161-0301(02)00110-7
Brisson N, Launay M, Mary B, Beaudoin N (2009) Conceptual basis, formalisations and parameterization of the STICS crop model. Editions Quae, Versailles
Carvalho M, Basch G (1995) Optimisation of nitrogen fertilisation. Fert Res 43:127–130. https://doi.org/10.1007/bf00747691
Challinor AJ, Watson J, Lobell DB, Howden SM, Smith DR, Chhetri N (2014) A meta-analysis of crop yield under climate change and adaptation. Nat Clim Chang 4:287. https://doi.org/10.1038/nclimate2153
Costa R et al (2013) Effect of sowing date and seeding rate on bread wheat yield and test weight under Mediterranean conditions. Emir J Food Agr 25:951–961. https://doi.org/10.9755/ejfa.v25i12.16731
Coucheney E et al (2015) Accuracy, robustness and behavior of the STICS soil–crop model for plant, water and nitrogen outputs: evaluation over a wide range of agro-environmental conditions in France. Environ Model Softw 64:177–190. https://doi.org/10.1016/j.envsoft.2014.11.024
Dahlgren P, Landelius T, Kallberg P, Gollvik S (2016) A high-resolution regional reanalysis for Europe. Part 1: three-dimensional reanalysis with the regional High-Resolution Limited-Area Model (HIRLAM). Q J Roy Meteor Soc 142:2119–2131. https://doi.org/10.1002/qj.2807
Debaeke P (2004) Scenario analysis for cereal management in water-limited conditions by the means of a crop simulation model (STICS). Agronomie 24:315–326. https://doi.org/10.1051/agro:2004035
Dee DP et al (2011) The ERA-Interim reanalysis: configuration and performance of the data assimilation system. Q J Roy Meteor Soc 137:553–597. https://doi.org/10.1002/qj.828
Deser C, Knutti R, Solomon S, Phillips AS (2012) Communication of the role of natural variability in future North American climate. Nat Clim Chang 2:775. https://doi.org/10.1038/nclimate1562
Dias AS, Lidon FC (2009) Evaluation of grain filling rate and duration in bread and durum wheat, under heat stress after anthesis. J Agron Crop Sci 195:137–147. https://doi.org/10.1111/j.1439-037X.2008.00347.x
FAO/IIASA/ISRIC/ISSCAS/JRC (2012) Harmonized world soil database (version 1.2). FAO, Rome, Italy and IIASA, Laxenburg, Austria
Farooq M, Bramley H, Palta JA, KHM S (2011) Heat stress in wheat during reproductive and grain-filling phases. Crit Rev Plant Sci 30:491–507. https://doi.org/10.1080/07352689.2011.615687
Gouveia C, Trigo RM (2008) Influence of climate variability on wheat production in Portugal. In: Soares A, Pereira MJ, Dimitrakopoulos R (eds) geoENV VI—geostatistics for environmental applications: Proceedings of the Sixth European Conference on Geostatistics for Environmental Applications. Springer Netherlands, Dordrecht, pp 335–345. https://doi.org/10.1007/978-1-4020-6448-7_28
Hengl T et al (2017) SoilGrids250m: global gridded soil information based on machine learning. Plos One 12:e0169748. https://doi.org/10.1371/journal.pone.0169748
Howden SM, Soussana J-F, Tubiello FN, Chhetri N, Dunlop M, Meinke H (2007) Adapting agriculture to climate change. Proc Natl Acad Sci 104:19691–19696. https://doi.org/10.1073/pnas.0701890104
IPCC (2013) The physical science basis. Contribution of working group I to the fifth assessment report of the intergovernmental panel on climate change. In: Stocker TF, Qin D, Plattner G-K, Tignor M, Allen SK, Boschung J, Nauels A, Xia Y, Bex V, Midgley PM (eds) . Cambridge University Press, Cambridge
IPCC (2015) Workshop Report of the Intergovernmental Panel on Climate Change Workshop on Regional Climate Projections and their Use in Impacts and Risk Analysis Studies. In: Stocker TF, Qin D, Plattner G-K, Tignor M (eds) . IPCC Working Group I Technical Support Unit, University of Bern, Bern
Jacob D et al (2014) EURO-CORDEX: new high-resolution climate change projections for European impact research. Reg Environ Chang 14:563–578. https://doi.org/10.1007/s10113-013-0499-2
Jégo G, Pattey E, Bourgeois G, Morrison MJ, Drury CF, Tremblay N, Tremblay G (2010) Calibration and performance evaluation of soybean and spring wheat cultivars using the STICS crop model in Eastern Canada. Field Crops Res 117:183–196. https://doi.org/10.1016/j.fcr.2010.03.008
Jones N, de Graaff J, Rodrigo I, Duarte F (2011) Historical review of land use changes in Portugal (before and after EU integration in 1986) and their implications for land degradation and conservation, with a focus on Centro and Alentejo regions. Appl Geogr 31:1036–1048. https://doi.org/10.1016/j.apgeog.2011.01.024
Kassie BT, Asseng S, Rotter RP, Hengsdijk H, Ruane AC, Van Ittersum MK (2015) Exploring climate change impacts and adaptation options for maize production in the Central Rift Valley of Ethiopia using different climate change scenarios and crop models. Clim Chang 129:145–158. https://doi.org/10.1007/s10584-014-1322-x
Kimball BA (2016) Crop responses to elevated CO2 and interactions with H2O, N, and temperature. Curr Opin Plant Biol 31:36–43. https://doi.org/10.1016/j.pbi.2016.03.006
Klein Tank AMG et al (2002) Daily dataset of 20th-century surface air temperature and precipitation series for the European Climate Assessment. Int J Climatol 22:1441–1453. https://doi.org/10.1002/joc.773
Landelius T, Dahlgren P, Gollvik S, Jansson A, Olsson E (2016) A high-resolution regional reanalysis for Europe. Part 2: 2D analysis of surface temperature, precipitation and wind. Q J Roy Meteor Soc 142:2132–2142. https://doi.org/10.1002/qj.2813
Main crops production (t) by Geographic localization (Agrarian region) and Specie (2018) Instituto Nacional de Estatística (INE). https://www.ine.pt/xportal/xmain?xpid=INE&xpgid=ine_indicadores&indOcorrCod=0000021&xlang=en&contexto=bd&selTab=tab2. Accessed 13 July 2018
Osborne T, Rose G, Wheeler T (2013) Variation in the global-scale impacts of climate change on crop productivity due to climate model uncertainty and adaptation. Agr Forest Meteorol 170:183–194. https://doi.org/10.1016/j.agrformet.2012.07.006
Páscoa P, Gouveia CM, Russo A, Trigo RM (2017) The role of drought on wheat yield interannual variability in the Iberian Peninsula from 1929 to 2012. Int J Biometeorol 61:439–451. https://doi.org/10.1007/s00484-016-1224-x
Pfeifroth U, Sanchez-Lorenzo A, Manara V, Trentmann J, Hollmann R (2018) Trends and variability of surface solar radiation in Europe based on surface- and satellite-based data records. J Geophys Res Atmos 123:1735–1754. https://doi.org/10.1002/2017JD027418
Rolim J, Teixeira JL, Catalao J, Shahidian S (2017) The impacts of climate change on irrigated agriculture in Southern Portugal. Irrig Drain 66:3–18. https://doi.org/10.1002/ird.1996
Rosenzweig C, Tubiello FN (1996) Effects of changes in minimum and maximum temperature on wheat yields in the central US A simulation study. Agr Forest Meteorol 80:215–230. https://doi.org/10.1016/0168-1923(95)02299-6
Santos JA, Belo-Pereira M, Fraga H, Pinto JG (2016) Understanding climate change projections for precipitation over western Europe with a weather typing approach. J Geophys Res-Atmos 121:1170–1189. https://doi.org/10.1002/2015jd024399
Schmidhuber J, Tubiello FN (2007) Global food security under climate change. Proceed Natl Acad Sci 104:19703–19708. https://doi.org/10.1073/pnas.0701976104
Scotti-Campos P, Semedo JN, Pais I, Oliveira M, Passarinho J, Ramalho JC (2014) Heat tolerance of Portuguese old bread wheat varieties. Emir J Food Agr 26:170–179. https://doi.org/10.9755/ejfa.v26i2.16761
Valverde P, de Carvalho M, Serralheiro R, Maia R, Ramos V, Oliveira B (2015) Climate change impacts on rainfed agriculture in the Guadiana river basin (Portugal). Agric Water Manag 150:35–45. https://doi.org/10.1016/j.agwat.2014.11.008
van Vuuren DP et al (2011) The representative concentration pathways: an overview. Clim Chang 109:5–31. https://doi.org/10.1007/s10584-011-0148-z
Wang B, Liu DL, Asseng S, Macadam I, Yu Q (2015) Impact of climate change on wheat flowering time in eastern Australia. Agr Forest Meteorol 209-210:11–21. https://doi.org/10.1016/j.agrformet.2015.04.028
Wang B, Liu DL, Asseng S, Macadam I, Yu Q (2017) Modelling wheat yield change under CO2 increase, heat and water stress in relation to plant available water capacity in eastern Australia. Eur J Agron 90:152–161. https://doi.org/10.1016/j.eja.2017.08.005
Yang W, Andréasson J, Phil Graham L, Olsson J, Rosberg J, Wetterhall F (2010) Distribution-based scaling to improve usability of regional climate model projections for hydrological climate change impacts studies. Hydrol Res 41:211–229. https://doi.org/10.2166/nh.2010.004
Acknowledgments
The authors acknowledge the FCT scholarship given to the first author, PD/BD/113617/2015, under the Doctoral Programme “Agricultural Production Chains−from fork to farm” (PD/00122/2012). We also thank the FCT for CEECIND/00447/2017.
Funding
This work was supported by European Investment Funds by FEDER/COMPETE/POCI–Operational Competitiveness and Internationalization Programme, under Project POCI-01-0145-FEDER-006958, and National Funds by FCT–Portuguese Foundation for Science and Technology, UID/AGR/04033/2013.
Author information
Authors and Affiliations
Corresponding author
Ethics declarations
Conflict of interest
The authors declare that they have no conflict of interest.
Additional information
Publisher’s note
Springer Nature remains neutral with regard to jurisdictional claims in published maps and institutional affiliations.
Rights and permissions
Open Access This article is distributed under the terms of the Creative Commons Attribution 4.0 International License (http://creativecommons.org/licenses/by/4.0/), which permits unrestricted use, distribution, and reproduction in any medium, provided you give appropriate credit to the original author(s) and the source, provide a link to the Creative Commons license, and indicate if changes were made.
About this article
Cite this article
Yang, C., Fraga, H., van Ieperen, W. et al. Effects of climate change and adaptation options on winter wheat yield under rainfed Mediterranean conditions in southern Portugal. Climatic Change 154, 159–178 (2019). https://doi.org/10.1007/s10584-019-02419-4
Received:
Accepted:
Published:
Issue Date:
DOI: https://doi.org/10.1007/s10584-019-02419-4