Abstract
We present an assessment on the predictability of the rate of penetration using artificial neural networks when only drilling records from an adjacent are at hand. The study was carried out with data from two Enhanced Geothermal System wells in South Korea. We compare five data-arrangement cases for neural network training. Differences in the specific values of parameters from the adjacent and the predicted well, such as the weight on bit, and the rotary speed influenced the prediction errors. Among the five cases, the highest error (85.3%) occurred for the case of cumulative data up to the point of prediction. However, some data-arrangements decrease the error, e.g. when employing the whole data (46.8%), or when using small data sections near prediction (case 4: 42.4%, and case 5: 39.7%). We suggest the combination of these last tree approaches when prediction ROP with limited data.
Access this chapter
Tax calculation will be finalised at checkout
Purchases are for personal use only
Preview
Unable to display preview. Download preview PDF.
Similar content being viewed by others
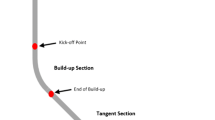
References
Bourgoyne, A. T. & Young, F. S. (1974). A multiple regression approach to optimal drilling and abnormal pressure detection. Soc. Pet. Eng. J. 14, pp 371-384.
Diaz, M., Kim, K. Y., Yeom, S., Zhuang, L., Park, S., & Min, K. B. (2017). Surface roughness characterization of open and closed rock joints in deep cores using X-ray computed tomography. Journal of Rock Mechanics and Mining Sciences, 98, pp 10-19.
Diaz, M. B., Kim, K. Y., Kang, T. H., & Shin, H. S. (2018). Drilling data from an enhanced geothermal project and its pre-processing for ROP forecasting improvement. Geothermics, 72, pp 348-357.
Diaz, M. B., Kim, K. Y., Shin, H. S., & Zhuang, L. (2019). Predicting rate of penetration during drilling of deep geothermal well in Korea using artificial neural networks and real-time data collection. Journal of Natural Gas Science and Engineering, 67, pp 225-232.
Hofmann, H., Zimmermann, G., Farkas, M., Huenges, E., Zang, A., Leonhardt, Kwiatek, G., Martinez-Garzon, P., Bohnhoff, M., Min, K.B. and Fokker, P. (2019). First field application of cyclic soft stimulation at the Pohang Enhanced Geothermal System site in Korea. Geophysical Journal International, 217(2), pp 926-949.
Lee, K. K., Ellsworth, W. L., Giardini, D., Townend, J., Ge, S., Shimamoto, T., Yeo, I.W., Kang, T.S., Rhie, J., Sheen, D.H. and Chang, C. (2019). Managing injection-induced seismic risks. Science, 364(6442), pp 730-732.
Lu, S. M. (2018). A global review of enhanced geothermal system (EGS). Renewable and Sustainable Energy Reviews, 81, pp 2902-2921.
Monazami, M., Hashemi, A., & Shahbazian, M. (2012). Drilling rate of penetration prediction using artificial neural network: a case study of one of Iranian southern oil fields. J. Oil Gas Bus, 6(6), pp 21-31.
Tester, J.W., Anderson, B. J., Batchelor, A.S., Blackwell, D.D., DiPippo, R. Drake, E.M., Garnish, J., Livesay, B., Moore, M.C., Nichols, K., Petty, S., Toksöz, M.N. (2006). The Future of Geothermal Energy: Impact of Enhanced Geothermal Systems (EGS) on the United States in the 21st Century. Massachusetts Institute of Technology, Cambridge, MA, USA.
Yuswandari, A., Prayoga, A., and Purba, D. (2019). Rate of Penetration (ROP) Prediction Using Artificial Neural Network to Predict ROP for Nearby Well in a Geothermal Field. In 44th Workshop on Geothermal Reservoir Engineering. Stanford University, California.
Zou, J., Han, Y., So, S. -S. (2008). Overview of Artificial Neural Networks, in: Humana Press, pp 14–22.
Author information
Authors and Affiliations
Corresponding author
Editor information
Editors and Affiliations
Rights and permissions
Copyright information
© 2020 Springer Nature Singapore Pte Ltd.
About this paper
Cite this paper
Diaz, M., Kim, K.Y., Lee, J., Shin, HS. (2020). Prediction of rate of penetration with data from adjacent well using artificial neural network. In: Duc Long, P., Dung, N. (eds) Geotechnics for Sustainable Infrastructure Development. Lecture Notes in Civil Engineering, vol 62. Springer, Singapore. https://doi.org/10.1007/978-981-15-2184-3_68
Download citation
DOI: https://doi.org/10.1007/978-981-15-2184-3_68
Published:
Publisher Name: Springer, Singapore
Print ISBN: 978-981-15-2183-6
Online ISBN: 978-981-15-2184-3
eBook Packages: EngineeringEngineering (R0)