Abstract
Stochastic distances combined with Minimum Distance method for region-based classification of Polarimetric Synthetic Aperture Radar (PolSAR) image was successfully verified in Silva et al. (2013). Methods like K-Nearest Neighbors may also adopt stochastic distances and then used in a similar purpose. The present study investigates the use of kernel methods for PolSAR region-based classification. For this purpose, the Jeffries-Matusita stochastic distance between Complex Multivariate Wishart distributions is integrated in a kernel function and then used in Support Vector Machine and Graph-Based kernel methods. A case study regarding PolSAR remote sensing image classification is carried to assess the above mentioned methods. The results show superiority of kernel methods in comparison to the other analyzed methods.
You have full access to this open access chapter, Download conference paper PDF
Similar content being viewed by others
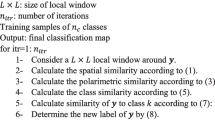
Keywords
1 Introduction
Polarimetric Synthetic Aperture Radar (PolSAR) are sensors able to record the amplitude, phase and orientation of electromagnetic waves backscattered from targets in earth surface. PolSAR image classification has been intensively investigated. Earlier studies about PolSAR image classification were developed with basis on the supervised Maximum Likelihood Classifier framework considering as probability density function the Complex Multivariate Wishart distribution [6] and on unsupervised classification process based on the eigenvalue analysis of coherency matrix [3].
The study and development of new methods for PolSAR data classification still being investigated. Region-based classification is useful for radar data, which are normally analyzed using pixel-based methods. The use of stochastic distances between Complex Multivariate Wishart distributions on the Minimum Distance Classifier for region-based classification was verified in [9] and better results were achieved in comparison to pioneer method proposed in [6]. Through the integration of stochastic distances between Multivariate Gaussian distributions in kernel functions, the Support Vector Machine (SVM) and Graph-Based (GB) methods were used for region-based classification of Synthetic Aperture Radar (SAR) in [7]. The K-Nearest Neighbors (KNN) method with stochastic distances was also reported in [7]. However, investigations involving other stochastic distances and kernel machines for PolSAR data still not made.
Face to the exposed, this study analyzes the use of stochastic distances on SVM and Graph-Based kernel methods, through kernel functions, for region-based classification of PolSAR image. Comparisons with the Minimum Distance Classifier framework investigated in [9] and KNN integrate with stochastic distances between Complex Multivariate Wishart distributions are presented. Such comparisons are conducted on a PolSAR image acquired from an actual PolSAR sensor with different classification scenarios.
2 Stochastic Distances for PolSAR Data
Supposing that targets on PolSAR images has homogeneous texture, the Complex Multivariate Wishart distribution can be used to model such targets:
where \(\mathbf {Z}\) is a n-looks covariance matrix computed from the average of n backscatter measurements in a neighborhood. Usually, the backscatter measurements are complex scattering vector \(\mathbf {z}^{T} = \left( S_{hh} \ S_{hv} \ S_{vv} \right) \) with components as complex number representing the amplitude and phase in a transmitting-receiving linear polarization combination. Regarding the parameters, n is the equivalent number of looks and \(\mathbf {\Sigma }\) represent the target mean covariance matrix computed through a set of independent samples. In addition, \(\varGamma _{3}(n) = \pi ^{3}\prod _{i=0}^{2}\varGamma (n - i)\), with \(\varGamma \left( \cdot \right) \) representing the gamma function.
In statistical analysis of PolSAR data stochastic distances appears as a powerful tool. These distances quantify the contrast between sets of information with basis on the dissimilarity between its probability distributions.
Let X and Y random variables following Complex Multivariate Wishart distributions with parameters \(\mathbf {\Sigma }_X\) and \(\mathbf {\Sigma }_Y\), respectively, both with equivalent number of looks equal to n. The Bathacharrya distance between X and Y is [5]:
Re-mapping the Bathacharrya measurements from \([0,\infty ]\) to [0, 2], Jeffries-Matusita arises as alternative distance:
3 Region-Based Classification and Kernel Methods
The region-based classification process consists of associating a class \(\omega _{j} \subset \varOmega , j=1,\ldots ,c\), to all pixels that compose a region \(\mathcal {R}_{i}\). The regions \(\mathcal {R}_{i}, i = 1, \ldots , r\), are subsets of pixels that partition the support \(\mathcal {S} \subset \mathbb {N}^2\) of a given image \(\mathcal {I}\). For supervised region-based classification, the decision rule is built using information from \(\mathcal {D} = \left\{ \left( \mathcal {R}_{i},\omega _{j} \right) \in \mathcal {S} \times \varOmega : i = 1, \ldots , m; j = 1, \ldots , c \right\} \), a set of labelled training regions. The notation \(\left( \mathcal {R}_{i},\omega _{j} \right) \) indicates that \(\mathcal {R}_{i}\) is assigned to \(\omega _{j}\).
A simple way to perform region-based classification is adopt the Minimum Distance Classifier framework using stochastic distances as a measure to compare the similarity between classes and unlabeled regions [9]. We refer to this method as Minimum Stochastic Distance Classifier (MSDC). Formally, let \(\mathcal {R}_{i}\) be an unlabeled region and let \(\text {D}(f_{\mathcal {R}_{i}},f_{\omega _{j}})\) be a stochastic distance between the distributions of the pixels in \(\mathcal {R}_{i}\) and the class \(\omega _{j}\), represented by \(f_{\omega _{j}}\) and modeled through the pixels of labeled regions assigned to \(\omega _{j}\) in \(\mathcal {D}\), an assignment \((\mathcal {R}_{i},\omega _{j})\) is made when the following rule is satisfied:
K-Nearest Neighbors (KNN) is another method successfully adopted for region-based classification using stochastic distances [7]. For this purpose, \(D(\cdot ,\cdot )\) in (4) is substituted by \(D_{\text {knn}}(\cdot ,\cdot )\) defined as:
where \(h_{j}(f_{\mathcal {R}_{i}}) = \# \left\{ \left( \bar{\mathcal {R}},\omega _{j}\right) \in V_{k}(\mathcal {R}_{i}) \right\} \), such that \(V_{k}(\mathcal {R}_{i})\) is the set of k training regions close to \(\mathcal {R}_{i}\) given a distance \(D(\cdot ,\cdot )\). Formally,
where \(\bar{\mathcal {R}}_{p}\) represents a new indexing of the k nearest regions of \(\mathcal {D}\) based on the proximity to \(\mathcal {R}_{i}\).
Besides MSDC and KNN, among several methods that may be adopted to perform region-based classification, kernel-based methods are an option. SVM [10] and GB [2] are examples of kernel-based methods. Such methods allows the use of kernel functions, \(K:\mathcal {X}^{2} \rightarrow \mathbb {R}\), which are usually adopted to improve classification performance on non-linearly separable data in the original attribute space \(\mathcal {X}\) as to generalize the application in problems where the input data are non-vectorial. In special, for region-based approach where the input data are regions, the use of an adequately kernel function is a convenient alternative.
A given \(K:\mathcal {X}^{2} \rightarrow \mathbb {R}\) is a kernel function if it is symmetric and conforms to Mercer conditions. A straight way to define a valid kernel is considering a general model, like the radial basis model [8]:
where \(g:\mathbb {R} \rightarrow \mathbb {R}\) is a strictly positive real function and \(d:\mathcal {X}^{2} \rightarrow \mathbb {R}\) is a metric.
From Eq. (7) it is possible to develop kernel functions for PolSAR region-based image classification. A reasonable choice for \(g(\cdot )\) is the negative exponential function. With respect to \(d(\cdot ,\cdot )\), which measures the similarity between the input data, adopt a metric based on stochastic distances is convenient. Straightly considering \(d(\cdot ,\cdot )\) as Bathacharrya (Eq. (2)) or Jeffries-Matusita (Eq. (3)) stochastic distances will not produces valid kernel functions since such distances does not attains the triangle inequality. However, admitting \(\tau \in \mathbb {R}_{+}\) such that \(d(\mathbf {x},\mathbf {y}) \le \tau \) for all \(\mathbf {x},\mathbf {y} \in \mathcal {X}\), the following expression provides a metric \(\overline{d}(\cdot , \cdot )\) from a distance \(d(\cdot , \cdot )\):
Once the values of \({\text {D}}_\text {JM}\) are limited to [0, 2], a metric \(\overline{{\text {D}}_\text {JM}}\) is defined substituting \(d(\cdot ,\cdot )\) by \(\text {D}_\text {JM}(\cdot ,\cdot )\) and adopting \(\tau = 2\) in Eq. (8). As result, the following kernel function is defined:
where \(\gamma \in \mathbb {R}_{+}\) is a regularization parameter.
4 Experiments and Results
In order to assess the performance of SVM and GB methods adopting the kernel function defined in Eq. (9) on region-based classification of PolSAR data, a case study regarding a multi-class remote sensing image classification under three distinct scenario was carried. The MSDC and KNN methods using the Jeffries-Matusita distance, as presented in Eqs. (4) and (5), were included in the analysis for comparison.
The PolSAR data adopted in this study corresponds to an image acquired on March \(13^{th}\), 2009, by the ALOS-PALSAR sensor in a region near the Tapajos National Forest, State of Pará, Brazil. This image has approximately \(20\,\text {m}\) resolution after a \(3 \times 3\) multi-look process. The following land use and land cover (LULC) types were considered: Primary Forest (PF), Regeneration (RE), Pasture (PS), Bare Soil (BS) and three types of Agriculture (A1, A2 and A3). Figure 1(a) present a color composition of the ALOS-PALSAR image. The spatial distribution of LULC samples is depicted in Fig. 1(b), where training and test samples correspond to solid and void polygons, respectively. Table 1 presents a summary about the LULC samples. The region-growing method, available in the Geographic Information System SPRING [1], was used in order to segment the image and define the regions. The segmentation parameters were chosen by visual inspection. Figure 1(c) represents the contours of the regions on the segmented study image.
As above mentioned, three distinct classification scenarios were considered. The first scenario is composed by all LULC classes identified in the study area. From the union of the agriculture classes (i.e., A1, A2 and A3) it is defined the new class called Agricultural Areas (AA), and then a second scenario it is created with the five classes AA, PF, PS, RE and BS. The last scenario has the Agricultural Areas, High Biomass (HB) and Low Biomass (LB) classes. While HB is obtained merging PF and RE classes, LB comes from the union between PS and BS. Such scenarios represent plausible situations, once classes with similar semantic are merged from scenario 1 to define the classes of scenarios 2 and 3. Additionally, worth observe that each scenario has a specific complexity in terms of number of classes and inter/intra-class contrasts. Consequently, its use for comparison purposes allows more robust analysis of the investigated methods. It is valid mention that training and testing samples of AA, HB and LB classes arise by simple merging the sample polygons of the individual classes.
The selections of adequate number of neighbors (k – KNN), penalty (C – SVM), neighbors influence (\(\alpha \) – GB) and kernel regularization (\(\gamma \) – Eq. (9)) parameters were based on a grid search process with tenfold cross-validation. The space search for each parameter was: \(k \in \{3,5,7,9\}, C \in \{ 1, 10, 100, 1000, 10000 \}, \alpha \in \{0.1, 0.2, \ldots , 1.0 \}\) and \(\gamma \in \{ 0.25, 0.5, \ldots , 3.0 \}\). The one-against-all multi-class strategy was adopted by SVM.
Concerning the PolSAR image and LULC classes in different scenarios, a total of 12 classification results were obtained. The accuracy of results were calculated by means of kappa agreement coefficient, regarding the LULC testing samples, and hypothesis test with \(5\%\) significance level were performed in order to compare the kappa values [4]. The analyzed methods were also compared in terms of computational time. The experiments were performed using a computer with an Intel Core i7 processor and 16 GB of RAM running the Ubuntu Linux version 14.4 operating system. The implementations were conducted using the IDL (Interactive Data Language) programming language. The performance of analyzed methods are shown in Fig. 2.
As initial discussion, we can observe that low kappa values were assigned to MSDC. Furthermore, the accuracy of MSDC decreases as the number of classes also decreases along the scenarios. This behavior is assigned to the scenario complexity, which increase when some LULC classes are merged giving place to new classes with higher variability.
Regarding the first scenario, the most accurate results was achieved by KNN method. Although GB presented a lower kappa coefficient in comparison to KNN, its accuracy values are statistically equivalent.
The accuracy levels presented by SVM and GB were higher in scenario 2 compared to other scenarios, where SVM was more accurate. It is worth note that KNN was superior to MSDC.
We also can observe that the variability of the classes in scenario 3 plays less effect on SVM and GB than MSDC and KNN. Furthermore, SVM and GB are statistically equivalent in this scenario.
Face to its simple algorithmic implementation, MSDC is the less expensive method in terms of computational time. SVM presents the higher computational time, which tends to increase as the amount of classes increase in reason of its multi-class classification architecture. An intermediate cost between MSDC and SVM is presented by GB method. As consequence of multiple comparisons needed to build the decision rule to classify each unlabeled region, KNN presented computational time over 5100 s.
Figure 3 depicts the classification results for each method and scenario. It can be note that, independently of method, RE and PS classes were not well discriminated in scenarios 1 and 2. In the first scenario, while MSDC and KNN were not able to discriminate BS areas, SVM and GB fails to identify A2 areas. GB tends to classify A1 as BS and SVM frequently classifies PS as RE areas. Focusing the second scenario, MSDC was imprecise classifying AA areas as SVM the BS class and KNN pasture areas. The last scenario reveals MSDC and KNN as unable to distinguish LB and AA classes.
5 Conclusions
This study verified the performance SVM and GB on region-based classification of PolSAR image in comparison to MSDC and KNN. The Jeffries-Matusita stochastic distances between Complex Multivariate Wishart distributions was integrated in a kernel function and then adopted by SVM and GB. A case study about LULC classification using ALOS-PALSAR image was addressed.
Both SVM and GB achieved higher accuracy levels, especially on scenarios with higher intra-class variability. Regarding the first scenario, where the contrast between classes is higher, KNN provided more accurate results. Lower accuracy values are assigned to MSDC in comparison to the analyzed methods.
The better tradeoff between classification accuracy and computational cost is offered by GB. Consequently, the GB method becomes a potential alternative for region-based classification of PolSAR data. Although KNN allowed accurate results the computational time its main drawback.
References
Camara, G., Souza, R.C.M., Ii, F.M., Freitas, U., Garrido, J.: Spring: integrating remote sensing and GIS by object-oriented data modelling. Comput. Graph. 20, 3 (1996)
Camps-Valls, G., Tatyana, V.B., Zhou, D.: Semi-supervised graph-based hyperspectral image classification. IEEE Trans. Geosci. Remote Sens. 45, 2044–3054 (2007)
Cloude, S.R., Pottier, E.: An entropy based classification scheme for land applications of polarimetric SAR. IEEE Trans. Geosci. Remote Sens. 35(1), 68–78 (1997)
Congalton, R.G., Green, K.: Assessing the Accuracy of Remotely Sensed Data. CRC Press, Boca Raton (2009)
Frery, A.C., Nascimento, A.D.C., Cintra, R.J.: Analytic expressions for stochastic distances between relaxed complex Wishart distributions. IEEE Trans. Geosci. Remote Sens. 52(2), 1213–1226 (2014)
Lee, J., Grunes, M., Kwok, R.: Classification of multi-look polarimetric SAR imagery based on complex Wishart distribution. Int. J. Remote Sens. 15(11), 2299–2311 (1994)
Negri, R.G., Dutra, L.V., Sant’Anna, S.J.S., Lu, D.: Examining region-based methods for land cover classification using stochastic distances. Int. J. Remote Sens. 37(8), 1902–1921 (2016). https://doi.org/10.1080/01431161.2016.1165883
Schölkopf, B., Smola, A.J.: Learning with Kernels: Support Vector Machines, Regularization, Optimization, and Beyond. Adaptive Computation and Machine Learning. MIT Press, Cambridge (2002)
Silva, W.B., Freitas, C.C., Sant’Anna, S.J.S., Frery, A.C.: Classification of segments in PolSAR imagery by minimum stochastic distances between Wishart distributions. IEEE J. Sel. Top. Appl. Earth Obs. Remote Sens. 6(3), 1263–1273 (2013)
Vapnik, V.N.: The Nature of Statistical Learning Theory. Springer-Verlag New York Inc., New York (1995)
Acknowledgments
The authors thank FAPESP (Proc.: 2014/14830-8) for funding this research.
Author information
Authors and Affiliations
Corresponding author
Editor information
Editors and Affiliations
Rights and permissions
Copyright information
© 2018 Springer International Publishing AG, part of Springer Nature
About this paper
Cite this paper
Negri, R.G., Casaca, W.C.O., Silva, E.A. (2018). Region-Based Classification of PolSAR Data Through Kernel Methods and Stochastic Distances. In: Mendoza, M., Velastín, S. (eds) Progress in Pattern Recognition, Image Analysis, Computer Vision, and Applications. CIARP 2017. Lecture Notes in Computer Science(), vol 10657. Springer, Cham. https://doi.org/10.1007/978-3-319-75193-1_52
Download citation
DOI: https://doi.org/10.1007/978-3-319-75193-1_52
Published:
Publisher Name: Springer, Cham
Print ISBN: 978-3-319-75192-4
Online ISBN: 978-3-319-75193-1
eBook Packages: Computer ScienceComputer Science (R0)