Abstract
In this paper a method for feature selection and classification of email spam messages is presented. The selection of features is performed in two steps: The selection is performed by measuring their entropy and a fine-tuning selection is implemented using a genetic algorithm. In the classification process, a Radial Basis Function Network is used to ensure robust classification rate even in case of complex cluster structure. The proposed method shows that, when using a two-level feature selection, a better accuracy is achieved than using one-stage selection. Also, the use of a lemmatizer or a stop-word list gives minimal classification improvement. The proposed method achieves 96-97% average accuracy when using only 20 features out of 15000.
Access this chapter
Tax calculation will be finalised at checkout
Purchases are for personal use only
Preview
Unable to display preview. Download preview PDF.
Similar content being viewed by others
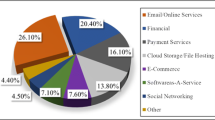
References
Sakkis, G., Androutsopoulos, I., Paliouras, G., Karkaletsis, V., Spyropoulos, C.D., Stamatopoulos, P.: A memory-based approach to anti-spam filtering for mailing lists. Information Retrieval 6, 49–73 (2003)
Androutsopoulos, I., Koutsias, J., Chandrinos, K.V., Paliouras, G., Spyropoulos, C.D.: An Evaluation of Naive Bayesian Anti-Spam Filtering. In: Proc. of the workshop on Machine Learning in the New Information Age (2000)
Lee, D.L., Chuang, H., Seamons, K.: Document Ranking and the Vector-Space Model. IEEE Software 14, 67–75 (1997)
Steinbach, M., Karypis, G., Kumar, V.: A Comparison of Document Clustering Techniques. In: KDD Workshop on Text Mining (2000)
Michelakis, E., Androutsopoulos, I., Paliouras, G., Sakkis, G., Stamatopoulos, P.: Filtron: A Learning-Based Anti-Spam Filter. In: Proc. of the 1st Conference on Email and Anti-Spam (2004)
Gavrilis, D., Tsoulos, I., Dermatas, E.: Stochastic Classification of Scientific Abstracts. In: Proceedings of the 6th Speech and Computer Conference, Patra (2005)
Pierre, J.M.: On the Automated Classification of Web Sites. Linkoping Electronic Articles in Computer and Information Science 6 (2001)
Deerwester, S., Dumais, S., Furnas, G., Landauer, T., Harshman, R.: Indexing by latent semantic analysis. Journal of the American Society for Information Science 46, 391–407 (1990)
Author information
Authors and Affiliations
Editor information
Editors and Affiliations
Rights and permissions
Copyright information
© 2006 Springer-Verlag Berlin Heidelberg
About this paper
Cite this paper
Gavrilis, D., Tsoulos, I.G., Dermatas, E. (2006). Neural Recognition and Genetic Features Selection for Robust Detection of E-Mail Spam. In: Antoniou, G., Potamias, G., Spyropoulos, C., Plexousakis, D. (eds) Advances in Artificial Intelligence. SETN 2006. Lecture Notes in Computer Science(), vol 3955. Springer, Berlin, Heidelberg. https://doi.org/10.1007/11752912_54
Download citation
DOI: https://doi.org/10.1007/11752912_54
Publisher Name: Springer, Berlin, Heidelberg
Print ISBN: 978-3-540-34117-8
Online ISBN: 978-3-540-34118-5
eBook Packages: Computer ScienceComputer Science (R0)