Abstract
Calibration is the process by which the execution of actions becomes scaled to the (changing) relationship between environmental features and the actor’s action capabilities. Though much research has investigated how individuals calibrate to perturbed optic flow, it remains unclear how different experimental factors contribute to the magnitude of calibration transfer. In the present study, we assessed how testing environment (Experiment 1), an adapted pretest-calibration-posttest design (Experiment 2), and bilateral ankle loading (Experiment 3) affected the magnitude of calibration to perturbed optic flow. We found that calibration transferred analogously to real-world and virtual environments. Although the magnitude of calibration transfer found here was greater than that reported by previous researchers, it was evident that calibration occurred rapidly and quickly plateaued, further supporting the claim that calibration is often incomplete despite continued calibration trials. We also saw an asymmetry in calibration magnitude, which may be due to a lack of appropriate perceptual-motor scaling prior to calibration. The implications of these findings for the assessment of distance perception and calibration in real-world and virtual environments are discussed.
Similar content being viewed by others
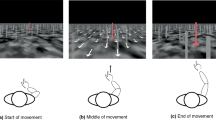
Successful interaction with an environment requires that self-motion be perceived veridically. In particular, we must be able to accurately perceive the rate at which we are moving within the environment, as this enables the selection of appropriate paths of travel, the avoidance of collisions, and so on. Self-motion perception largely depends on “lawful relationships between the structure of light and the movements of an observer through a stationary environment” (Warren, 1988, p. 344), or more simply, optic flow. When moving through an environment, we sample and reveal transformations in the global optic array, and this lawfully generated information allows us to regulate forces of movement (Warren, 1990) and perform stable patterns of action (Bertenthal, Rose, & Bai, 1997). As such, self-motion perception is crucial for path integration (i.e., gauging traversed distances). Individuals are able to perceive traversed distances via multiple perceptual modalities (Harrison, 2020). Thus, when information from one modality becomes unavailable, (e.g., when blindfolded), individuals can leverage other available information to perceive self-motion and gauge traversed distances. For instance, when asked to estimate egocentric distances via a blind walking task, individuals have been shown to judge distances with a high degree of accuracy (Loomis, da Silva, Fujita, & Fukusima, 1992; Loomis & Knapp, 2003; Sahm, Creem-Regehr, Thompson, & Willemsen, 2005).
Calibration to perturbed optic flow
What happens then when the information available in an organism’s environment is perturbed? When task demands change, such as when constraints arise and/or when perturbations are introduced into the organism-environment system, organisms must calibrate to continue to regulate their behavior (Redding & Wallace, 2003; Warren, 1990). For instance, if the organism is provided an opportunity to interact with the environment, the coupling between the perceptual and motor systems can then be recalibrated such that affordances are accurately perceived (e.g., Mark, 1987; for recent reviews, see Brand & de Oliveira, 2017; van Andel, Cole, & Pepping, 2017).
With respect to walking, an important source of information for calibration is optic flow, which has been studied in the context of both perceived direction and distance. Both have similarly shown that calibration is task specific. For example, “optic flow recalibrates a task-specific mapping from the visual direction of the target to the direction of locomotion” (Bruggeman & Warren, 2010, p. 1007). This task-specific, or functionally specific, nature of calibration has frequently been illustrated by inducing visual perturbations into the organism-environment system. For example, Martin, Keating, Goodkin, Bastian, and Thach (1996) asked participants to wear prism adaptation goggles while completing a right-handed throwing task and an overhand throwing task. They found that individuals calibrated to the prism goggles, demonstrated by throw impact points displaced in the opposite direction of the gaze shift, upon removal of the goggles. However, calibration did not transfer from the right hand to the left hand nor from overhand to underhand throwing, supporting task-specific calibration. Using a similar paradigm applied to walking distance, Rieser, Pick, Ashmead, and Garing (1995) decoupled optic flow rate from walking velocity by asking participants to walk at a set speed on a treadmill that was towed by a tractor travelling at a different speed. An important finding from this study was that participants exhibited calibration to the perturbed optic flow. That is, participants in the biomechanically faster (visually slower) condition overshot walking distances in the posttest, and participants in the biomechanically slower (visually faster) condition undershot distances in the posttest. In support of the task-specific nature of calibration, calibration to perturbed optic flow during walking did not transfer to a throwing task. Calibration of one action typically does not transfer to a different action (Bruggeman & Warren, 2010; Proffitt, 2008; Rieser et al., 1995; Witt, 2011; Witt, Proffitt, & Epstein, 2010). This underscores that what is being calibrated is not some internally represented perceived depth, as this would affect all actions utilizing that perceived depth (Pagano & Isenhower, 2008), but a task-specific perception-action system. What is calibrated is the mapping between units of perception and the units of a specific action (Bingham & Pagano, 1998; Bingham, Pan, & Mon-Williams, 2014; Day et al., 2019; Pan, Coats, & Bingham, 2014).
Since Rieser et al.’s (1995) initial study, researchers have employed similar methodologies to investigate calibration to perturbed optic flow using virtual reality (VR; e.g., Durgin et al., 2005; Kunz, Creem-Regehr, & Thompson, 2009, 2013, 2015; Mohler et al., 2004; Mohler et al., 2007; Ziemer et al., 2013). Despite the usefulness of VR as a tool for inducing these perturbations, there has been much debate over the issue of depth compression. Compared with the real world, individuals have been shown to underestimate egocentric distances in virtual environments (Geuss, Stefanucci, Creem-Regehr, & Thompson, 2012; Loomis & Knapp, 2003; Sahm et al., 2005). Furthermore, Ziemer et al. (2013) found that calibration transfer of perturbed optic flow differed depending on whether imagined walking assessment occurred in a real-world or virtual environment. Specifically, they found that participants underestimated distances more in a virtual environment than in a real-world environment following exposure to a visually faster optic flow. Following exposure to visually slower optic flow, they found that participants overestimated distances more in a virtual environment than in the real world.
In a recent study, however, Siegel and Kelly (2017) showed that allowing individuals the opportunity to interact with virtual environments effectively diminishes depth compression. Furthermore, Kelly, Cherep, and Siegel (2017) found that blind walking distance estimates in VR using an HTC Vive head-mounted display were comparable to distances estimates in the real world, which was not the case for older VR equipment. Given this, it is possible that depth compression in VR is less of a concern for newer VR equipment, such as the HTC Vive. To appropriately identify whether depth compression was an influential factor in calibration magnitude and transfer, the present study evaluated distance perception and calibration in both real-world and virtual testing environments.
Effects of perturbed limb weight on distance perception
In addition to inducing visual perturbations, such as perturbed optic flow, researchers have also induced physical perturbations, such as ankle loading. In one study, Walter, Peterson, Li, Wagman, and Stoffregen (2019) investigated how the addition of weights impacted affordances for walking on land and at sea. They found that participants’ walking ability along a narrow path was reduced when wearing ankle weights. Specifically, participants on both land and at sea judged that they could walk farther when they were not wearing ankle weights. When encumbered with ankle weights, participants walked slower on both land and at sea. Further, Paquet, Taillon-Hobson, and Lajoie (2015) investigated the effects of ankle loading on distance perception. In this study, participants estimated distances via blind walking while wearing no ankle weights, a single ankle weight, or two ankle weights. The results from this study showed that participants overestimated distances when their ankles were loaded with a 2.73 kg weight unilaterally and bilaterally compared with when their ankles were not loaded. Ankle weights increase energy expenditure, oxygen consumption, and net metabolic rate (Browning, Modica, Kram, & Goswami, 2007; Graves, Martin, Miltenberger, & Pollock, 1988; Skinner & Barrack, 1990), which indicates that the increase in distance walked found by Paquet et al. (2015) could be the result of an increased perception of metabolic cost required for task completion (see Proffitt, Stefanucci, Banton, & Epstein, 2003).
Additionally, the overshooting of distances found by Paquet et al. (2015) may have been a result of a recalibration of perceived forward displacement. That is, individuals may have kinesthetically scaled their forward displacement to be shorter than it was (Paquet et al., 2015), ultimately causing them to walk farther. Shibata, Gyoba, and Takeshima (2012) showed that participants underestimated end limb positions during reaching when the arm/hand was weighted and in motion. These results suggest that walking with ankle weights may impact individuals’ scaling of their leg length, as this involves swinging of the weighted limb. Importantly, participants in both studies were purposefully prevented from receiving feedback about their distance estimates (Paquet et al., 2015) and their limb length estimates (Shibata et al., 2012). That is, they were not informed on the accuracy of their estimates. Were they to receive feedback on the accuracy of their estimates, however, participants may have properly calibrated to the perturbations and exhibited more accurate estimates.
Present study
Previous research has shown that the extent to which individuals recalibrate to perturbed optic flow does not match the rate of optic flow experienced during the adaptation phase. In fact, calibration effects have varied greatly across studies, ranging from 3% to 22% in the magnitude of overshoot and undershoot relative to baseline distance estimates (Adams et al., 2018; Durgin et al., 2005; Kunz et al., 2013, 2015; Mohler et al., 2007). The aim of the present study, therefore, was to determine how the testing environment (real versus virtual), the experimental design (the scheduling of calibration trials), and the presence of ankle loading influence the magnitude of calibration to perturbed optic flow. First, we were interested in differences between real-world and virtual environments for evaluating calibration transfer. That is, identifying whether calibration effects were stronger in a real-world environment or a virtual environment. Much of the previous research in this domain has involved an adaptation phase in a virtual environment and testing phases in a real-world environment (e.g., Adams et al., 2018; Durgin et al., 2005; Mohler et al., 2007). However, there is little evidence on whether testing environment influences calibration to perturbed optic flow. In one study, Ziemer et al. (2013) investigated calibration transfer of perturbed optic flow to real-world and virtual environments. The results of this study showed that the magnitude of calibration depended on whether the distance estimation task, imagined walking, occurred in a real-world or virtual environment. They found that the magnitude of calibration was greater for both visually slower and visually faster conditions in the virtual environment compared with the real-world environment. Importantly, Ziemer et al. (2013) only compared imagined walking estimates between the real-world and virtual environments, and the use of a large screen display and treadmill limited the participants’ abilities to fully explore the virtual environment. Therefore, in Experiment 1, we evaluated calibration transfer to both real-world and virtual environments using immersive virtual reality technology in a large room. This allowed participants in both testing environments to walk freely at their own paces for the entirety of the experiment.
Second, we were interested in how experimental design impacts the magnitude of calibration. For the most part, studies in this domain have utilized a pretest-calibration-posttest design, in which participants estimate distances before and after a period of exposure to perturbed optic flow (i.e., a calibration or adaptation phase). In an attempt to increase the magnitude of calibration, researchers have increased the amount of exposure to perturbed optic flow (Durgin et al., 2005). This has had little success on increasing calibration effects. In an effort to successfully increase calibration effects, Experiment 2 incorporated an adapted pretest-calibration-posttest design to determine whether experimental design impacts the magnitude of calibration.
Lastly, we were interested in how ankle loading impacts the magnitude of calibration. Previous research has shown that adding weight to participants increases energy expenditure for task completion (Browning et al., 2007) and influences walking ability (Walter et al., 2019). Recent findings indicate that ankle loading results in an increase in distance estimates (Paquet et al., 2015). Thus, in an effort to increase the magnitude of calibration, Experiment 3 involved pairing bilateral ankle loading and slower optic flow to determine whether this would produce a stronger calibration effect.
Experiment 1
The purpose of Experiment 1 was to evaluate calibration of perturbed optic flow in two different testing environments. Participants estimated distances in either a real-world environment or a virtual environment in a pretest-calibration-posttest design. In each testing environment, participants experienced one of three perturbed optic flow conditions (visually slower, visually faster, or visually matched) during the calibration phase. We expected participants to underestimate distances in the posttest after exposure to visually faster optic flow and overestimate distances in the posttest after exposure to visually slower optic flow rate. We also expected these findings to be moderated by environment, such that there would be differences in the magnitude of underestimation and overestimation, depending on the testing environment (real world or virtual).
Method
Power analysis
To determine the Level 2 sample size (i.e., number of participants), a power analysis using an effect size of .4 (Cohen, Cohen, West, & Aiken, 2003) and an alpha of .05 revealed that a sample size of 60 participants would produce power of .80. To determine the Level 1 sample size (i.e., number of trials), the nested-ness of the data was considered. Data for each trial was nested within participants, such that some within-subject variance was accounted for by between-subject variables. Here, the number of trials does not accurately represent the number of independent observations. Rather, the intraclass correlation (ICC) is an index of nesting that can be used to identify the number of trials needed to represent the effective sample size of independent observations (Bickel, 2007; Snijders & Bosker, 2011). With an ICC ranging from .25 to .35, we identified that 45 trials per participant would produce an effective total sample size ranging from 225 to 164 participants. Using an effect size of .3 and an alpha of .05, the power analysis revealed that both effective sample sizes would produce power levels above .99, which is sufficient power to detect cross-level interactions (Van Der Leeden & Busing, 1994).
Participants
Sixty undergraduate students (44 females, age M = 19.8 years, SD = 5.1 years) from Clemson University participated in this study for partial fulfillment of course requirements after providing informed consent. All participants had normal or corrected-to-normal vision. Individuals with a history of epilepsy, seizures, neurological problems, or motor impairments were prohibited from participating in the study. The study was performed with approval of the Institutional Review Board of Clemson University.
Apparatus
An HTC Vive Pro System (HTC, Taiwan) was used to track participants’ movements. Four Vive base stations were positioned approximately 7 feet above the floor to track HTC Vive trackers, with one base station located in each corner of the room. Head movements were tracked using a Vive head mounted display (HMD) at a sampling rate of 90 Hz. The Vive HMD display was binocular with a 110-degree horizontal field of view. The HMD’s interpupillary distance (IPD) setting was adjusted to match each participant’s IPD. Two HTC Vive trackers were affixed to the left and right shoulders of an empty hiking backpack. The backpack was fit to each participant so that each tracker was positioned upright, relative to the participant’s shoulders (i.e., the trackers faced the ceiling). Positional data along the X, Y, and Z axes were obtained using a Unity application at a sampling rate of 90 Hz. These positional data were used to obtain participants’ distance judgments. Prior to analysis, the data were filtered for noise using a 4th order Butterworth filter at 90 samples with a cutoff frequency of 6 Hz.
The dimensions of the virtual scene for the virtual testing environment were created to match those of the real-world testing environment (see Fig. 1). We verified that the virtual testing environment’s dimensions and visual angle subtended matched the real-world testing environment through a two-step process outlined in Bhargava et al. (2020). The virtual scene for the calibration phases consisted of a 12 × 2.5-meter hallway with cinderblock walls. The purpose of using a hallway lined with cinderblocks for the calibration environment was to increase the texture information available and to increase the optic flow experienced by participants. All virtual scenes and objects were created using Unity.
Design and procedure
This experiment utilized a pretest-calibration-posttest design to assess the effects of perturbed optic flow on blind walking estimates in real-world and virtual environments. Participants were randomly assigned to a real-world (n = 30) or virtual testing environment (n = 30) where they completed the pretest and posttest trials. The calibration trials always occurred in the virtual environment. Participants were also randomly assigned to an optic flow condition, such that 10 participants in each testing environment experienced each optic flow condition.
Real-world environment procedure
Participants completed the experiment in three phases. In the pretest phase, participants completed 15 blind walking trials to targets randomly placed 2, 3, and 4 meters away. Participants viewed the target, covered their eyes with a blindfold, and then walked to where they believe the cone was located. After making each distance estimate, participants were guided back to the initial starting point by an experimenter. No feedback was provided to the participants on the accuracy of their distance estimates. Upon completion of the pretest phase, participants completed the calibration phase. They were outfitted with an HMD and asked to walk to targets randomly placed 2.5, 3.5, and 4.5 meters away. These target distances purposefully differed from the pretest and posttest target distances to discourage participants from memorizing the target distances. During this phase of the experiment, participants experienced one of three optic flow conditions: visually slower (translational gain of ×0.5), visually faster (translational gain of ×2), or visually matched (translational gain of ×1). After this phase, participants removed the HMD and began the posttest phase, which was identical to the pretest phase.
Virtual environment procedure
The procedure was the same as that for the real-world condition, except that the pretest and posttest phases occurred in a virtual environment matching that of the real-world condition, which was viewed via the HMD. During the pretest and posttest, participants’ views were occluded with a virtual gray screen instead of a blindfold.
Results
Analysis preparation
Prior to conducting analyses, we assessed the extent to which the data were nested by obtaining the intraclass correlation coefficient (ICC) from the null model. We found an ICC of 0.50, indicating that 50% of the variance in distance judgments was at the group level. This nesting of the data produces multiple levels of variance. Within-subjects variables (Level 1 variables) produce residual variance, between-subjects variables (Level 2 variables) produce intercept variance, and cross level interactions (Level 1 by Level 2 interactions) produce slope variance. To account for these multiple levels of variance, multilevel mixed effects modeling was used (for more information, see Woltman, Feldstain, MacKay, & Rocchi, 2012). To identify outliers in the data set, the residuals from the full model were saved and standardized. Observations with standardized score values falling outside of ±3 were removed from the data set. This outlier analysis resulted in less than 1% of the data being removed.
Multilevel mixed effects modeling
We utilized a conservative model approach in an effort to mitigate the likelihood of spurious results. First, a main effects model was run that included only the main effects (Phase, Environment, and Condition). The results reported below for each of these main effects are derived from this initial model. Analysis of two-way interactions was done by adding each interaction term to the main effects model separately. That is, a separate model was run to obtain the results for each two-way interaction term. To assess the three-way interaction, a model with all main effects, relevant two-way interaction terms, and the three-way interaction was run. All models also included a random effect of participant ID.
Effect size calculation
Effect sizes for Level 1 variables were calculated by comparing the residual variance of the model including the fixed effect of interest with the same model without the fixed effect. Similarly, effects sizes for Level 2 variables were calculated by comparing the intercept variance of the model including the fixed effect of interest with the same model without the fixed effect. This comparison yielded sr2 values, which refer to the unique contribution by each fixed effect. Effect sizes for cross-level interactions were calculated by comparing the slope variance of the model including the interaction of interest with the same model without the interaction. To obtain the slope variance, the random effect of the Level 1 variable involved in the cross-level interaction was added to the models.
Analysis
To standardize distance estimates across the target distances, we calculated the proportion of target distance walked by dividing participants’ distance estimates by the respective target distance. Proportion of target distance walked, therefore, served as the dependent measure in a multilevel mixed effects model. Phase (pretest vs. posttest), Environment (real vs. virtual), and Condition (matched, slower, or faster optic flow) served as independent measures.
There was a main effect of Phase, F(1, 1713) = 291.96, p < .001, sr2 = .15. Holding all other variables constant, the proportion of distance walked was greater in the posttest (M = 0.98, SE = 0.02) compared with the pretest (M = 0.89, SE = 0.02). There was no significant main effect of Condition or Environment.
As expected, there was a significant interaction between Phase and Condition, F(2, 1711) = 286.97, p < .001, sr2 = .59. There were significant differences between the pretest and posttest for all three optic flow conditions (see Fig. 2), and p values for the following pairwise comparisons were adjusted using the Tukey method for comparing a family of six estimates. In both the visually matched and visually slower conditions, the proportion of target distance walked was greater in the posttest compared with the pretest (ps < .001; see Fig. 3). In the visually faster condition, the proportion of target distance walked was smaller in the posttest compared with the pretest, t(1711) = −5.48, p < .001.
There was also a significant, yet trivial, interaction between Phase and Environment, F(1, 1712) = 7.69, p = .006, sr2 < .001. Pairwise comparisons revealed that differences existed between the different phases, but not between the testing environments. In both the real-world environment and the virtual environment, there was a significant difference in the proportion of target distance walked between the pretest and the posttest (ps < .001; see Fig. 4). However, there were no statistically significant differences in the proportion of target distance walked between the two testing environments for the pretest or the posttest. Further, the interaction between Condition and Environment was not statistically significant.
Discussion
In Experiment 1, we investigated transfer of calibration to perturbed optic flow to real-world and virtual environments to identify the effect of testing environment on calibration and distance perception. Although depth compression is a common issue in virtual environments, we did not find significant differences in distance perception between the real-world environment and the virtual environment. As expected, we found that individuals overshot distances after exposure to visually slower optic flow and undershot distances after exposure to visually faster optic flow. Furthermore, in the visually matched optic flow condition, participants overshot distances in the posttest, but this overshoot was consistent with near-perfect performance on the blind-walking distance estimation task. This finding is consistent with previous research suggesting that interacting with the environment is crucial for successfully calibrating one’s scaling of the environment (Brand & de Oliveira, 2017; Siegel & Kelly, 2017).
Like previous research (Durgin et al., 2005; Rieser et al., 1995), we also found an asymmetry in the magnitude of calibration between the visually slower and visually faster optic flow conditions. Participants in the visually slower condition overshot distances by approximately 29% on average, and participants in the visually faster condition undershot distances by approximately 3% on average. Finally, we found that the magnitude of calibration was not equivalent to the amount of gain received. Specifically, participants did not walk twice as far in the visually slower condition, or half as far in the visually faster condition. This is consistent with the idea that calibration occurs rapidly, but plateaus. Thus, the goal of Experiment 2 was to investigate how interleaving the calibration and posttest trials might affect the magnitude of calibration.
Experiment 2
The purpose of Experiment 2 was to investigate the possibility of increasing the effect of calibration to perturbed optic flow. Here, we utilized an adapted pretest-calibration-posttest design similar to Durgin et al. (2005), in which the calibration and posttest trials were interleaved. We sought to understand how interweaving the calibration and posttest trials affected distance estimation. Specifically, we were interested to see if distance estimates became more overestimated for the visually slower optic flow condition and more underestimated for the visually faster optic flow condition as the trials progressed. That is, we predicted that interweaving the calibration and posttest trials would produce a larger effect of calibration for both conditions.
Method
Participants
Based on the power analysis from Experiment 1, we assigned 10 participants to each condition. As such, 20 undergraduate students (nine females, age M = 19.7 years, SD = 1.2 years) from Clemson University participated in this study for partial fulfillment of course requirements after providing informed consent. All participants had normal or corrected-to-normal vision. Individuals with a history of epilepsy, seizures, neurological problems, or motor impairments were prohibited from participating in the study. The study was performed with approval of the Institutional Review Board of Clemson University.
Apparatus
Like in Experiment 1, the HTC Vive Pro System was used to track participants movements. Positional data from the HMD were used to obtain participants’ distance estimates.
Design and procedure
This experiment used an adapted pretest-calibration-posttest design to assess the effects of perturbed optic flow on blind walking estimates in virtual environments. As such, all three phases of the experimental design occurred in a virtual environment. Like in Experiment 1, the pretest and posttest environments were a virtual replication of the physical room, and the calibration environment was a virtual hallway. In the pretest, participants completed 15 blind walking trials to targets located 2, 3, and 4 meters away. This served as a baseline. Vision was occluded by graying out the screen on the HMD. The calibration and posttest phases for this experiment were adapted to be interleaved, such that participants completed a calibration trial, then a posttest trial, then a calibration trial, and so forth. The calibration trials required participants to complete sighted walking trials to targets located 2.5, 3.5, and 4.5 meters away. During the calibration trials, half of the participants experienced visually faster optic flow (translational gain of ×2), and half of the participants experienced visually slower optic flow (translational gain of ×0.5). During the posttest trials, participants completed blind walking trials to targets located 2, 3, and 4 meters away. Participants completed 30 interleaved trials (15 calibration trials and 15 posttest trials).
Results
Analysis preparation
Prior to conducting analyses, we assessed the extent to which the data were nested by obtaining the ICC from the null model. We found an ICC of 0.46, indicating that 46% of the variance in distance judgments was at the group level. To identify outliers in the data set, the residuals from the full model were saved and standardized. Observations with standardized score values falling outside of ±3 were removed from the data set. This outlier analysis resulted in less than 2% of the data being removed. Like Experiment 1, we utilized a conservative model approach. Additionally, effect sizes were calculated in the same manner as described in Experiment 1.
Analysis
For this experiment, we were interested in the differences between the two conditions and phases for each target distance. Like in Experiment 1, distance estimates were standardized across the target distances by converting them to a proportion. The proportion of target distance walked, therefore, served as the dependent measure in a multilevel mixed-effects model. Trial, Phase, and Condition served as independent measures.
There was a significant main effect of Trial, F(1, 571) = 7.03, p = .008, sr2 = .009. This effect was rather trivial, as the proportion of target distance walked was estimated to increase by approximately 0.003 for every increase in Trial, while holding all other variables constant. There was also a significant main effect of Phase, F(1, 571) = 8.55, p = .003, sr2 = .01. Holding all variables constant, the proportion of target distance walked was larger in the posttest (M = 1.03, SE = 0.04) compared with the pretest (M = 0.94, SE = 0.04). Lastly, there was a significant main effect of Condition, F(1,18) = 20.68, p < .001, sr2 = 0.5. Holding all variables constant, the proportion of target distance walked was larger for the visually slower condition (M = 1.12, SE = 0.04) compared with the visually faster condition (M = 0.84, SE = 0.04).
As expected, the interaction between Phase and Condition was statistically significant, F(1, 570) = 749.41, p < .001, sr2 = 0.82 (see Fig. 5). The p values for the following pairwise comparisons were adjusted using the Tukey method for comparing a family of four estimates. For the visually slower condition, the proportion of target distance walked was significantly greater in the posttest compared with the pretest, t(570) = 16.38, p < .001. Conversely, for the visually faster condition, the proportion of target distance walked was significantly smaller in the posttest compared with the pretest, t(570) = −8.43, p < .001 (see Fig. 6).
In the posttest, the proportion of distance walked was significantly greater in the visually slower condition compared with the visually faster condition, t(19) = 8.78, p < .001. There was no statistically significant difference in the proportion of distance walked between the visually faster and visually slower conditions in the pretest. To better understand the effect of interweaving the calibration and posttest trials, we assessed the effect of calibration for each condition at the start (Trial 1), middle (Trial 8), and end of the posttest (Trial 15) in a manner similar to Durgin et al. (2005). Relative to the average proportion of target distance walked in the pretest (baseline), participants in the visually slower condition overshot distances by an average of 28% at Trial 1 of the posttest (see Fig. 7). This is consistent with the idea that calibration to perturbed optic flow occurs rapidly. Relative to Trial 1, participants overshot distances by 14% at Trial 8 and by 22% at Trial 15 of the posttest. Overall, participants overshot distances by 49% relative to the baseline.
For the visually faster condition, participants undershot distances by an average of 11% at Trial 1 of the posttest relative to the baseline (see Fig. 7). Interestingly, participants overshot distances by 1% at Trial 8 and almost 4% at Trial 15 relative to Trial 1 of the posttest. Overall, participants undershot distances by 11% relative to the baseline.
Finally, we conducted independent-samples t tests to compare the mean proportions of target distances walked between the posttests of Experiments 1 and 2. For the visually slower conditions, we found that proportions were, on average, greater in Experiment 2, t(276) = 5.61, p < .001, indicating that participants overshot distances more in the posttest of Experiment 2 compared with Experiment 1 (see Fig. 8). For the visually faster conditions, we found that proportions were, on average, smaller in Experiment 2, t(250) = −4.6, p < .001, indicating that participants undershot distances more in the posttest of Experiment 2 than in the posttest of Experiment 1. These findings suggest that the magnitude of calibration was greater in Experiment 2 than in Experiment 1. Participants were able to more fully calibrate when they experienced the calibration and posttest trials in an interleaved manner than when the calibration and posttest trials were experienced in separate blocks.
Discussion
In Experiment 2, participants were exposed to visually slower or visually faster optic flow and asked to estimate distances via blind walking. For both conditions, we saw that calibration to perturbed optic flow occurred rapidly. After one trial of exposure to visually slower or visually faster optic flow, participants’ distance estimates increased by 28% or decreased by 11%, respectively. For the visually slower condition, interweaving the calibration and posttest trials appeared somewhat effective in extending the effect of calibration. That is, as participants were exposed to more trials of perturbed optic flow, their distance estimates increased. However, the increase in distance estimates (i.e., the magnitude of overshoot) was not systematic. For the visually faster condition, interweaving the calibration and posttest trials appeared less effective. Distance estimates at the first posttest trial were similarly underestimated when averaged across all trials. Looking at overall averages, we found that participants overshot distances by 49% in the posttest relative to the pretest for visually slower condition. This magnitude of calibration is greater than that documented by previous literature (e.g., Durgin et al., 2005). These results illustrate that there was a clear asymmetry between the visually slower and visually faster conditions. We note, however, that the magnitude of overshoot is still not equivalent to the magnitude of the perturbation to optic flow. Based on these findings, we were interested to see if we could further increase the magnitude of overshoot of distances by incorporating ankle loading. In Experiment 3, we investigated the effects of combining exposure to slower optic flow and bilateral ankle loading.
Experiment 3
In Experiments 1 and 2, we focused on perturbing optic information to determine how testing environment and experimental design might play a role in calibration. In Experiment 3, we wanted to investigate whether pairing the perturbed optic information with perturbed mechanical information would increase calibration effects. Previous research has shown that exposure to visually slower optic flow (e.g., Rieser et al., 1995) and loading of the ankles (Paquet et al., 2015) both lead to overestimations in distance walked to previously seen visual targets. As such, Experiment 3 involved evaluating the effects of ankle loading on calibration to perturbed optic flow. The hypothesis was that by causing the participants to calibrate their walking to the ankle weights, thus inducing an additional adjustment in their walking behavior, would cause them to more completely calibrate to the altered optic flow, as the perturbed optic flow would be utilized in adjusting to the added weight. This experiment utilized a standard pretest-calibration-posttest design, where all participants experienced visually slower optic flow during the calibration phase, which was followed by posttest trials in a separate phase, like in Experiment 1. In a between-subjects design, ankle weights were added to participants’ ankles during the calibration phase only, during both the calibration phase and the posttest phase, or during all three phases. Experiment 1 provided a comparison condition with no ankle weights in any of the phases. We predicted that the addition of ankle weights would magnify the overshooting of distances that is typical of calibration to slower optic flow.
Method
Participants
Based on the power analysis from Experiment 1, we assigned 10 participants to each condition. As such, 30 undergraduate students (24 females, age M = 19.4 years, SD = 3.1 years) from Clemson University participated in this study for partial course fulfillment after providing informed consent. All participants had normal or corrected-to-normal vision. Individuals with a history of epilepsy, seizures, neurological problems, or motor impairments were prohibited from participating in the study.
Apparatus
Like Experiment 1, the HTC Vive Pro System was used to track participants’ movements. Positional data from the HMD was used to obtain participants’ distance estimates. The experimenter fit the participant with 2.27 kg (5 lbs.) weights on each ankle via a Velcro strap.
Design and procedure
This experiment utilized a pretest-calibration-posttest design. All phases of this experiment were completed in the same virtual environments employed in Experiment 1. Like Experiment 1, participants completed 15 blind walking trials to targets 2, 3, and 4 meters away in the pretest and posttest. In the intervening calibration phase, all participants experienced a visually slower optic flow (translational gain ×0.5) while completing 15 sighted walking trials to targets 2.5, 3.5, and 4.5 meters away. Ten participants were assigned to each of the ankle weight conditions: ankle weights worn only during the calibration phase (C), ankle weights worn during the calibration and posttest phase (CP), or ankle weights worn during all three phases (PCP). The data for a fourth baseline condition were taken from the 10 participants in Experiment 1 who were assigned to the virtual testing environment and visually slower optic flow. This condition was identical to those of the present experiment, except that the participants did not wear ankle weights. This will be referred to as the no weight (NW) condition.
Results
Analysis preparation
Prior to conducting analyses, we assessed the extent to which the data were nested by obtaining the intraclass correlation coefficient (ICC) from the null model. We found an ICC of 0.31, indicating that 31% of the variance in distance judgments was at the group level. As such, multilevel mixed-effects modeling was utilized for analysis. To identify outliers in the data set, the residuals from the full model were saved and standardized. Observations with standardized score values falling outside of ±3 were removed from the data set. This outlier analysis resulted in less than 2% of the data being removed. Like in Experiment 1, we utilized a conservative model approach. Effect sizes were calculated in the same manner described in Experiment 1.
Analysis
Like Experiment 1, distance estimates were standardized across the target distances by converting them to a proportion. The proportion of target distance walked, therefore, served as the dependent measure in a multilevel mixed effects model. Trial, Phase, and Condition served as independent measures.
There was a main effect of Phase, F(1, 1132) = 86.48, p < .001, sr2 = .04. Holding all variables constant, the proportion of target distance walked was larger in the posttest (M = 1.15, SE = 0.02) compared with the pretest (M = 0.91, SE = 0.02). There was also a main effect of Condition, F(1, 36) = 2.93, p = .047, sr2 = .08. The proportion of target distance walked was approximately 1.0 for all conditions, with pretest and posttest combined. Pairwise comparisons using a p-value adjustment for a family of four estimates revealed that the proportion of distance walked in the C condition (M = 1.11, SE = 0.04) was significantly different than the proportion of distance walked in the PCP condition, M = 0.96, SE = 0.04, t(36) = 2.97, p = .026. There were no other significant differences between conditions in the proportion of target distance walked. There was also no main effect of trial.
As expected, there was a significant interaction between Phase and Condition, F(3, 1129) = 70.57, p < .001, sr2 = .3. The p values for the following pairwise comparisons were adjusted using the Tukey method for comparing a family of eight estimates. For all four conditions, the proportion of target distance walked was significantly different between the pretest and posttest (ps < .01). Specifically, participants walked farther in the posttest compared with the pretest in all conditions (see Fig. 9). However, the magnitude of calibration transfer differed across the conditions. Relative to their respective pretests, participants overshot distances by 42% in the NW condition, by 9% in the C condition, by 25% in the CP condition, and by 36% in the PCP condition. For the pretest alone, the proportion of target distance walked was significantly different in the C condition compared with the NW condition, t(38) = 3.87, p = .009, and compared with the PCP condition, t(38) = 4.67, p = .001. The reason for this effect is unknown, as all participants experienced the same pretest. Notably, the proportion of target distance walked in the posttest did not significantly differ among the four conditions. That is, participants’ distance estimates were statistically similar across all conditions in the posttest.
Discussion
In this experiment, participants were exposed to slower optic flow while either wearing or not wearing ankle weights during the different phases of the experiment. We saw that participants calibrated to perturbed optic flow in each of the ankle weight conditions. The magnitude of this effect, however, varied greatly among the different conditions. Previous research suggested that ankle loading increases distance estimations (Paquet et al., 2015). As such, we predicted that combining ankle loading and slower optic flow would result in the greatest overestimations of distance. Interestingly, the greatest effect of calibration was evidenced by participants in the NW condition, where participants’ ankles were not loaded with weights in any of the phases. In this condition, participants walked 42% farther in the posttest relative to the pretest. There was still an effect of calibration for the CP and PCP ankle loading conditions, but it was smaller than that of the NW condition.
In the C condition, the effect of calibration was minimal; participants’ distance estimates were only slightly different between the pretest and posttest. These results suggest that the addition of ankle weights was not effective in increasing the magnitude of calibration to perturbed optic flow. This also suggests that walking with ankle weights is a different task than walking without them, as the least amount of calibration was evident when the posttest was incongruent with the calibration phase—namely when participants walked with weights during calibration, but then did not walk with them during the posttest. This is consistent with past findings that calibration does not transfer between two tasks that are sufficiently different from each other (Bingham et al., 2014; Bruggeman & Warren, 2010; Proffitt, 2008; Rieser et al., 1995; Witt, 2011; Witt et al., 2010)
General discussion
In the present study, we investigated how different experimental factors influenced calibration. Specifically, we assessed how testing environment (Experiment 1), an adapted pretest-calibration-posttest design (Experiment 2), and bilateral ankle loading (Experiment 3) affected the magnitude of calibration to perturbed optic flow. Consistent with previous findings (Adams et al., 2018; Durgin et al., 2005; Kunz et al., 2013, 2015; Mohler et al., 2007; Rieser et al., 1995), we found that participants calibrated to perturbed optic flow, such that those in the visually slower condition overshot distances, and those in the visually faster condition undershot distances. Previously, researchers have shown that individuals estimate distances near perfectly in the real world and underestimate distances in virtual environments (Geuss et al., 2012; Loomis et al., 1992; Loomis & Knapp, 2003; Sahm et al., 2005). Here, however, we found that in the pretest, participants underestimated distances in both testing environments. Although this was unexpected, it suggests that interaction is crucial for the appropriate scaling of perceptual-motor activity within novel environments. That successful interaction with an environment requires intrinsic scaling of the environment is further supported by our findings in the visually matched condition. In this condition, participants initially underestimated distances, but upon receiving appropriate visual information in the calibration phase (i.e., simulated optic flow properly matched to their walking rate), participants judged distances accurately in the posttest. This is consistent with recent findings on the value of walking interaction with virtual environments. For instance, Siegel and Kelly (2017) found that allowing participants an opportunity to physically walk within a virtual environment successfully reduced depth compression in both near and far space.
Like the pretest, we found that participants estimated distances similarly in both testing environments in the posttest, indicating that calibration was similar regardless of testing environment. That is, we did not find any significant differences in distance estimates between the real-world testing environment and the virtual testing environment. This contradicts previous findings that calibration magnitude is greater in virtual environments than in real-world environments (Ziemer et al., 2013). This discrepancy is possibly explained by the differences in distance estimation task (imagined walking vs. blind walking) and virtual reality technology employed (large screen display vs. head-mounted display) between Ziemer et al.’s (2013) study and the present study. The lack of difference in distance estimates between the testing environments found here is most likely explained by the use of newer virtual reality technology, as distance perception via the HTC Vive has been shown to be similar to real-world distance perception (Kelly et al., 2017).
We found that calibration carried over from a virtual calibration phase to both real and virtual posttest phases in a like manner, but that calibration during walking with ankle weights had very little carryover to walking without them. Given the task-specific nature of calibration (Bingham et al., 2014; Bruggeman & Warren, 2010; Proffitt, 2008; Rieser et al., 1995; Witt, 2011; Witt et al., 2010), this indicates that walking in VR is a similar task to walking in the real world, while walking with ankle weights is not the same task as walking without them.
Overall, our findings suggest that the issue of depth compression in VR can be easily mitigated by allowing individuals an opportunity to interact with the virtual environment prior to task completion or assessment. Our prior work involving manual reaches resulted in a similar conclusion (Altenhoff et al., 2012; Ebrahimi, Altenhoff, Pagano, & Babu, 2015). The similarity between the real-world and virtual testing environments implicate VR as a useful and valuable tool for assessing distance perception and calibration. Further, these findings suggest that rescaling of a virtual environment to reduce depth compression may be unnecessary.
In Experiments 1 and 2, we found that all participants exhibited calibration, but that the magnitude of calibration was greatest for the visually slower condition. This is consistent with previous findings that there is an asymmetry in the magnitude of calibration between visually slower and visually faster optic flow conditions (Durgin et al., 2005; Rieser et al., 1995). In Experiment 1, we found that participants in the visually slower condition overshot distances by approximately 29% on average, and participants in the visually faster condition undershot distances by approximately 3% on average. Durgin et al. (2005) claim that the use of a treadmill may have contributed to the asymmetry in calibration magnitude. Because treadmills were not utilized in the present experiment, the asymmetry may have been due to a lack of appropriate perceptual scaling in the initial pretest. This is suggested by the rescaling of walking distance between the pretest and posttest phases in the visually matched condition. The asymmetry found in both experiments may also be explained by how individuals scale their perceptions of walking rate from simulated optic flow rate in VR. For example, when asked to match walking rate to optic flow rate in a virtual environment, individuals matched a faster optic flow rate to their walking rate (Banton, Stefanucci, Durgin, Fass, & Proffitt, 2005). If a faster optic flow rate is more of a match to perceived walking speed, this may explain why exposure to faster optic flow rate results in minimal undershooting of distances compared with exposure to slower optic flow. Once again, however, it should be noted that a high level of accuracy was achieved with visually matched optic flow in both the real and virtual posttest conditions, after participants calibrated in VR during the calibration phase. This underscores the efficacy of calibration to remedy perceptual-motor distortions in VR, and other perturbing environments (Altenhoff et al., 2012; Bingham & Pagano, 1998; Ebrahimi et al., 2015).
The results of Experiment 2 further showed that interleaving the calibration and posttest trials was an effective method for increasing the magnitude of calibration. Compared with Experiment 1, participants overshot distances more in the visually slower condition and undershot distances more in the visually faster condition. That is, participants in Experiment 2 more fully calibrated to the perturbed optic flow than the participants in Experiment 1. This suggests that experimental design is a critical component in the evaluation of calibration. Lastly, the findings from Experiment 2 illustrated that interleaving the calibration and posttest trials was mostly effective when perturbed optic flow was slower than participants’ walking rates. Participants exposed to slower optic flow overestimated distances by almost 50%, which is more than twice that documented by Durgin et al. (2005). Despite this increase in overall magnitude, calibration still plateaued. Unlike the visually slower condition, the plateau in calibration was almost instantaneous for the visually faster condition (see Fig. 7). These findings support the idea that calibration can occur rapidly.
In Experiment 3, we found that adding weights to participants’ ankles did not effectively increase their distance estimates. When participants only wore ankle weights during the calibration phase, there was only a slight difference in their distance estimates between the pretest and posttest. These results suggest that exposing participants to slower optic flow and bilateral ankle loading in a simultaneous manner was not an effective method for increasing the magnitude of calibration to perturbed optic flow. One possible explanation for this lack of calibration is that there was insufficient functional and informational similarity between the conditions of the calibration phase and testing phase (Franchak, 2020). For example, in a prism adaptation study, Fernández-Ruiz et al. (2000), examined the effects of loading the wrist with weights at different points during a pretest, calibration phase, and posttest. After calibration the participants exhibited an aftereffect (i.e., they underthrew to the targets). This aftereffect was more pronounced if the participants kept the weights on during the posttest, and it could be extinguished over time when participants made throws without the weights in the posttest. However, this aftereffect reappeared when the participants put the weights back onto their wrists. Fernández-Ruiz et al. (2000) argued that muscle memory, therefore, “is at least partly restricted to the specific state of the set of muscles during the adaptation process” (p. 197). Put differently, calibration is task specific, in that it will only transfer to actions that are similar to those in which the calibration occurred (Bingham et al., 2014; Bruggeman & Warren, 2010; Proffitt, 2008; Rieser et al., 1995; Witt, 2011; Witt et al., 2010). Literature reviews have underscored this task specificity, as well as the finding that the speed of calibration is contingent on the allowance of active exploration and the aptness of the information available to the perceptual-motor system (Brand & Oliveira, 2017; Fajen, 2005; van Andel et al., 2017).
That calibration depends on functional and information similarity would suggest that maintaining ankle loading during both the calibration and posttest phases would produce an overshoot in distances, which is what we found. In the CP condition, we saw that participants overwalked more relative to both the pretest and the C condition. We noted, however, that the overwalking in the CP condition could have been for two reasons. Participants may have overwalked because of calibration or simply because of ankle loading (see Paquet et al., 2015). The purpose of the PCP condition, where ankle weights were worn for the duration of the study, was to help elucidate the reason for overwalking in the CP condition. The amount of overshoot in both the CP and PCP conditions were similar, suggesting that the overshoot in the CP condition was not simply because ankle weights may lead to overestimations of distance. That is, participants in both conditions exhibited calibration. Looking at the pretest distance estimates from the PCP condition, we found that participants underestimated distances when encumbered with ankle weights, which was contrary to Paquet et al.’s (2015) findings. Paquet et al. claimed that overwalking when encumbered with ankle weights may have been due to a misperception in forward displacement. Specifically, they argued that individuals underestimated their forward displacement, resulting in overwalking. Given that individuals have been shown to underestimate arm length when the hand/arm were weighted (Shibata et al., 2012), a misperception in forward displacement could explain Paquet et al.’s (2015) findings, but it would not explain the findings from the present study.
Although we found that participants equally underestimated distances in the both real-world and virtual environments, it is possible that depth compression contributed to the underestimation found in the PCP condition. Paquet et al.’s (2015) research was conducted in a real-world environment, whereas our study was conducted in a virtual environment. Perhaps allowing participants to walk unencumbered in the virtual environment prior to the blind walking task would provide a more accurate with rescaling of the environment. When encumbered with ankle weights, participants did not fully experience the perturbation until they engaged in the blind walking task. As such, it is possible that participants initially made distance estimates based on the perceived energy expenditure for walking without ankle weights. It is also likely that participants calibrated to the ankle weights after a few trials of walking, which may have impacted our findings. Future research comparing distance estimation with and without an initial walking interaction (similar to Mark, 1987) is needed to disentangle how individuals calibrate to bilateral ankle loading. Lastly, although the magnitude of overestimation for both the CP and PCP conditions was greater than that found in the literature, the magnitude of overshoot for all three ankle weight conditions was less than that in the NW condition. Thus, the results of Experiment 3 suggest that combining ankle weights with slower optic flow is not an effective method for increasing the magnitude of calibration.
In conclusion, the experiments presented here illustrate that the asymmetry and plateau effects found in calibration to perturbed optic flow are not easily mitigated. Though we were able to successfully increase the magnitude of calibration transfer, compared with previous studies, it was clear that calibration occurred rapidly and quickly plateaued. Across the three experiments, we found that interleaving the calibration and posttest trials (Experiment 2) was the most effective method for increasing the magnitude of calibration. With respect to the asymmetry effect, we suggest this is due to a lack of appropriate scaling to the rate of optic flow prior to calibration, though future research is needed to fully identify the source of this effect. We replicated findings that perceptual-motor activity mitigates depth compression in VR and that distance perception in a newer VR system is analogous to distance perception in the real world. We were unable to replicate findings that ankle loading increases distance estimation. Therefore, future research is needed to understand how bilateral ankle loading, and similar motoric perturbations, affect end limb positioning and distance estimation.
References
Adams, H., Narasimham, G., Rieser, J., Creem-Regehr, S., Stefanucci, J., & Bodenheimer, B. (2018). Locomotive recalibration and prism adaptation of children and teens in immersive virtual environments. IEEE Transactions on Visualization and Computer Graphics, 24(4), 1408–1417. https://doi.org/10.1109/TVCG.2018.2794072
Altenhoff, B. M., Napieralski, P. E., Long, L. O., Bertrand, J. W., Pagano, C. C., Babu, S. V., & Davis, T. A. (2012). Effects of visual and haptic feedback on near-field depth perception in an immersive virtual environment. Proceedings of the ACM Symposium on Applied Perception (pp. 71–78). New York, NY: ACM.
Banton, T., Stefanucci, J., Durgin, F., Fass, A., & Proffitt, D. (2005). The perception of walking speed in a virtual environment. Presence: Teleoperators and Virtual Environments, 14(4), 394–406. https://doi.org/10.1162/105474605774785262
Bertenthal, B. I., Rose, J. L., & Bai, D. L. (1997). Perception-action coupling in the development of visual control of posture. Journal of Experimental Psychology: Human Perception and Performance, 23(6), 1631–1643.
Bhargava, A., Lucaites, K. M., Hartman, L. S., Solini, H., Bertrand, J. W., Robb, A. C., … Babu, S. V. (2020). Revisiting affordance perception in contemporary virtual reality. Virtual Reality, 1–12. https://doi.org/10.1007/s10055-020-00432-y
Bickel, R. (2007). Multilevel analysis for applied research: It’s just regression! New York, NY: Guilford Press.
Bingham, G. P., & Pagano, C. C. (1998). The necessity of a perception-action approach to definite distance perception: Monocular distance perception to guide reaching. Journal of Experimental Psychology: Human Perception and Performance, 24, 145–168. https://doi.org/10.1037/0096-1523.24.1.145
Bingham, G. P., Pan, J. S., & Mon-Williams, M. A. (2014). Calibration is both functional and anatomical. Journal of Experimental Psychology: Human Perception and Performance, 40, 61–70. https://doi.org/10.1037/a0033458
Brand, M. T., & de Oliveira, R. F. (2017). Recalibration in functional perceptual-motor tasks: A systematic review. Human Movement Science, 56, 54–70. https://doi.org/10.1016/j.humov.2017.10.020
Browning, R. C., Modica, J. R., Kram, R., & Goswami, A. (2007). The effects of adding mass to the legs on the energetics and biomechanics of walking. Medicine & Science in Sports & Exercise, 39, 515–525.
Bruggeman, H., & Warren, W. H. (2010). The direction of walking—but not throwing or kicking—is adapted by optic flow. Psychological Science, 21(7), 1006–1013. https://doi.org/10.1177/0956797610372635
Cohen, J., Cohen, P., West, S. G., & Aiken, L. S. (2003). Applied multiple correlation/regression analysis for the social sciences (3rd ed.). Mahwah, NJ: Erlbaum.
Day, B., Ebrahimi, E., Hartman, L.S., Pagano, C. C., Robb, A. C., & Babu, S. V. (2019). Examining the effects of altered avatars on perception-action in virtual reality. Journal of Experimental Psychology: Applied, 25, 1–24.
Durgin, F. H., Pelah, A., Fox, L. F., Lewis, J., Kane, R., & Walley, K. A. (2005). Self-motion perception during locomotor recalibration: More than meets the eye. Journal of Experimental Psychology: Human Perception and Performance, 31(3), 398–419. https://doi.org/10.1037/0096-1523.31.3.398
Ebrahimi, E., Altenhoff, B., Pagano, C. C., & Babu, S. V. (2015, March 23–24). Carryover effects of calibration to visual and proprioceptive information on near field distance judgments in 3D user interaction. Proceedings of the IEEE 10th Symposium on 3D User Interfaces, Arles, France.
Fajen, B. R. (2005). Perceiving possibilities for action: On the necessity of calibration and perceptual learning for the visual guidance of action. Perception, 34, 717–740.
Fernández-Ruiz, J., Hall-Haro, C., Díaz, R., Mischner, J., Vergara, P., & Lopez-Garcia, J. C. (2000). Learning motor synergies makes use of information on muscular load. Learning & Memory, 7(4), 193–198.
Franchak, J. M. (2020). Calibration of perception fails to transfer between functionally similar affordances. Quarterly Journal of Experimental Psychology, 73, 1311–1325. https://doi.org/10.1177/1747021820926884
Geuss, M. N., Stefanucci, J. K., Creem-Regehr, S. H., & Thompson, W. B. (2012). Effect of viewing plane on perceived distances in real and virtual environments. Journal of Experimental Psychology: Human Perception and Performance, 38(5), 1242–1253. https://doi.org/10.1037/a0027524
Graves, J. E., Martin, D., Miltenberger, L. A., & Pollock, M. L. (1988). Physiological responses to walking with hand weights, wrist weights, and ankle weights. Medicine & Science in Sports & Exercise, 20(3), 265–271.
Harrison, S. J. (2020). Human odometry with a two-legged hopping gait: A test of the gait symmetry theory. Ecological Psychology, 32(1), 58–78. https://doi.org/10.1080/10407413.2019.1708200
Kelly, J. W., Cherep, L. A., & Siegel, Z. D. (2017). Perceived space in the HTC Vive. ACM Transactions on Applied Perception, 15(1), 1–16. https://doi.org/10.1145/3106155
Kunz, B. R., Creem-Regehr, S. H., & Thompson, W. B. (2009). Evidence for motor simulation in imagined locomotion. Journal of Experimental Psychology: Human Perception and Performance, 35(5), 1458–1471. https://doi.org/10.1037/a0015786
Kunz, B. R., Creem-Regehr, S. H., & Thompson, W. B. (2013). Does perceptual-motor calibration generalize across two different forms of locomotion? Investigations of walking and wheelchairs. PLOS ONE, 8(2), e54446. https://doi.org/10.1371/journal.pone.0054446
Kunz, B. R., Creem-Regehr, S. H., & Thompson, W. B. (2015). Testing the mechanisms underlying improved distance judgments in virtual environments. Perception, 44(4), 446–453. https://doi.org/10.1068/p7929
Loomis, J. M., da Silva, J. A., Fujita, N., & Fukusima, S. S. (1992). Visual space perception and visually directed action. Journal of Experimental Psychology: Human Perception and Performance, 18(4), 906–921. https://doi.org/10.1037/0096-1523.18.4.906
Loomis, J. M., & Knapp, J. M. (2003). Visual perception of egocentric distance in real and virtual environments. In L. J. Hettinger & M. W. Haas (Eds.), Virtual and adaptive environments: Applications, implications, and human performance issues (Vol. 11, pp. 21–46). Mahwah, NJ: Erlbaum. https://doi.org/10.1201/9781410608888.pt1
Mark, L. S. (1987). Eyeheight-scaled information about affordances: A study of sittting and stair climbing. Journal of Experimental Psychology: Human Perception and Performance, 13(3), 361–370.
Martin, T. A., Keating, J. G., Goodkin, H. P., Bastian, A. J., & Thach, W. T. (1996). Throwing while looking through prisms: II. Specificity and storage of multiple gaze--throw calibrations. Brain, 119(4), 1199–1211. https://doi.org/10.1093/brain/119.4.1199
Mohler, B. J., Thompson, W. B., Creem-Regehr, S. H., Herbert, P., Warren, W., Rieser, J. J., & Willemsen, P. (2004). Visual motion influences locomotion in a treadmill virtual environment. Proceedings of the 1st Symposium on Applied Perception in Graphics and Visualization (pp. 19–22). https://doi.org/10.1145/1012551.1012554
Mohler, B. J., Thompson, W. B., Creem-Regehr, S. H., Willemsen, P., Pick, Jr., H. L., & Rieser, J. J. (2007). Calibration of locomotion resulting from visual motion in a treadmill-based virtual environment. ACM Transactions on Applied Perception, 4(1), 4. https://doi.org/10.1145/1227134.1227138
Pagano, C. C., & Isenhower, R. W. (2008). Expectation affects verbal judgments but not reaches to visually perceived egocentric distances. Psychonomic Bulletin & Review, 15, 437–442.
Pan, J. S., Coats, R. O., & Bingham, G. P. (2014). Calibration is action specific but perturbation of perceptual units is not. Journal of Experimental Psychology: Human Perception and Performance, 40, 404–415. https://doi.org/10.1037/a0033795
Paquet, N., Taillon-Hobson, A., & Lajoie, Y. (2015). Effect of ankle weight on blind navigation. Perceptual and Motor Skills, 120(2), 502–518. https://doi.org/10.2466/25.PMS.120v10x0
Proffitt, D. R. (2008). An action-specific approach to spatial perception. In R. Klatzky, B. MacWhinney, & M. Berhmann (Eds.), Embodiment, ego-space, and action (pp. 179–202). Mahwah, NJ: Erlbaum.
Proffitt, D. R., Stefanucci, J., Banton, T., & Epstein, W. (2003). The role of effort in perceiving distance. Psychological Science, 14(2), 106–112. https://doi.org/10.1111/1467-9280.t01-1-01427
Redding, G. M., & Wallace, B. (2003). Dual prism adaptation: Calibration or alignment? Journal of Motor Behavior, 35(4), 399–408. https://doi.org/10.1080/00222890309603159
Rieser, J. J., Pick, H. L., Ashmead, D. H., & Garing, A. E. (1995). Calibration of human locomotion and models of perceptual-motor organization. Journal of Experimental Psychology: Human Perception and Performance, 21(3), 480–497.
Sahm, C. S., Creem-Regehr, S. H., Thompson, W. B., & Willemsen, P. (2005). Throwing versus walking as indicators of distance perception in similar real and virtual environments. ACM Transactions on Applied Perception, 2(1), 35–45. https://doi.org/10.1145/1048687.1048690
Shibata, H., Gyoba, J., & Takeshima, Y. (2012). Perception of the end position of a limb loaded with a weight. Attention, Perception, & Psychophysics, 74(1), 225–238. https://doi.org/10.3758/s13414-011-0232-5
Siegel, Z. D., & Kelly, J. W. (2017). Walking through a virtual environment improves perceived size within and beyond the walked space. Attention, Perception, & Psychophysics, 79(1), 39–44. https://doi.org/10.3758/s13414-016-1243-z
Skinner, H. B., & Barrack, R. L. (1990). Ankle weighting effect on gait in able-bodied adults. Archives of Physical Medicine and Rehabilitation, 71(2), 112–115.
Snijders, T. A., & Bosker, R. J. (2011). Multilevel analysis: An introduction to basic and advanced multilevel modeling. Thousand Oaks, CA: SAGE Publications.
Solini, H. M., Bhargava, A., & Pagano, C. C. (2019). Transfer of calibration in virtual reality to both real and virtual environments. Proceedings of the Human Factors and Ergonomics Society Annual Meeting, 63(1), 1943–1947. https://doi.org/10.1177/1071181319631224
van Andel, S., Cole, M. H., & Pepping, G. J. (2017). A systematic review on perceptual-motor calibration to changes in action capabilities. Human Movement Science, 51, 59–71. https://doi.org/10.1016/j.humov.2016.11.004
Van der Leeden, R., & Busing, F. M. T. A. (1994). First iteration versus IGLS/RIGLS estimates in two-level models: A Monte Carlo study with ML3. Preprint PRM, 94(03).
Walter, H. J., Peterson, N., Li, R., Wagman, J. B., & Stoffregen, T. A. (2019). Sensitivity to changes in dynamic affordances for walking on land and at sea. PLOS ONE, 14(10), e0221974.
Warren, W. H. (1988). Action modes and laws of control for the visual guidance of action. In Advances in Psychology (Vol. 50, pp. 339–379). Elsevier. https://doi.org/10.1016/S0166-4115(08)62564-9
Warren, W. H. (1990). The perception-action coupling. In H. Bloch & B. I. Bertenthal (Eds.), Sensory-Motor Organizations and Development in Infancy and Early Childhood (pp. 23–37). Heidelberg, Germany: Springer Netherlands. https://doi.org/10.1007/978-94-009-2071-2_2
Witt, J. K. (2011). Action’s effect on perception. Current Directions in Psychological Science, 20, 201-206.
Witt, J. K., Proffitt, D. R., & Epstein, W. (2010). When and how are spatial perceptions scaled? Journal of Experimental Psychology: Human Perceptionand Performance, 36, 1153–1160. https://doi.org/10.1037/a0019947
Woltman, H., Feldstain, A., MacKay, J. C., & Rocchi, M. (2012). An introduction to hierarchical linear modeling. Tutorials in Quantitative Methods for Psychology, 8(1), 52–69. https://doi.org/10.20982/tqmp.08.1.p052
Ziemer, C. J., Branson, M. J., Chihak, B. J., Kearney, J. K., Cremer, J. F., & Plumert, J. M. (2013). Manipulating perception versus action in recalibration tasks. Attention, Perception, & Psychophysics, 75(6), 1260–1274. https://doi.org/10.3758/s13414-013-0473-6
Acknowledgements
Preliminary findings from Experiment 1 were presented at the 2019 Annual Meeting of the Human Factors and Ergonomics Society, Seattle, WA (Solini, Bhargava, & Pagano, 2019).
Open practices statement
The data for these experiments can be obtained from the first author. Email: hsolini@g.clemson.edu. None of the experiments was preregistered.
Author information
Authors and Affiliations
Corresponding author
Additional information
Publisher’s note
Springer Nature remains neutral with regard to jurisdictional claims in published maps and institutional affiliations.
Rights and permissions
About this article
Cite this article
Solini, H.M., Bhargava, A. & Pagano, C.C. The effects of testing environment, experimental design, and ankle loading on calibration to perturbed optic flow during locomotion. Atten Percept Psychophys 83, 497–511 (2021). https://doi.org/10.3758/s13414-020-02200-1
Accepted:
Published:
Issue Date:
DOI: https://doi.org/10.3758/s13414-020-02200-1