Abstract
Hippocampal blood–brain barrier (BBB) permeability may increase in normal healthy ageing and contribute to neurodegenerative disease. To examine this hypothesis, we investigated the correlation between blood–brain barrier (BBB) permeability, regional brain volume, memory functions and health and lifestyle factors in The Metropolit 1953 Danish Male Birth Cohort. We used dynamic contrast-enhanced magnetic resonance imaging (DCE-MRI) with a gadolinium-based contrast agent to assess BBB permeability in 77 participants in the cohort. BBB permeability was measured as Ki values in the hippocampus, thalamus and white matter. Over a 10-year period, we observed progressive atrophy of both the left and right hippocampus (p = 0.001). There was no significant correlation between current BBB permeability and hippocampal volume, prior atrophy or cognition. The hippocampus volume ratio was associated with better visual and verbal memory scores (p < 0.01). Regional BBB differences revealed higher Ki values in the hippocampus and white matter than in the thalamus (p < 0.001). Participants diagnosed with type II diabetes had significantly higher BBB permeability in the white matter (p = 0.015) and thalamus (p = 0.016), which was associated with a higher Fazekas score (p = 0.024). We do not find evidence that BBB integrity is correlated with age-related hippocampal atrophy or cognitive functions. The association between diabetes, white matter hyperintensities and increased BBB permeability is consistent with the idea that cerebrovascular disease compromises BBB integrity. Our findings suggest that the hippocampus is particularly prone to age-related atrophy, which may explain some of the cognitive changes that accompany older age, but this prior atrophy is not correlated with current BBB permeability.
Similar content being viewed by others
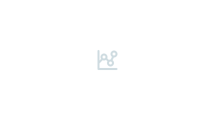
Introduction
The blood–brain barrier (BBB) is a highly specialized protective structure between the blood vessels in the brain and brain tissue. The BBB consists of endothelial cells adjoined by tight junctions and sheathed by perivascular mural cells called pericytes [1,2,3,4]. This structure creates a barrier that limits the entry of neurotoxic plasma components, red blood cells, pathogens and leucocytes into the brain [2, 5]. The BBB is critical for maintaining the chemical composition of the brain interstitial fluid, which is crucial for optimal synaptic function, information processing and neuronal connectivity [4]. Breakdown of the BBB will allow toxic components to enter the brain, which compromises synaptic and neuronal function and may trigger neurodegeneration [4,5,6,7].
BBB permeability, ageing and neurodegeneration may be linked, but this issue is controversial. Some studies have observed significant associations between BBB integrity, ageing and neurodegeneration [2, 8,9,10], whereas other studies have not [11, 12]. Furthermore, there are disagreements regarding the distribution of changes among brain regions that are difficult to resolve. Some studies report that the hippocampus and its subregions are particularly vulnerable to age-related decline and speculate that loss of BBB integrity in these areas may be a precursor to neurodegenerative diseases, such as Alzheimer’s disease (AD) [2, 8, 9]. Conversely, other studies suggest that the BBB integrity in the white and cortical grey matter is the first to suffer an age-related decline and that this may be associated with increased vascular burden [10, 12,13,14].
Furthermore, it is well-established that our brains undergo atrophy as we age, with annual atrophy rates of 0.2–0.5% [15,16,17]. It has been suggested that the medial temporal lobes and the hippocampal structure are particularly vulnerable to age-related atrophy and that this vulnerability is partially associated with reduced memory functions [18,19,20,21,22]. The hippocampus is also a hallmark structure in AD, and studies have suggested that the hippocampus volume may be used to predict conversion from MCI to AD [23, 24]. However, little is known regarding the association between the hippocampal atrophy rate and BBB permeability.
The present study examined BBB permeability in middle-aged men from The Metropolit 1953 Danish Male Birth Cohort [25] that had been examined repeatedly with regard to cognitive skills and general and brain health since birth. Our first objective was to investigate whether BBB integrity in the hippocampus and white matter accompanied cognitive function in this group of aged males. The second objective was to examine whether there is an association between hippocampal BBB integrity and age-related volume loss in the hippocampus over a 10-year period. Our final objective was to explore whether BBB integrity changes are associated with health and lifestyle factors, such as education, body mass index (BMI) and smoking and drinking habits, and with relevant clinical diagnoses, such as hypertension, stroke and heart disease.
Methods
Participants
Participants were recruited from The Metropolit 1953 Danish Male Birth Cohort. The Metropolit cohort was established in 1965 and consists of men born in the Copenhagen Metropolitan area in 1953 [25]. The participants were examined physiologically and cognitively at several time points throughout their lives, making them ideal for a longitudinal ageing study. Previous studies from this cohort have focused on age-related changes in brain structures [26], electrical activity [27,28,29,30] and cerebrovascular function [31]. For the present study, we recruited a subset of participants from the cohort who had been examined at three different timepoints 5 years apart, with the first examination taking place in 2011/2012 (T1), the second in 2015/2016 (T2) and the third in 2021/2022 (T3). The demographic, health and lifestyle characteristics of the participants are presented in Table 1. The sample sizes for each timepoint and measurement are presented in Table 2.
Neuropsychological examination
Cognitive functions were assessed at all timepoints using a comprehensive neuropsychological test battery. Since this article addresses the role of the hippocampus in ageing and cognition, we only considered cognitive tests dependent and sensitive to the integrity of the hippocampus and the medial temporal lobes. The verbal paired associates (VPA) test was used to measure verbal memory. Visual memory was assessed using paired associative learning (PAL) and pattern recognition memory (PRM) tests, which are part of the computerized Cambridge Neuropsychological Test Automated Battery (CANTAB). For the PAL score, we used the total errors at 6 and 8 shapes, since they are found to be particularly sensitive for detecting early cognitive decline. The mini-mental state examination (MMSE) was used to rule out dementia. All neuropsychological tests were administered by certified hospital staff.
MRI acquisition and processing
Magnetic resonance imaging (MRI) was performed on a Philips Achieva 3 T scanner (Philips, Best, The Netherlands) with a 32-channel phased array head coil. Whole-brain 3D T1-weighted high-resolution anatomical scans were used to assess volumetry (echo time (TE) = 5.11 ms; repetition time (TR) = 11.2 ms; flip angle = 8°, field of view (FOV) = 240 × 256 × 180 mm3; voxel size = 0.70 × 0.76 × 0.70 mm3). Images were segmented using the standard longitudinal segmentation pipeline in FreeSurfer software suite (v7.1.1, Martinos Center for Biomedical Imaging, Massachusetts, USA).
For dynamic contrast-enhanced (DCE) imaging, we used a 2D multislice T1-weighted saturation-recovery gradient-echo sequence (flip angle 30°; repetition time = 3.9 ms; echo time = 1.9 ms; centric phase ordering; acquired matrix 96 × 61; acquired voxel size 2.40 × 2.98 × 8 mm3 (interpolated to 0.90 × 0.89 × 8 mm3); field of view 230 × 182 mm2; five slices; slice thickness 8 mm). The total number of frames was 490, obtained with a time resolution of 1.85 s. Participants were injected with a gadolinium-based contrast agent (Gadovist) at the 10th and 54th timepoints, with a dosage corresponding to 0.045 mmol/kg bodyweight for each bolus. Three slices were angulated perpendicular to the hippocampus. The remaining two slices were angulated parallel to the ac-pc line below the corpus callosum. An axial T2-weighted MRI (5 slices; TE = 100 ms; TR = 3000 ms; field-of-view = 230 × 119 mm2; voxel size = 0.57 × 0.57 × 1.5 (interpolated to 0.45 × 0.45 × 1.5)) sequence of five slices with the same orientation, and slice thickness as the DCE sequence was used to manually draw regions of interest in the hippocampus, thalamus and white matter (WM) [25], one drawn in each hemisphere for each region of interest (ROI). Care was taken to avoid white matter hyperintensities and blood vessels when drawing the ROI. Examples of drawn placement of ROIs are shown in Fig. 1.
DCE analysis
The DCE MRI data were analysed with a semiautomated procedure using in-house developed MATLAB (MathWorks, Natick, MA, USA) software, as previously described [32]. The median value of permeability was extracted for each ROI to avoid the effects of possible outliers. Tissue concentration–time curves were evaluated using a Patlak model, as described in previous work [32]. Permeability values, measured as Ki, are reported as ml/100 g/min.
Health and lifestyle
The following health and lifestyle parameters were collected: educational attainment (number of school years), exercise frequency, BMI, alcohol consumption (units per week), smoking habits (smoker/not smoker and cigarettes per day), hypertension (yes/no), hypercholesterolemia (yes/no), diabetes (yes/no), history of heart disease (yes/no), history of stroke (yes/no), and Fazekas score for white matter lesions. The values of the health and lifestyle parameters are summarized in Table 1.
Statistical analysis
All statistical analyses were calculated using RStudio Version 1.3.1093 (R Core Team, 2013. R: a language and environment for statistical computing. R Foundation for Statistical Computing, Vienna, Austria. URL https://www.r-project.org/).
Missing data
Due to the nature of the study, which consists of cognitive testing (pen-and-paper and computerized), interviews, and MRI (DCE-MRI and structural MRI), there are different sample sizes of the databases (depending on which analysis is conducted), and some missing data between the databases. These missing data are assumed to be missing at random i.e. because of technical errors and failed data acquisition. To make it more understandable, we will provide a summary of each database. Out of the 108 participants who were MRI scanned, 31 BBB measurements were missing or removed. Fifteen were due to the participants not wanting to receive contrast agent, and 16 data points were removed because of extensive movement during the measurement. Furthermore, 2 participants who were MRI scanned did not attain volumetric data due to failed segmentation because of poor image quality. The longitudinal hippocampal decline was calculated based on percentage volume decline from T1 to T3. Thus, it only included participants present at both T1 and T3, constituting 102 participants.
Database 1 is used to assess the association between Ki and cognition and consists of a total number of 77 participants, with missing data points as follows: 1 for Ki, 4 for PRM, 2 for VPA and 3 for PAL. Database 2 is used to assess the association between hippocampal Ki and volume and consists of a total number of 77 participants, with missing data points as follows: 2 for hippocampus volume ratio (RGM) and 5 for hippocampus volume decline. Database 3 is used to assess the association between hippocampus volume and cognition. It consists of 114 participants, with missing data points as follows: 8 for PRM, 2 for VPA, 5 for PAL, 8 for hippocampal volume and 12 for hippocampal volume decline. Database 4 was used to examine the association between Ki and the health parameters and included a total number of 77 participants i.e. only the participants who had a BBB measurement. The missing data were as follows: 35 for number of cigarettes a day, 8 for units of alcohol consumed a week, 3 missing for physical activity, 5 missing Fazekas’s score and 2 missing general health information.
Linear mixed-effects models
To assess longitudinal changes in hippocampal volume, we applied a linear mixed-effects model (LMM), with time (T1, T2 and T3) as a fixed effect, participant as a repeated effect (to account for the random effect of each individual) and an unstructured covariance pattern to account for correlation between repeated measurements. We ran two versions of the longitudinal model. For the first model, we used hippocampal volume normalized to intracranial volume (ICV) as the dependent variable. For the second model, we normalized hippocampal volume to the remaining grey matter (RGM) volume by taking the ratio of the two measurements. This was done to inspect how hippocampal volume progressed relative to other grey matter regions. T1 was set as the reference in both models. Only the RGM-corrected hippocampal volume was used for subsequent analysis. The decennial atrophy rate was calculated as the percentage decline in left/right hippocampus volume (mm3) from T1 to T3. A paired Welch two-sample t test was used to compare left and right hippocampus volumes.
LMM was applied to assess longitudinal changes in cognitive functions for the VPA and PRM (PAL was only measured at T2 and T3). However, since no significant changes in cognitive functions over the 10-year span were observed in any of the tests, we only used the cognitive score attained at T3 for all subsequent analyses.
Linear regression and multiple linear regression
Linear regression was used to assess the association between BBB permeability and current cognitive scores. The cognitive score was set as the dependent variable, and Ki was set as the independent variable (hippocampus or WM). This yielded five different linear models per ROI.
Another linear regression model was used to assess the association between BBB permeability and hippocampal volume. Hippocampal volume (mean hippocampus volume ratio (RGM), mean hippocampal volume decline) was set as the dependent variable, and Ki was set as the independent variable (mean Ki), yielding two different regression models.
Linear regression was applied to assess the association between hippocampal volume and cognition. The cognitive score was set as the dependent variable, and left hippocampus volume and right hippocampus volume were set as regressors. One model was run per cognitive test per side, yielding a total of ten models.
Multiple linear regression was used to assess the effect of health parameters on BBB permeability in the hippocampus, thalamus and WM. The Ki of each ROI was set as the dependent variable, and all health variables were set as regressors, yielding a total of three multiple regression models. Statistically significant regressors were rerun in a submodel with the ROI as the dependent variable and the significant variable as the regressor.
Analysis of variance
A within-groups one-way ANOVA was performed to compare differences in Ki values between the areas of interest, namely, the hippocampus, thalamus and WM. A post hoc Bonferroni adjusted pairwise t test was used to compare mean Ki values between the regions of interest (ROIs).
Other statistical tests
P values were adjusted for multiple testing using a Bonferroni correction. Power was calculated using G*Power software, version 3.1.9.6 [33].
Results
Regional differences in BBB permeability
Within-groups one-way ANOVA revealed a statistically significant difference in Ki between the areas of interest (ROIs) (F(1.4, 108.9) = 10.81, p = 0.037) (Fig. 2). Pairwise t tests with a Bonferroni adjustment revealed a significantly higher Ki in the hippocampus (p = 0.001) and WM (p < 0.001) than in the thalamus but not between the hippocampus and WM. The Ki results are summarized in Table 2.
No association between cognition and BBB permeability
Linear regression was performed with cognition (VPA, PRM or PAL) as the dependent variable and BBB permeability as the independent variable (hippocampus or WM). There were no significant associations between any cognitive variables and either BBB measure (Fig. 3A, B).
A Association between cognitive tests and the mean hippocampus Ki value. B Association between cognitive tests and the mean WM Ki value. C Association between cognitive tests and the left and right hippocampus volume ratio (RGM). D Association between cognitive tests and percentage hippocampal volume decline from T1-T3. E Hippocampal volume trajectory, ICV (top) and RGM (bottom) corrected. F Association between the mean hippocampus volume ratio (RGM) (top) and the mean hippocampus volume decline (T1–T3) (bottom) and mean hippocampal Ki value
BBB permeability and hippocampal volume
First, we tested whether the mean hippocampus Ki correlated with the mean hippocampus volume ratio (RGM). Second, we tested whether the mean hippocampal Ki correlated with the mean hippocampal atrophy rate from T1 to T3. However, no significant association was found between hippocampal volume or the degree of atrophy and the hippocampal Ki (Fig. 3F).
Longitudinal changes in hippocampal volume
The annual atrophy rate was ≈1.2%, calculated as a percentage decrease from T1 to T3. The trajectory of hippocampal volume differed depending on the applied normalization method. When the hippocampal volume was normalized to the intracranial volume (ICV), we observed a linear decline, which became slightly more steep (i.e. more progressive) from T2 to T3. When normalizing the hippocampus to the remaining grey matter (RGM), the hippocampal volume peaked at T2, indicating that the hippocampus atrophied at a slower rate than the remaining grey matter. After its peak at T2, the hippocampus experienced an accelerated atrophy rate surpassing that of the grey matter. The right hippocampus was significantly larger than the left hippocampus at all timepoints (p < 0.001; 95% CI [− 2.5e−04, − 8.8e−05]) (Fig. 3E).
Cognition and hippocampal volume
Next, we investigated whether there was an association between the hippocampus volume ratio (RGM) at T3 and verbal or visual memory function. A significant association was found between the PRM score and the right hippocampal volume ratio (RGM), where a larger right hippocampus volume ratio was correlated with better PRM score (p = 0.011) (Fig. 3C). This remained significant after adjusting for multiple testing. We observed a significant association between the VPA retention score and the left (p < 0.01) and right hippocampus volume ratios (p < 0.01), where a larger hippocampal volume ratio (RGM) was associated with better retention scores (i.e., fewer errors) (Fig. 3C). There was no significant association between hippocampal volume decline and cognition (Fig. 3D). Post hoc power analysis revealed a power of 0.99 for all mentioned significant associations. There was also a significant association between the left hippocampus volume ratio and the VPA word pair score (p = 0.048), but this did not remain significant after correction for multiple testing.
Health and lifestyle
To assess whether health and lifestyle factors are related to BBB permeability in the hippocampus, thalamus or WM, we ran three separate multiple regression models with the hippocampus, thalamus or WM Ki as the dependent variable and all health parameters as regressors. An increased Ki in the WM was significantly associated with a diabetes diagnosis (p = 0.0149) and a higher BMI (p = 0.0079). Likewise, an increased Ki value in the thalamus was significantly associated with a diabetes diagnosis (p = 0.0161) and a higher Fazekas score in the periventricular white matter (PVWM) (p = 0.0237) (Fig. 4). Since multiple regression only includes pairwise complete observations, the full model only had 36 observations. Therefore, we ran a submodel with only the significant regressors as independent variables, which would include a larger sample size (n = 74). Diabetes was significant when run in the submodel as a single regressor for WM (p = 0.0398), and the thalamus was borderline significant (p = 0.0883). PVWM and BMI were no longer significant as a single regressor for any ROI, but the BMI showed a tendency towards significance (p = 0.0807) (Fig. 4).
Post hoc analysis of association between overall vascular risk factor and BBB permeability
To further examine the effect of vascular risk factors, we combined the risk factors into an overall vascular risk factor score. In this combined score, we included diabetes (yes/no), BMI and Fazekas score. Participants were assigned 0–3 points, depending on whether they had the vascular risk factors and to what extent. Points were assigned in the following manner: diabetes, if yes = 1 point, if no = 0 point. BMI, if equal to or more than 27 = 1, if less than 27 = 0 point. Fazekas scores were first averaged across DWM and PVWM, then divided by 3 to achieve a score ranging from 0 to 1. There was a significant association between vascular risk factors (N = 72) and Ki in the hippocampus (p = 0.015), thalamus (p = 0.007) and white matter (p = 0.001), where higher vascular risk factor was associated with increased Ki (Supplementary Fig. 1).
Discussion
We examined whether BBB permeability accompanied cognitive dysfunction or hippocampal atrophy rate in a cohort of participants without dementia disease. Our focus was on the hippocampus, considering the importance of this structure in both ageing and Alzheimer’s disease. We report age-related hippocampal atrophy and an association between the hippocampal volume ratio and the verbal and visual memory score at T3. There were no significant associations in any of our ROIs between BBB permeability and cognition, hippocampal volume or atrophy rate. However, participants diagnosed with type II diabetes had significantly higher BBB permeability in the thalamus and WM.
Ageing, cognition, and BBB permeability
There are discrepancies in the literature regarding the relationship between BBB permeability and cognitive function. Several studies have found an association between increased BBB leakage in the hippocampus and cognitive dysfunction, including incipient and current AD [2, 8, 9]. This is intriguing, considering the crucial role of the hippocampus in both ageing and neurodegeneration. However, other studies have been unable to confirm the same relationship between the hippocampal BBB and cognition. Instead, these studies point to an association between increased BBB leakage in the white and grey matter and cognitive functions [13, 34].
We report a lack of association between BBB leakage and cognition, in accordance with the view that BBB leakage in healthy ageing is small — if it indeed exists at all [35]. This may partly be because our cohort had not yet developed cognitive decline. According to previous research and per the well-known proposed model by Jack et al. [36], cognitive impairments are one of the last AD biomarkers to be affected. Furthermore, since we did not observe a significant decline in memory or MMSE scores, we only assessed current cognitive performance. When Verheggen et al. [10] used only the most recent measure of cognition — as opposed to a longitudinal approach — they also could not find a significant relationship between BBB permeability in white matter and cognition. Therefore, it is possible that the relationship between the white matter BBB and cognition is most apparent over time and might only be evident at an older age than that of the current cohort [10].
A previous study by Montagne et al. [2] observed a breakdown of the hippocampus BBB in normal ageing and accelerated breakdown in the hippocampus BBB in individuals with MCI. Furthermore, the authors suggest that the hippocampal BBB may be the first to deteriorate in normal ageing and that it might precede the hippocampal atrophy observed in AD. In two other studies, the same group observed breakdown of the hippocampus BBB in individuals with early cognitive dysfunction, even in the absence of amyloid-beta plaques and tau tangles [9], and increased hippocampal BBB permeability in individuals with the APOE4 genotype [8]. With this in mind, BBB disruptions may evolve differently in healthy and pathological ageing, with white matter regions being primarily affected in healthy ageing and the hippocampal BBB being more disrupted in prodromal AD [10].
Regional BBB differences
We observed regional differences in BBB permeability between the hippocampus, thalamus and WM. The thalamus was the least permeable (i.e. lowest Ki), whereas the hippocampus and WM had more leaky BBBs (i.e. higher Ki values). As previously mentioned, the BBB consists of and is dependent on cerebral endothelial cells, pericytes and astrocytes, all of which are distributed heterogeneously in the brain. Consequently, the BBB is a heterogeneous unit, and different brain regions are characterized by variations in BBB tightness depending on the distribution of endothelial cells, astrocytes and pericytes in that area [37]. Furthermore, the properties of the BBB may change as a result of ageing, alterations in hormonal status or disease conditions [37]. Consequently, it is possible that the regions most exposed to the effects of ageing, namely, the hippocampus and white matter, are also the regions with the most age-related BBB disruption. In line with this, a previous study from our group reported that young, healthy participants had higher BBB permeability in grey matter regions, such as the thalamus and lower Ki values in the white matter [38]. This suggests that white matter regions exhibit a more robust BBB integrity at a younger age; however, we find the opposite in the current study, which indicated that the WM BBB loses integrity with increasing age.
Hippocampal atrophy
We report that the hippocampus undergoes progressive atrophy in the course of normal healthy ageing. Specifically, we found that the hippocampus undergoes atrophy at a slower pace than the remaining grey matter until the age of 63. Thereafter, the hippocampus undergoes atrophy at a faster rate than the remaining grey matter. Our findings are consistent with one study of 19,000 individuals from the UK Biobank [39] and a second cross-sectional study of 1100 participants [40]. Consistent with previous observations, the right hippocampus was significantly larger than the left hippocampus at all timepoints [39, 41,42,43].
We observed an average annual atrophy rate of 1.2% from 58 to 68 years of age. This is consistent with previous findings of hippocampal atrophy in healthy ageing (see: [44] for meta-analysis). However, AD patients are observed to have a smaller hippocampal volume than healthy individuals, possibly due to a faster atrophy rate [45]. Our data also show a relatively large spread of decennial atrophy rates, ranging from 0.9 to 20%. Therefore, although it is clear that some volume reduction is expected during healthy ageing, atrophy exceeding a certain threshold might be a sign of incipient neurodegenerative disease. In support of this result, previous studies have found a smaller hippocampal volume to be associated with cognitive deficits and conversion to AD [23, 24, 46]. Furthermore, an increasing body of evidence suggests that the volume and shape of hippocampal subfields might be a more accurate biomarker for separating healthy and pathological ageing than the overall hippocampal volume [45, 47].
We observed a significant association between the hippocampus volume ratio and verbal and visual memory performance. The crucial role of the hippocampus in memory formation and retrieval is well established in cognitive neuroscience [48,49,50]. In particular, the hippocampus is known for its crucial role in upholding spatial memory tasks in both rodents and humans [51,52,53]. Therefore, it is unsurprising that memory deficits are common with brain ageing and that this is one of the early symptoms of AD [51, 54].
We were particularly interested in whether BBB permeability correlated with hippocampal atrophy, in support of studies indicating that increased permeability of the hippocampal BBB may cause hippocampal atrophy and neurodegeneration [2]. While we observed a loss of hippocampal volume and found a significant association between hippocampal volume and visual memory, we did not find evidence that the current BBB properties correlate with prior atrophy rates.
Health and lifestyle
Participants diagnosed with type II diabetes were observed to have higher BBB permeability in the WM and thalamus than participants without diabetes. We believe that the adverse effects of diabetes on the cerebrovascular system likely contribute to compromised BBB integrity. This is further supported by the finding that increased BBB permeability was also associated with a higher Fazekas score in the PVWM as well as the BMI.
Previous studies have found a relationship between diabetes and BBB permeability in rodents. In their review, Brook et al. [55] proposed several mechanisms that may trigger BBB dysfunction in type II diabetes, such as oxidative stress and chronic inflammation. Oxidative stress may lead to degradation of BBB tight junctions or endothelial cell apoptosis by activating caspase-3 [56]. Meanwhile, chronic inflammation affects brain endothelial cells, leading to dysregulation of BBB tight junctions [55]. Last, hyperglycaemia may disrupt BBB integrity via different mechanisms, such as upregulation of endothelial cell inflammation, production of superoxide [57] or neovascularization [58]. In accordance with this, another study [59] observed increased BBB permeability in patients with type II diabetes and in participants with more white matter hyperintensities. However, only seven patients in the current cohort were diagnosed with type II diabetes. Therefore, this aspect should be investigated further with a larger sample size.
Limitations
The current study has some limitations, which are discussed below. First, we only obtained the BBB measurement at one timepoint. Therefore, we could not assess whether BBB permeability changed with age. We also did not have data from younger participants who had undergone identical scanning sequences. Therefore, we could not assess cross-sectional differences in BBB permeability between young and old age groups for all our ROIs. Second, the generalizability of our study is limited because all our participants were male. This is due to the original purpose of the Metropolit cohort, which was established in 1965 to study social mobility. At the time, social mobility was regarded to be mainly connected with the male breadwinner’s occupation, and hence, only men were included in the study [25]. Despite the limitations of examining a male-only cohort, we believe that the extensive information accumulated from the cohort over the years is valuable for research on ageing and should be utilized. Studies investigating sex differences in BBB permeability in humans are scarce, but some studies have indicated that there may be sex-related differences in BBB permeability [60]. This should be kept in mind when studying BBB permeability and interpreting findings.
It is possible that we did not observe a decline in memory functions due to cohort effects (i.e. attrition, selection bias), retest effects or tester effects. We attempted to control for these effects in the following way: Linear mixed effect models are considered robust when investigating longitudinal changes and can compensate for differing sample sizes to a certain extent [61]. All staff administering the cognitive tests underwent the same training procedure and were certified by the same neuropsychologist. Finally, we believe that since there is a 5-year span between each timepoint, it is unlikely that retest effects largely confounded the participant’s test results. Nevertheless, we mention these factors because they are common challenges in longitudinal studies and are likely present to some extent, even when efforts are made to control them.
Since measures of BBB permeability were only available to us at the final time, we could neither study age-related changes between BBB permeability nor investigate causal relationships between cognition and volume/atrophy. However, we assume BBB degradation to be a long-term developing process, entailing that the participants with a currently high Ki also had a previously high Ki. With this assumption in mind, we hypothesized that Ki would correlate with cognition and volume/atrophy. Thus, although we cannot determine the causal relationship between these variables, this analysis contributes to illuminating the relationship between BBB permeability, cognition and volume/atrophy in a healthy cohort.
It should be mentioned that methods for obtaining BBB permeability measurements vary significantly. Our BBB measurement setup has been used previously in studies of patients with multiple sclerosis and is founded on basic simulation and validation studies [38, 62,63,64]. The time resolution is an essential difference between our method and the method of Montagne et al. (2015), who applied a time resolution of 15.4 s against our resolution of 1.85 s, which allowed us to better sample the internal carotid artery (AIF), giving us a more accurate input function. Conversely, the study of Montagne et al. has a superior spatial resolution (0.6 × 0.6 × 5 mm), which allows them to inspect BBB changes more accurately in subregions of the hippocampus, such as the CA1, CA3 and dentate gyrus. Both methods have advantages and disadvantages, and the methodological differences should be considered if comparing studies. Furthermore, our images are taken axially and with an 8-mm thickness. This might produce partial volume effects, as the slices could include non-hippocampal tissue. However, we note that we took great care when placing and drawing our ROIs as accurately as possible to limit possible partial volume effects. We also note that our observed Ki values are robust across ROI. If there were considerable partial volume effects in the hippocampus, we would expect Ki values for this region to deviate and be significantly higher than those observed in the thalamus and WM. This is not the case; thus, we feel confident that partial volume effects do not constitute a significant problem.
Finally, we wish to comment on the occurrence of negative Ki values. A negative Ki value may seem counterintuitive, since it is not biologically possible to attain a Ki of less than 0. We know that in a healthy brain, the gadolinium compound is too big and will not pass through the BBB, meaning that Ki would, in most cases, be approximately 0. However, all biological measurements consist of a certain amount of measurement error. This error, in addition to the Patlak-method being a linear regression, will, in some instances, cause the Ki, which in this context is analogous to a regression coefficient, to be calculated as below 0. It is possible to take measures to avoid this, such as setting a limit in the Patlak-regression and not permitting Ki values below 0. However, this does not circumvent the issue that the BBB permeability for common MRI contrast agents is very low and close to zero. Moreover, we do not view it as a viable option to remove the negative values. Although they do not make sense biologically, they make sense statistically. Since our average Ki value in healthy participants should be 0, we would also expect a certain number of points above and below 0, following a normal distribution. Removing these data would distort the distribution and our results. Furthermore, the data has been quality-checked, and bad data has already been excluded. Therefore, we regard the remaining data as valid, although some Ki values are below 0 due to chance and measurement uncertainty.
Conclusion
Our data suggest that BBB permeability is not correlated with cognitive function or hippocampal volume in healthy brain ageing. We report an age-related hippocampal atrophy rate in line with previous research, but this is not correlated with current hippocampal BBB permeability. However, BBB permeability differences were associated with diabetes and white matter hyperintensities in concert with the notion that type II diabetes is associated with vascular pathology. Our study and other studies indicate that the BBB is affected differently based on sex, age and clinical diagnosis. Therefore, the BBB may behave differently in individuals at risk for developing AD. We suggest that future studies assess BBB integrity longitudinally from a life course perspective and investigate how the trajectory of permeability measures differ across age and gender and in brain pathologies, such as Alzheimer’s disease.
References
Abbott NJ, Patabendige AA, Dolman DE, Yusof SR, Begley DJ. Structure and function of the blood-brain barrier. Neurobiol Dis. 2010;37(1):13–25.
Montagne A, Barnes SR, Sweeney MD, Halliday MR, Sagare AP, Zhao Z, Toga AW, Jacobs RE, Liu CY, Amezcua L, Harrington MG, Chui HC, Law M, Zlokovic BV. Blood-brain barrier breakdown in the aging human hippocampus. Neuron. 2015;85(2):296–302.
Saunders NR, Liddelow SA, Dziegielewska KM. Barrier mechanisms in the developing brain. Front Pharmacol. 2012;3(March):1–18.
Sweeney MD, Sagare AP, Zlokovic BV. Blood-brain barrier breakdown in Alzheimer disease and other neurodegenerative disorders. Nat Rev Neurol. 2018;14(3):133–50.
Zlokovic BV. The blood-brain barrier in health and chronic neurodegenerative disorders. Neuron. 2008;57(2):178–201.
Bowman GL, Dayon L, Kirkland R, Wojcik J, Peyratout G, Severin IC, Henry H, Oikonomidi A, Migliavacca E, Bacher M, Popp J. Blood-brain barrier breakdown, neuroinflammation, and cognitive decline in older adults. Alzheim Dement. 2018;14(12):1640–50.
Iadecola C. Dangerous leaks: blood-brain barrier woes in the aging hippocampus. Neuron. 2015;85(2):231–3.
Montagne A, Nation DA, Sagare AP, Barisano G, Sweeney MD, Chakhoyan A, Pachicano M, Joe E, Nelson AR, D’Orazio LM, Buennagel DP, Harrington MG, Benzinger TLS, Fagan AM, Ringman JM, Schneider LS, Morris JC, Reiman EM, Caselli RJ, Chui HC, TCW J, Chen Y, Pa J, Conti PS, Law M, Toga AW, Zlokovic BV. APOE4 leads to blood–brain barrier dysfunction predicting cognitive decline. Nature. 2020;(November 2019)1–6.
Nation DA, Sweeney MD, Montagne A, Sagare AP, D’Orazio LM, Pachicano M, Sepehrband F, Nelson AR, Buennagel DP, Harrington MG, Benzinger TL, Fagan AM, Ringman JM, Schneider LS, Morris JC, Chui HC, Law M, Toga AW, Zlokovic BV. Blood–brain barrier breakdown is an early biomarker of human cognitive dysfunction. Nat Med. 2019;25(2):270–6.
Verheggen IC, de Jong JJ, van Boxtel MP, Postma AA, Jansen JF, Verhey FR, Backes WH. Imaging the role of blood–brain barrier disruption in normal cognitive ageing. GeroScience. 2020;42(6):1751–64.
Starr JM, Farrall AJ, Armitage P, McGurn B, Wardlaw J. Blood-brain barrier permeability in Alzheimer’s disease: a case-control MRI study. Psychiatry Res-Neuroimaging. 2009;171(3):232–41.
van de Haar HJ, Burgmans S, Jansen JFA, van Osch MJP, van Buchem MA, Muller M, Hofman PAM, Verhey FRJ, Backes WH. Blood-brain barrier leakage in patients with early Alzheimer disease. Radiology. 2016;281(2):527–615.
Verheggen ICM, de Jong JJA, van Boxtel MPJ, Gronenschild EHBM, Palm WM, Postma AA, Jansen JFA, Verhey FRJ, Backes WH. Increase in blood–brain barrier leakage in healthy, older adults. GeroScience. 2020;42(6):1183–93.
Rosenberg GA. Blood-brain barrier permeability in aging and Alzheimer’s disease. J Prev Alzheim Dis. 2014;1(3):138–9.
Enzinger C, Fazekas F, Matthews PM, Ropele S, Schmidt H, Smith S, Schmidt R. Risk factors for progression of brain atrophy in aging: six-year follow-up of normal subjects. Neurology. 2005;64(10):1704–11.
Fjell AM, McEvoy L, Holland D, Dale AM, Walhovd KB. What is normal in normal aging? Effects of aging, amyloid and Alzheimer’s disease on the cerebral cortex and the hippocampus. Prog Neurobiol. 2014;117:20–40.
Hedman AM, van Haren NE, Schnack HG, Kahn RS, Hulshoff Pol HE. Human brain changes across the life span: a review of 56 longitudinal magnetic resonance imaging studies. Human Brain Mapp. 2012;33(8):1987–2002.
Aljondi R, Szoeke C, Steward C, Yates P, Desmond P. A decade of changes in brain volume and cognition. Brain Imaging Behav. 2019;13(2):554–63.
Koenig KA, Sakaie KE, Lowe MJ, Lin J, Stone L, Bermel RA, Beall EB, Rao SM, Trapp BD, Phillips MD. Hippocampal volume is related to cognitive decline and fornicial diffusion measures in multiple sclerosis. Magn Reson Imaging. 2014;32(4):354–8.
Thomann PA, Wustenberg T, Nolte HM, Menzel PB, Wolf RC, Essig M, Schroder J. Hip- pocampal and entorhinal cortex volume decline in cognitively intact elderly. Psychiatry Res-Neuroimaging. 2013;211(1):31–6.
Bettio LE, Rajendran L, Gil-Mohapel J. The effects of aging in the hippocampus and cognitive decline. Neurosci Biobehav Rev. 2017;79(April):66–86.
Evans TE, Adams HH, Licher S, Wolters FJ, van der Lugt A, Ikram MK, O’Sullivan MJ, Vernooij MW, Ikram MA. Subregional volumes of the hippocampus in relation to cognitive function and risk of dementia. Neuroimage. 2018;178(May):129–35.
Karas G, Sluimer J, Goekoop R, Van Der Flier W, Rombouts SA, Vrenken H, Scheltens P, Fox N, Barkhof F. Amnestic mild cognitive impairment: structural MR imaging findings predictive of conversion to Alzheimer disease. Am J Neuroradiol. 2008;29(5):944–9.
Kerchner GA, Deutsch GK, Zeineh M, Dougherty RF, Saranathan M, Rutt BK. Hippocampal CA1 apical neuropil atrophy and memory performance in Alzheimer’s disease. Neuroimage. 2012;63(1):194–202.
Osler M, Lund R, Kriegbaum M, Christensen U, Andersen AMN. Cohort profile: the Metropolit 1953 Danish male birth cohort. Int J Epidemiol. 2006;35(3):541–5.
Zarnani K, Nichols TE, Alfaro-Almagro F, Fagerlund B, Lauritzen M, Rostrup E, Smith SM. Discovering markers of healthy aging: a prospective study in a Danish male birth cohort. Aging. 2019;11(16):5943–74.
Horwitz A, Thomsen MD, Wiegand I, Horwitz H, Klemp M, Nikolic M, Rask L, Lauritzen M, Benedek K. Visual steady state in relation to age and cognitive function. PLoS One. 2017;12(2):1–23.
Horwitz A, Mortensen EL, Osler M, Fagerlund B, Lauritzen M, Benedek K. Passive doublesensory evoked coherence correlates with long-term memory capacity. Front Hum Neurosci. 2017;11(December):1–21.
Horwitz A, Klemp M, Horwitz H, Thomsen MD, Rostrup E, Mortensen EL, Osler M, Lauritzen M, Benedek K. Brain responses to passive sensory stimulation correlate with intelligence. Front Aging Neurosci. 2019;10(JUL):1–17.
Richard N, Nikolic M, Mortensen EL, Osler M, Lauritzen M, Benedek K. Steady-state visual evoked potential temporal dynamics reveal correlates of cognitive decline. Clin Neurophysiol. 2020;131(4):836–46.
Vestergaard MB, Lindberg U, Knudsen MH, Urdanibia-Centelles O, Bakhtiari A, Mortensen EL, Osler M, Fagerlund B, Benedek K, Lauritzen M, Larsson HBW. Subclinical cognitive deficits are associated with reduced cerebrovascular response to visual stimulation in mid-sixties men. GeroScience. 2022;(0123456789).
Larsson HB, Courivaud F, Rostrup E, Hansen AE. Measurement of brain perfusion, blood volume, and blood-brain barrier permeability, using dynamic contrast-enhanced T1-weighted MRI at 3 tesla. Magn Reson Med. 2009;62(5):1270–81.
Faul F, Erdfelder E. G*Power 3: a flexible statistical power analysis program for the social, behavioral, and biomedical sciences. Behav Res Methods. 2007;39:175–91.
Li Y, Li M, Zhang X, Shi Q, Yang S, Fan H, Qin W, Yang L, Yuan J, Jiang T, Hu W. Higher blood–brain barrier permeability is associated with higher white matter hyperintensities burden. J Neurol. 2017;264(7):1474–81.
Banks WA, Reed MJ, Logsdon AF, Rhea EM, Erickson MA. Healthy aging and the blood–brain barrier. Nature Aging. 2021;1(March).
Jack CR, Knopman DS, Jagust WJ, Petersen RC, Weiner MW, Aisen PS, Shaw LM, Vemuri P, Wiste HJ, Weigand SD, Lesnick TG, Pankratz VS, Donohue MC, Trojanowski JQ. Tracking pathophysiological processes in Alzheimer’s disease: an updated hypothetical model of dynamic biomarkers. Lancet Neurol. 2013;12(2):207–16.
Wilhelm I, Ny´ ul-T´ oth A, Suciu M, Hermenean A, Krizbai IA. Heterogeneity of the blood-brain barrier. Tissue Barriers. 2016;4 (1).
Cramer SP, Larsson HBW, Knudsen MH, Simonsen HJ, Vestergaard MB, Lindberg U. Reproducibility and optimal arterial input function selection in dynamic contrast-enhanced perfusion MRI in the healthy brain. J Magn Reson Imaging. 2022;1–12.
Nobis L, Manohar SG, Smith SM, Alfaro-Almagro F, Jenkinson M, Mackay CE, Husain M. Hippocampal volume across age: nomograms derived from over 19,700 people in UK Biobank. NeuroImage: Clin. 2019;23(March):101904.
Fjell AM, Westlye LT, Grydeland H, Amlien I, Espeseth T, Reinvang I, Raz N, Holland D, Dale AM, Walhovd KB. Critical ages in the life course of the adult brain: nonlinear subcortical aging. Neurobiol Aging. 2013;34(10):2239–47.
Kuo F, Massoud TF. Structural asymmetries in normal brain anatomy: a brief overview. Ann Anat. 2022;241:151894.
Pedraza O, Bowers D, Gilmore R. Asymmetry of the hippocampus and amygdala in MRI volumetric measurements of normal adults. J Int Neuropsychol Soc. 2004;10(5):664–78.
Shi F, Liu B, Zhou Y, Yu C, Jiang T. Hippocampal volume and asymmetry in mild cognitive impairment and Alzheimer’s disease: meta-analyses of MRI studies. Hippocampus. 2009;19(11):1055–64.
Fraser MA, Shaw ME, Cherbuin N. A systematic review and meta-analysis of longitudinal hippocampal atrophy in healthy human ageing. Neuroimage. 2015;112:364–74.
Franko E, Joly O. Evaluating Alzheimer’s disease progression using rate of regional hippocampal atrophy. PLoS One. 2013;8(8).
Devanand DP, Pradhaban G, Liu X, Khandji A, De Santi S, Segal S, Rusinek H, Pelton GH, Honig LS, Mayeux R, Stern Y, Tabert MH, De Leon MJ. Hippocampal and entorhinal atrophy in mild cognitive impairment: prediction of Alzheimer disease. Neurology. 2007;68(11):828–36.
de Flores R, La Joie R, Chetelat G. Structural imaging of hippocampal subfields in healthy aging and Alzheimer’s disease. Neuroscience. 2015;309:29–50.
Tulving E. Tulving (1972).pdf, 1972.
Lisman J, Buzsáki G, Eichenbaum H, Nadel L, Ranganath C, Redish AD. How the HPC contributes to memory, navigation and cognition. 2017;20(11).
Knierim JJ. The hippocampus. Curr Biol. 2015;25(23):R1116–21.
Konishi K, Mckenzie S, Etchamendy N, Roy S, Bohbot VD. Hippocampus-dependent spatial learning is associated with higher global cognition among healthy older adults. Neuropsychologia. 2017;106(July 2016):310–21.
Moser MB, Moser EI. Distributed encoding and retrieval of spatial memory in the hippocampus. J Neurosci. 1998;18(18):7535–42.
Fritch HA, MacEvoy SP, Thakral PP, Jeye BM, Ross RS, Slotnick SD. The anterior hippocampus is associated with spatial memory encoding. Brain Res. 2020;1732(June 2019):146696.
Vlcek K, Laczˇo J. Neural correlates of spatial navigation changes in mild cognitive impairment and Alzheimer’s disease. Front Behav Neurosci. 2014;8(MAR):1–6.
Brook E, Mamo J, Wong R, Al-Salami H, Falasca M, Lam V, Takechi R. Blood-brain barrier disturbances in diabetes-associated dementia: therapeutic potential for cannabinoids. Pharmacol Res. 2019;141(September 2018):291–7.
Zehendner CM, Librizzi L, de Curtis M, Kuhlmann CR, Luhmann HJ. Caspase-3 contributes to ZO-1 and Cl-5 Tight-junction disruption in rapid anoxic neurovascular unit damage. PLoS One. 2011;6(2):1–11.
Won SJ, Tang XN, Suh SW, Yenari MA, Swanson RA. Hyperglycemia promotes tissue plasminogen activator-induced hemorrhage by increasing superoxide production. Ann Neurol. 2011;70(4):583–90.
Prakash R, Johnson M, Fagan SC, Ergul A. Cerebral neovascularization and remodeling patterns in two different models of type 2 diabetes. PLoS One. 2013;8(2).
Starr JM, Wardlaw JM, Ferguson K, MacLullich A, Deary IJ, Marshall I. Increased blood-brain barrier permeability in type II diabetes demonstrated by gadolinium magnetic resonance imaging. J Neurol Neurosurg Psychiatry. 2003;74(1):70–6.
Moon Y, Lim C, Kim Y, Moon WJ. Sex-related differences in regional blood–brain barrier integrity in non-demented elderly subjects. Int J Mol Sci. 2021;22(6):1–15.
Ozenne B, Forman J. Introduction to linear mixed models for repeated measurements data: analysis of single group studies with R-package LMMstar. Section of Biostatistics, University of Copenhagen: Lecture notes; 2021. p. 1–43.
Cramer SP, Larsson HB. Accurate determination of blood-brain barrier permeability using dynamic contrast-enhanced T1-weighted MRI: a simulation and in vivo study on healthy subjects and multiple sclerosis patients. J Cereb Blood Flow Metab. 2014;34(10):1655–65.
Cramer SP, Simonsen H, Frederiksen JL, Rostrup E, Larsson HB. Abnormal blood-brain barrier permeability in normal appearing white matter in multiple sclerosis investigated by MRI. NeuroImage: Clin. 2014;4:182–9.
Knudsen MH, Lindberg U, Frederiksen JL, Vestergaard MB, Simonsen HJ, Varatharaj A, Galea I, Blinkenberg M, Sellebjerg F, Larsson HBW, Cramer SP. Blood-brain barrier permeability changes in the first year after alemtuzumab treatment predict 2-year outcomes in relapsing-remitting multiple sclerosis. Mult Sclerosis Relat Disord. 2022;63(May).
Acknowledgements
We want to thank the radiographers Karina Elin Segers, Helle Juhl Simonsen and Robabeh Hozouri Tavangar for all their help with the acquisition of the MRI scans. We thank Sine Kongsbak Arvedsen and Natalia Christina Brandstrup for coordinating the project and data collection. We also thank Jayachandra Mitta Raghava for his work on the segmentation of the MRI scans.
Funding
The study was supported by the Lundbeck Foundation Grant (Drug delivery to the brain and blood–brain barrier transport mechanisms, #R302-2018–2266) and a Nordea Foundation Grant to the Center for Healthy Ageing at the University of Copenhagen. The Copenhagen Ageing and Midlife Biobank is supported by grants from the Velux Foundation (VELUX26145 and 31539).
Author information
Authors and Affiliations
Corresponding author
Ethics declarations
Ethical approval
The study was approved by the Capital Region of Denmark’s Committee on Health Research Ethics (H-1–2014032). All the participants provided written consent regarding their participation and publication of the current data.
Conflict of interest
The authors declare no competing interests.
Additional information
Publisher's note
Springer Nature remains neutral with regard to jurisdictional claims in published maps and institutional affiliations.
The authors, Henrik BW Larsson and Ulrich Lindberg, contributed equally and share senior authorship.
Supplementary Information
Below is the link to the electronic supplementary material.
Rights and permissions
This article is published under an open access license. Please check the 'Copyright Information' section either on this page or in the PDF for details of this license and what re-use is permitted. If your intended use exceeds what is permitted by the license or if you are unable to locate the licence and re-use information, please contact the Rights and Permissions team.
About this article
Cite this article
Bakhtiari, A., Vestergaard, M.B., Benedek, K. et al. Changes in hippocampal volume during a preceding 10-year period do not correlate with cognitive performance and hippocampal blood‒brain barrier permeability in cognitively normal late-middle-aged men. GeroScience 45, 1161–1175 (2023). https://doi.org/10.1007/s11357-022-00712-2
Received:
Accepted:
Published:
Issue Date:
DOI: https://doi.org/10.1007/s11357-022-00712-2